How to improve the data denoising effect in C++ big data development?
How to improve the data denoising effect in C big data development?
Abstract:
In C big data development, data denoising is a very important task. The purpose of data denoising is to eliminate random fluctuations caused by noise and improve the quality and reliability of data. For large-scale data sets, efficiency and accuracy are often two aspects we need to balance. This article will introduce several methods to improve the data denoising effect in C big data development, and attach corresponding code examples.
- Data preprocessing
Before performing data denoising, you first need to perform some preprocessing work on the original data to improve the denoising effect. Common preprocessing methods include data cleaning, data segmentation and feature extraction.
Data cleaning: Reduce the impact of noise by deleting or correcting outliers and missing values in the data.
Data splitting: Split large-scale data sets into multiple smaller data blocks to facilitate distributed processing and parallel computing.
Feature extraction: Extract useful features from the original data to facilitate subsequent data analysis and mining. Commonly used feature extraction methods include principal component analysis (PCA), singular value decomposition (SVD), etc.
- Commonly used denoising algorithms
In C big data development, commonly used denoising algorithms include moving average method, median filtering method, wavelet transform, etc.
Moving average method: The moving average method is a simple and effective denoising method. It removes noise fluctuations by averaging the data over a period of time. The following is a sample code:
void moving_average_filter(float* data, int size, int window_size) { for (int i = window_size; i < size - window_size; i++) { float sum = 0.0; for (int j = i - window_size; j <= i + window_size; j++) { sum += data[j]; } data[i] = sum / (2 * window_size + 1); } }
Median filtering method: Median filtering method removes noise by calculating the median value of data within a period of time. It can better retain the edge information of the signal and is suitable for removing impulse noise. The following is a sample code:
void median_filter(float* data, int size, int window_size) { for (int i = window_size; i < size - window_size; i++) { float temp[2*window_size+1]; for (int j = i - window_size; j <= i + window_size; j++) { temp[j - (i - window_size)] = data[j]; } std::sort(temp, temp + 2*window_size+1); data[i] = temp[window_size]; } }
Wavelet transform: Wavelet transform is a denoising method based on time-frequency analysis. It is able to decompose the original signal into sub-signals of different frequencies and eliminate noise through threshold processing. The following is a sample code:
void wavelet_transform(float* data, int size) { // 进行小波变换 // ... // 设置阈值 float threshold = 0.0; // 阈值处理 for (int i = 0; i < size; i++) { if (data[i] < threshold) { data[i] = 0.0; } } }
- Parallel Computing Optimization
When processing large-scale data sets, single-machine computing may not be able to meet the requirements. In C big data development, parallel computing can be used to accelerate the data denoising process and improve efficiency.
For example, OpenMP can be used to implement multi-threaded parallel computing. The following is a sample code:
#include <omp.h> void parallel_moving_average_filter(float* data, int size, int window_size) { #pragma omp parallel for for (int i = window_size; i < size - window_size; i++) { ... } }
By rationally using parallel computing, the computing power of multi-core processors can be fully utilized and the efficiency of data denoising can be improved.
Conclusion:
This article introduces methods to improve data denoising effect in C big data development, and gives corresponding code examples. Through data preprocessing, selecting appropriate denoising algorithms, and parallel computing optimization, we can achieve efficient and accurate data denoising on large-scale data sets. I hope readers can learn from this article how to improve the data denoising effect in C big data development, and be applied and improved in practical applications.
The above is the detailed content of How to improve the data denoising effect in C++ big data development?. For more information, please follow other related articles on the PHP Chinese website!
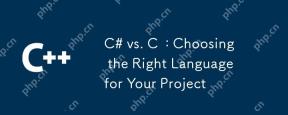
C# is suitable for projects that require development efficiency and type safety, while C is suitable for projects that require high performance and hardware control. 1) C# provides garbage collection and LINQ, suitable for enterprise applications and Windows development. 2)C is known for its high performance and underlying control, and is widely used in gaming and system programming.
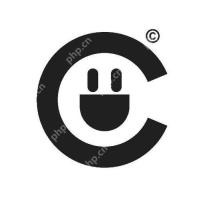
C code optimization can be achieved through the following strategies: 1. Manually manage memory for optimization use; 2. Write code that complies with compiler optimization rules; 3. Select appropriate algorithms and data structures; 4. Use inline functions to reduce call overhead; 5. Apply template metaprogramming to optimize at compile time; 6. Avoid unnecessary copying, use moving semantics and reference parameters; 7. Use const correctly to help compiler optimization; 8. Select appropriate data structures, such as std::vector.
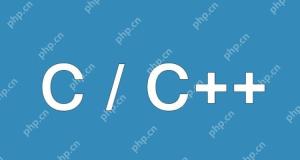
The volatile keyword in C is used to inform the compiler that the value of the variable may be changed outside of code control and therefore cannot be optimized. 1) It is often used to read variables that may be modified by hardware or interrupt service programs, such as sensor state. 2) Volatile cannot guarantee multi-thread safety, and should use mutex locks or atomic operations. 3) Using volatile may cause performance slight to decrease, but ensure program correctness.
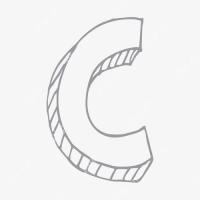
Measuring thread performance in C can use the timing tools, performance analysis tools, and custom timers in the standard library. 1. Use the library to measure execution time. 2. Use gprof for performance analysis. The steps include adding the -pg option during compilation, running the program to generate a gmon.out file, and generating a performance report. 3. Use Valgrind's Callgrind module to perform more detailed analysis. The steps include running the program to generate the callgrind.out file and viewing the results using kcachegrind. 4. Custom timers can flexibly measure the execution time of a specific code segment. These methods help to fully understand thread performance and optimize code.
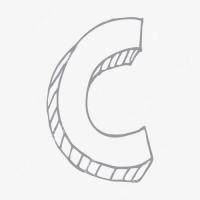
Using the chrono library in C can allow you to control time and time intervals more accurately. Let's explore the charm of this library. C's chrono library is part of the standard library, which provides a modern way to deal with time and time intervals. For programmers who have suffered from time.h and ctime, chrono is undoubtedly a boon. It not only improves the readability and maintainability of the code, but also provides higher accuracy and flexibility. Let's start with the basics. The chrono library mainly includes the following key components: std::chrono::system_clock: represents the system clock, used to obtain the current time. std::chron
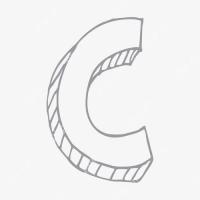
C performs well in real-time operating system (RTOS) programming, providing efficient execution efficiency and precise time management. 1) C Meet the needs of RTOS through direct operation of hardware resources and efficient memory management. 2) Using object-oriented features, C can design a flexible task scheduling system. 3) C supports efficient interrupt processing, but dynamic memory allocation and exception processing must be avoided to ensure real-time. 4) Template programming and inline functions help in performance optimization. 5) In practical applications, C can be used to implement an efficient logging system.
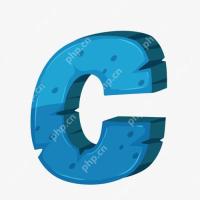
ABI compatibility in C refers to whether binary code generated by different compilers or versions can be compatible without recompilation. 1. Function calling conventions, 2. Name modification, 3. Virtual function table layout, 4. Structure and class layout are the main aspects involved.
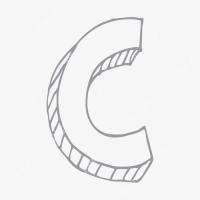
DMA in C refers to DirectMemoryAccess, a direct memory access technology, allowing hardware devices to directly transmit data to memory without CPU intervention. 1) DMA operation is highly dependent on hardware devices and drivers, and the implementation method varies from system to system. 2) Direct access to memory may bring security risks, and the correctness and security of the code must be ensured. 3) DMA can improve performance, but improper use may lead to degradation of system performance. Through practice and learning, we can master the skills of using DMA and maximize its effectiveness in scenarios such as high-speed data transmission and real-time signal processing.


Hot AI Tools

Undresser.AI Undress
AI-powered app for creating realistic nude photos

AI Clothes Remover
Online AI tool for removing clothes from photos.

Undress AI Tool
Undress images for free

Clothoff.io
AI clothes remover

Video Face Swap
Swap faces in any video effortlessly with our completely free AI face swap tool!

Hot Article

Hot Tools

MantisBT
Mantis is an easy-to-deploy web-based defect tracking tool designed to aid in product defect tracking. It requires PHP, MySQL and a web server. Check out our demo and hosting services.
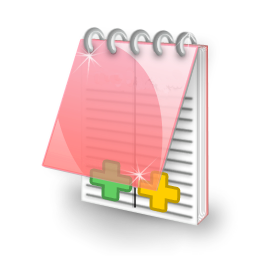
EditPlus Chinese cracked version
Small size, syntax highlighting, does not support code prompt function

SublimeText3 Chinese version
Chinese version, very easy to use
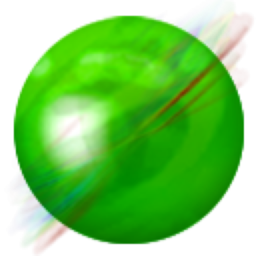
ZendStudio 13.5.1 Mac
Powerful PHP integrated development environment

SecLists
SecLists is the ultimate security tester's companion. It is a collection of various types of lists that are frequently used during security assessments, all in one place. SecLists helps make security testing more efficient and productive by conveniently providing all the lists a security tester might need. List types include usernames, passwords, URLs, fuzzing payloads, sensitive data patterns, web shells, and more. The tester can simply pull this repository onto a new test machine and he will have access to every type of list he needs.
