Autoencoder is an unsupervised learning algorithm that can learn the feature expression of input data and is widely used in deep learning. This article will introduce autoencoders in Python.
1. Introduction to Autoencoder
Autoencoder (Autoencoder) is a neural network that contains an encoder and a decoder. The encoder compresses the input data (such as images, text) into a small vector, and the decoder reconstructs the original input data based on this vector. Through this compression-reconstruction process, the autoencoder can learn a low-dimensional representation of the input data, that is, a feature expression.
The training process of the autoencoder is unsupervised and does not require labeled data. The principle is to minimize the reconstruction error between input and output, so that the encoder and decoder jointly learn the feature representation of the input data. The structure of autoencoders can be diversified, such as ordinary autoencoders, convolutional autoencoders, cyclic autoencoders, etc.
2. Implementing autoencoders in Python
Implementing autoencoders in Python usually uses deep learning frameworks, such as TensorFlow, Keras, PyTorch, etc. The following is a basic autoencoder example, implemented using Keras:
from keras.layers import Input, Dense from keras.models import Model # 定义编码器 input_img = Input(shape=(784,)) encoded = Dense(128, activation='relu')(input_img) encoded = Dense(64, activation='relu')(encoded) encoded_output = Dense(32, activation='relu')(encoded) # 定义解码器 decoded = Dense(64, activation='relu')(encoded_output) decoded = Dense(128, activation='relu')(decoded) decoded_output = Dense(784, activation='sigmoid')(decoded) # 定义自动编码器模型 autoencoder = Model(inputs=input_img, outputs=decoded_output) # 编译模型 autoencoder.compile(optimizer='adam', loss='binary_crossentropy') # 加载数据 from keras.datasets import mnist import numpy as np (x_train, _), (x_test, _) = mnist.load_data() x_train = x_train.astype('float32') / 255. x_test = x_test.astype('float32') / 255. x_train = x_train.reshape((len(x_train), np.prod(x_train.shape[1:]))) x_test = x_test.reshape((len(x_test), np.prod(x_test.shape[1:]))) # 训练模型 autoencoder.fit(x_train, x_train, epochs=50, batch_size=256, shuffle=True, validation_data=(x_test, x_test))
In this example, the Dense layer is used to define the encoder and decoder, and the activation functions are relu and sigmoid. Taking the MNIST handwritten digits data set as an example, the model is trained for 50 epochs. Through the model established through training, the low-dimensional feature representation of the data can be obtained through the encoder.
3. Application of Autoencoders
Autoencoders are widely used in feature learning, data dimensionality reduction, image compression and other fields. The following is an example of the application of autoencoders in image compression:
# 压缩图像 encoded_imgs = encoder.predict(x_test) # 解压缩图像 decoded_imgs = decoder.predict(encoded_imgs) # 可视化图像 import matplotlib.pyplot as plt n = 10 # 选择要可视化的图像数量 plt.figure(figsize=(20, 4)) for i in range(n): # 原始图像 ax = plt.subplot(2, n, i + 1) plt.imshow(x_test[i].reshape(28, 28)) plt.gray() ax.get_xaxis().set_visible(False) ax.get_yaxis().set_visible(False) # 压缩后的图像 ax = plt.subplot(2, n, i + 1 + n) plt.imshow(decoded_imgs[i].reshape(28, 28)) plt.gray() ax.get_xaxis().set_visible(False) ax.get_yaxis().set_visible(False) plt.show()
In the above example, the trained autoencoder is used to perform image compression on the MNIST handwritten digits data set, and the noise is removed during the compression and decompression process. , and through visualization, we can see that the compressed image is restored quite well.
4. Conclusion
Autoencoder is one of the very basic models in deep learning and is an essential step in understanding deep learning. It is very convenient to implement autoencoders in Python. You only need to choose a suitable deep learning framework, such as Keras, PyTorch, etc. Through autoencoders, we can learn important features of input data and implement applications such as image compression and feature learning.
The above is the detailed content of What are autoencoders in Python?. For more information, please follow other related articles on the PHP Chinese website!
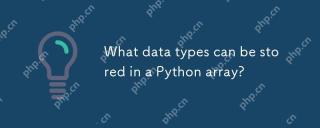
Pythonlistscanstoreanydatatype,arraymodulearraysstoreonetype,andNumPyarraysarefornumericalcomputations.1)Listsareversatilebutlessmemory-efficient.2)Arraymodulearraysarememory-efficientforhomogeneousdata.3)NumPyarraysareoptimizedforperformanceinscient
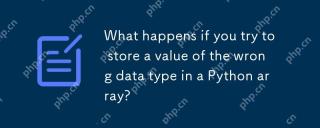
WhenyouattempttostoreavalueofthewrongdatatypeinaPythonarray,you'llencounteraTypeError.Thisisduetothearraymodule'sstricttypeenforcement,whichrequiresallelementstobeofthesametypeasspecifiedbythetypecode.Forperformancereasons,arraysaremoreefficientthanl
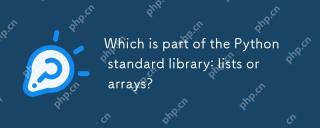
Pythonlistsarepartofthestandardlibrary,whilearraysarenot.Listsarebuilt-in,versatile,andusedforstoringcollections,whereasarraysareprovidedbythearraymoduleandlesscommonlyusedduetolimitedfunctionality.
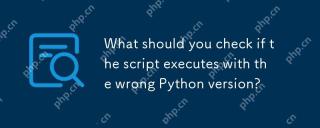
ThescriptisrunningwiththewrongPythonversionduetoincorrectdefaultinterpretersettings.Tofixthis:1)CheckthedefaultPythonversionusingpython--versionorpython3--version.2)Usevirtualenvironmentsbycreatingonewithpython3.9-mvenvmyenv,activatingit,andverifying
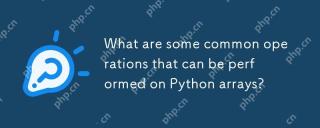
Pythonarrayssupportvariousoperations:1)Slicingextractssubsets,2)Appending/Extendingaddselements,3)Insertingplaceselementsatspecificpositions,4)Removingdeleteselements,5)Sorting/Reversingchangesorder,and6)Listcomprehensionscreatenewlistsbasedonexistin
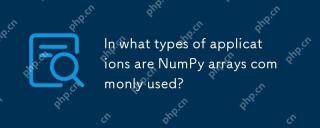
NumPyarraysareessentialforapplicationsrequiringefficientnumericalcomputationsanddatamanipulation.Theyarecrucialindatascience,machinelearning,physics,engineering,andfinanceduetotheirabilitytohandlelarge-scaledataefficiently.Forexample,infinancialanaly
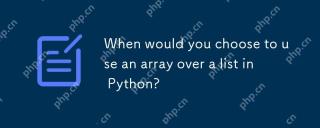
Useanarray.arrayoveralistinPythonwhendealingwithhomogeneousdata,performance-criticalcode,orinterfacingwithCcode.1)HomogeneousData:Arrayssavememorywithtypedelements.2)Performance-CriticalCode:Arraysofferbetterperformancefornumericaloperations.3)Interf
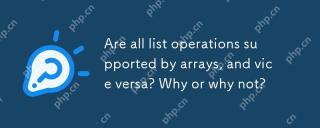
No,notalllistoperationsaresupportedbyarrays,andviceversa.1)Arraysdonotsupportdynamicoperationslikeappendorinsertwithoutresizing,whichimpactsperformance.2)Listsdonotguaranteeconstanttimecomplexityfordirectaccesslikearraysdo.


Hot AI Tools

Undresser.AI Undress
AI-powered app for creating realistic nude photos

AI Clothes Remover
Online AI tool for removing clothes from photos.

Undress AI Tool
Undress images for free

Clothoff.io
AI clothes remover

Video Face Swap
Swap faces in any video effortlessly with our completely free AI face swap tool!

Hot Article

Hot Tools

Notepad++7.3.1
Easy-to-use and free code editor

MantisBT
Mantis is an easy-to-deploy web-based defect tracking tool designed to aid in product defect tracking. It requires PHP, MySQL and a web server. Check out our demo and hosting services.

DVWA
Damn Vulnerable Web App (DVWA) is a PHP/MySQL web application that is very vulnerable. Its main goals are to be an aid for security professionals to test their skills and tools in a legal environment, to help web developers better understand the process of securing web applications, and to help teachers/students teach/learn in a classroom environment Web application security. The goal of DVWA is to practice some of the most common web vulnerabilities through a simple and straightforward interface, with varying degrees of difficulty. Please note that this software

mPDF
mPDF is a PHP library that can generate PDF files from UTF-8 encoded HTML. The original author, Ian Back, wrote mPDF to output PDF files "on the fly" from his website and handle different languages. It is slower than original scripts like HTML2FPDF and produces larger files when using Unicode fonts, but supports CSS styles etc. and has a lot of enhancements. Supports almost all languages, including RTL (Arabic and Hebrew) and CJK (Chinese, Japanese and Korean). Supports nested block-level elements (such as P, DIV),
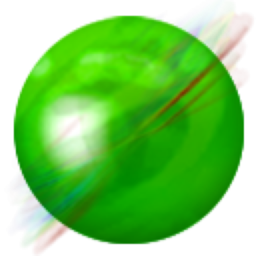
ZendStudio 13.5.1 Mac
Powerful PHP integrated development environment
