This article mainly introduces "MySQL index optimization methods". In daily operations, I believe many people have doubts about the MySQL index optimization methods. The editor has reviewed various materials and compiled them. Here is a simple and easy-to-use operation method. I hope it will help everyone answer your doubts about "MySQL index optimization method"! Next, please follow the editor to learn together!
1.Data preparation
#1.建立员工表,并创建name,age,position索引,id为自增主键 CREATE TABLE `employees` ( `id` int(11) NOT NULL AUTO_INCREMENT, `name` varchar(24) NOT NULL DEFAULT '' COMMENT '姓名', `age` int(11) NOT NULL DEFAULT '0' COMMENT '年龄', `position` varchar(20) NOT NULL DEFAULT '' COMMENT '职位', `hire_time` timestamp NOT NULL DEFAULT CURRENT_TIMESTAMP COMMENT '入职时间', PRIMARY KEY (`id`), KEY `idx_name_age_position` (`name`,`age`,`position`) USING BTREE ) ENGINE=InnoDB AUTO_INCREMENT=100010 DEFAULT CHARSET=utf8 COMMENT='员工记录表' # 2.前面插入三条数据,并建立employees_min_copy表插入这三条数据 INSERT INTO employees (name,age,`position`,hire_time) VALUES ('LiLei',22,'manager','2021-08-17 21:00:55') ,('HanMeimei',23,'dev','2021-08-17 21:00:55') ,('Lucy',23,'dev','2021-08-17 21:00:55') ; #3.再通过执行计划向表中插入十万条数据 #3.1建立存储过程,往employees表中插入数据(MySQL8.0版本) DELIMITER $$ USE `zhebase`$$ DROP PROCEDURE IF EXISTS `batch_insert_employees`$$ CREATE PROCEDURE `batch_insert_employees`(IN `start_number` BIGINT,IN `counts` BIGINT) BEGIN DECLARE start_number BIGINT DEFAULT start_number; DECLARE stop_number BIGINT DEFAULT start_number; SET stop_number=start_number + counts; WHILE start_number < stop_number DO INSERT INTO employees(name,age,position,hire_time) VALUES(CONCAT('zhang',start_number),start_number,'dev',now()); SET start_number=start_number+1; END WHILE ; COMMIT; END$$ DELIMITER ; #3.2执行存储过程插入十万条数据 CALL batch_insert_employees(1,100000);
2.Example 1
1.Joint index No. A field using a range will not go into the index
EXPLAIN SELECT * FROM employees WHERE name > 'LiLei' AND age = 22 AND position ='manager';
Reason: MySQL may internally think that the first field should use a range, and the result set should be very large, and it needs to be Returning to the table is not efficient. It is better to use full table scan directly. But we can force the index to be used
EXPLAIN SELECT * FROM employees force index(idx_name_age_position) WHERE name > 'LiLei' AND age = 22 AND position ='manager';
-- 关闭查询缓存 set global query_cache_size=0; set global query_cache_type=0; -- 执行时间0.321s SELECT * FROM employees WHERE name > 'LiLei'; -- 执行时间0.458s SELECT * FROM employees force index(idx_name_age_position) WHERE name > 'LiLei';
Forced indexing is used to allow the first field range search of the joint index to also use the index. The scanned rows seem to be less, but the final search efficiency is not necessarily higher than the full table scan, because the table return efficiency is not high
In this case, if you can use a covering index, use the covering index for optimizationEXPLAIN SELECT name,age,position FROM employees WHERE name > 'LiLei' AND age = 22 AND position ='manager';
2.in and or in the table data If the volume is relatively large, the index will be used. If there are not many table records, a full table scan will be selected
EXPLAIN SELECT * FROM employees WHERE name in ('LiLei','HanMeimei','Lucy') AND age = 22 AND position ='manager'; #表数据量大走索引,数据量小全表扫描 EXPLAIN SELECT * FROM employees WHERE (name = 'LiLei' or name = 'HanMeimei') AND age = 22 AND position ='manager';
3 .like xx% Regardless of the amount of data, the index will generally be used
EXPLAIN SELECT * FROM employees WHERE name like 'LiLei%' AND age = 22 AND position ='manager';
Index Condition Pushdown (ICP) To optimize like xx%.
Index pushdown: For the auxiliary joint index (idx_name_age_position), usually according to the leftmost prefix principle, SELECT * FROM employees WHERE name like 'LiLei%' AND age = 22 AND position ='manager' Because
name is a range query, after filtering, age and position are unordered, subsequent indexes cannot be used, only the name field will be used index.
Before MySQL5.6: First match the index whose name starts with 'LiLei' in the index tree, and then perform the table return operation based on the primary key under the index. On the primary key index, match age and position
- ##MySQL 5.6 and later:
Introduce index pushdown, first match the index whose name starts with 'LiLei' in the index tree, and at the same time Judge all the conditional fields that exist in the database, filter out the records that do not meet the conditions, and then return the table to match other conditions and query the entire row of data.
- Advantages:
Filtering out records that do not meet the conditions before returning to the table can effectively reduce the number of table returns and improve query efficiency.
It may be because the range search result set is generally larger, like xx% in most cases, the result set after filtering is smaller. When the result set is large, subsequent fields must be matched every time it is retrieved, which is not necessarily faster than returning the table immediately. But it is not absolute. Sometimes Like xx% will not be pushed down the index. 3.How does MySQL choose the appropriate index?
Let’s first look at two SQL statements:# MySQL直接使用全表扫描
EXPLAIN select * from employees where name > 'a';
# MySQL走索引
EXPLAIN select * from employees where name > 'zzz';
We found that the first SQL performed a full table scan, and the second SQL has gone to the index. Corresponding to the first SQL, MySQL calculated the execution cost and found that the cost of indexing was higher than that of a full scan (indexing requires traversing the name field and then performing a table return operation to find the final data, which is slower than directly querying the clustered index tree). In this case
. As for how MySQL selects the final index, you can use the 下面是执行后的Trace中的内容: 由 Trace字段可知,全表扫描的 cost_for_plan = 9394.9 小于使用索引 cost_for_plan = 16311,故最终选择全表扫描。 分析: 案例1 由最左前缀法则分析出索引中间不能出现断层,只使用了 name 索引前缀,也可以从key_len = 3n + 2 看出。age 索引列用在排序过程中,因为Extra字段里没有 Using filesort 而是 Using index condition 。 分析: 案例2 索引查询使用了 name 索引前缀,但排序由于跳过了 age 所以Extra字段出现了 Using filesort 。 分析: 案例3 查询时使用了 name 索引,age 和 postion 用于排序,不会出现 Using filesort 分析: 案例4 查询时使用了 name 索引,age 和 postion 顺序与创建索引树不一致,出现了 Using filesort 分析: 案例5 查询时使用了 name 索引,age 和 postion 顺序与创建索引树不一致,但 name、age 为常量,MySQL 会自动优化,不会出现 Using filesort 分析: 案例6 排序顺序一样,但 order by 默认升序,导致与索引的排序方式不同,出现了 Using filesort 。 MySQL8.0 以上版本有降序索引可以支持这种查询。 分析: 案例7 对于排序来说,多个相等条件也是范围查询,出现了 Using filesort 。 分析: 案例8 原因同前面的例子,可以使用覆盖索引优化。 MySQL排序总结: 1、MySQL支持两种方式的排序 filesort 和 index,Using index是指MySQL扫描索引本身完成排序。Using filesort 是指MySQL扫描聚簇索引(整张表)进行排序。index效率高,filesort效率低。 2、order by 满足两种情况会使用 Using index(不绝对) a.order by 语句使用索引最左前列。 b.使用 where 子句与 order by 子句条件列组合满足索引最左前列。 3、尽量在索引列上完成排序,遵循最左前缀法则。 4、如果 order by 的条件不在索引列上,就会产生Using filesort。 5、能用覆盖索引尽量用覆盖索引 6、group by 与 order by 很类似,其实质是先排序后分组(group by 底层:先执行一次 order by 再进行分组),遵照索引创建顺序的最左前缀法则。对于group by的优化如果不需要排序的可以加上order by null 禁止排序。注意,where高于having,能写在where中的限定条件就不要去having限定了。 Using filesort 文件排序原理 filesort文件排序方式有: 单路排序:是一次性取出满足条件行的所有字段,然后在 sort buffer 中进行排序。用trace工具得到sort_mode信息显示或者 双路排序(又叫回表排序模式) :先根据相应的条件取出相应的排序字段和可以直接定位行数据的行 ID,然后在 sort buffer 中进行排序,排序完后需要再次取回其它需要的字段。用trace工具得到sort_mode信息显示 MySQL 通过比较系统变量 max_length_for_sort_data(默认1024字节) 的大小和需要查询的字段总大小来判断使用哪种排序模式。 字段的总长度 字段的总长度 >max_length_for_sort_data ,使用双路排序 单路排序会把所有需要查询的字段都放到 sort buffer 中排序,而双路排序只会把主键和需要排序的字段放到 sort buffer 中进行排序,然后再通过主键回到原表查询需要的字段。 单路排序过程: a.从索引 name 找到第一个满足 name = 'Lucy' 条件的主键 id b.回表根据主键 id 取出整行,取出所有字段的值,存入 sort_buffer 中 c.从索引name找到下一个满足 name = 'Lucy' 条件的主键 id d.重复步骤 2、3 直到不满足 name = 'Lucy' e.对 sort_buffer 中的数据按照字段 position 进行排序 f.返回结果 双路排序过程: a.从索引 name 找到第一个满足 name ='Lucy' 的主键 id b.根据主键 id 取出整行,把排序字段 position 和主键 id 这两个字段放到 sort buffer 中 c.从索引 name 取下一个满足 name = 'Lucy' 记录的主键 id d.重复 3、4 直到不满足 name = 'Lucy' e.对 sort_buffer 中的字段 position 和主键 id 按照字段 position 进行排序 f.遍历排序好的 id 和字段 position,按照 id 的值回到原表中取出所有字段的值返回 这条 SQL 语句实际查询了 10010 条记录,然后丢弃了前面的 10000 条记录,所以,在 数据量很大时,执行效率是非常非常低的。一般需要对分页查询进行优化。 优化方法: 1.根据自增且连续的主键排序的分页查询 当一个表的主键连续且自增时,可以使用该方法进行优化,但如果自增不连续会造成数据丢失。 2.根据非主键字段排序的分页查询 先通过排序和分页操作先查出主键,然后根据主键查出对应的记录。 嵌套循环连接 Nested-Loop Join(NLJ) 算法 基于块的嵌套循环连接 Block Nested-Loop Join(BNL)算法 通常,当MySQL查询被驱动表的关联字段没有索引时,会采用BNL算法进行关联查询。当存在索引时,通常会选择NLJ算法,因为 NLJ 算法在有索引的情况下比 BNL 算法效率更高 1.嵌套循环连接 Nested-Loop Join(NLJ) 算法 原理:一次一行循环地从第一张表(驱动表)中读取行,在这行数据中取到关联字段,根据关联字段在另一张表(被驱动表)里取出满足条件的行,然后取出两张表的结果合集。 从执行计划可以了解的信息: a.驱动表是 t2,被驱动表是 t1( inner join 时 SQL优化器会小表驱动大表,外连接则根据连接类型区分) b.使用了 NLJ 算法。如果执行计划 Extra 中未出现 Using join buffer 则表示使用的 join 算法是 NLJ 整个过程会读取 t2 表的所有数据(扫描100行),然后遍历这每行数据中字段 a 的值,根据 t2 表中 a 的值索引扫描 t1 表中的对应行(扫描100次 t1 表的索引,1次扫描可以认为最终只扫描 t1 表一行完整数据,也就是总共 t1 表也扫描了100行)。因此整个过程扫描了 200 行 。 2. 基于块的嵌套循环连接 Block Nested-Loop Join(BNL)算法 原理:把驱动表的数据读入到 join_buffer 中,然后扫描被驱动表,把被驱动表每一行取出来跟 join_buffer 中的数据做对比 整个过程对表 t1 和 t2 都做了一次全表扫描,因此扫描的总行数为10000(表 t1 的数据总量) + 100(表 t2 的数据总量) = 10100。并且 join_buffer 里的数据是无序的,因此对表 t1 中的每一行,都要做 100 次判断,所以内存中的判断次数是 100 * 10000= 100 万次(非扫描次数) 。 注意: join_buffer 的大小是由参数 join_buffer_size 控制,默认256k。如果 t2 放不下就会使用分段策略(先从 t2 表取出部分数据,比对完就清空 join_buffer,再重新拿出来余下的部分进行比对)。 被驱动表的关联字段无索引为什么要选择使用 BNL 算法而不使用 NLJ 算法? 如第二条 SQL,如果使用 NLJ 算法扫描行数为 100 * 10000 = 100万,这个是磁盘扫描。使用 BNL 算法仅需扫描 100100 行。 对于表关联 SQL 的优化 尽量少关联(在阿里规范中,关联表不能超过三种,可以后端代码单独查询,循环关联) 小表驱动大表,写多表连接 SQL 时如果明确知道哪张表是小表可以用straight_join写法固定连接驱动方式,节约 MySQL 优化器判断时间. 关联字段加索引,大表关联字段一定要加索引,尽量使得 MySQL 在进行 join 操作时选择NLJ算法 多表连接是非常难以优化的,最好95%的场景都使用单表来完成,复杂场景交个JAVA代码,大规模计算交给大数据工具,无需效率才考虑连接 原则:小表驱动大表 注意:根据某个字段 count 不会统计字段为 null 的行 四条语句的效率几乎可以忽略,效率对比如下: 字段有索引: count(* )≈count(1)>count(字段)>count(主键 id) 段)>count(主键 id) 字段无索引: count(*)≈count(1)>count(主键 id)>count(字段) 常见优化方法: 1.对于 MyISAM 存储引擎的表做不带 where 条件的 count 查询性能是很高的,数据总行数直接写在磁盘上,查询不需要计算。innodb 存储引擎的表则不会记录(因为有MVCC机制) 2.对与不用知道确切行的可以直接使用 3.将总数维护到 Redis 里面,插入或删除表数据行的时候同时维护 Redis 里的表总行数 key 的计数值(用 incr 或 decr 命令),但是这种方式可能不准,很难保证表操作和Redis 操作的事务一致性。 4.增加数据库计数表,插入或删除表数据行的时候同时维护计数表,且它们在同一个事务里操作 1、代码先行,索引后上,先开发完主体业务代码,再把涉及到该表相关sql都要拿出来分析之后再建立索引。 2、联合索引尽量覆盖条件,可以设计一个或者两三个联合索引(单值索引要少建),让每一个联合索引都尽量去包含SQL语句里的 where、order by、group by 的字段,且这些联合索引字段顺序尽量满足 SQL查询的最左前缀原则。 3、不要在小基数字段上建立索引,无法进行快速的二分查找,不能能发挥出B+树快速二分查找的优势来,没有意义 4、尽量对字段类型较小的列设计索引,尽量对字段类型较小的列设计索引,比如 Tinyint 之类,字段类型较小的话,占用磁盘空间小,搜索的时性能更好。 5、长字符串可以采用前缀索引,比如针对某个字段的前20个字符建立索引,即:每个值的前20个字符放入索引树中,搜索时会先匹配前而是个字符,再回表到聚簇索引取出来完整的 name 字段值进行比较。但排序(order by 和 group by)时无法使用该索引。 6、where 与 order by 冲突时优先 where,大多数情况下根据索引进行 where 筛选一般筛选出来的数据比较少,然后做排序成本会更低。 7. Optimization based on slow SQL queries You can do specific index optimization for these slow SQL queries based on some slow SQL in the monitoring background (MySQL provides it, just Set specific parameters). Trace tool
to view it. Butturning on the trace tool will affect MySQL performance and needs to be closed immediately after use. #开启trace
set session optimizer_trace="enabled=on",end_markers_in_json=on;
#关闭trace
set session optimizer_trace="enabled=off";
#使用trace
select * from employees where name > 'a' order by position;
select * from information_schema.OPTIMIZER_TRACE;
{
"steps": [
{
#第一阶段:SQL准备阶段,格式化sql
"join_preparation": {
"select#": 1,
"steps": [
{
"expanded_query": "/* select#1 */ select `employees`.`id` AS `id`,`employees`.`name` AS `name`,`employees`.`age` AS `age`,`employees`.`position` AS `position`,`employees`.`hire_time` AS `hire_time` from `employees` where (`employees`.`name` > 'a') order by `employees`.`position` limit 0,200"
}
] /* steps */
} /* join_preparation */
},
{
#第二阶段:SQL优化阶段
"join_optimization": {
"select#": 1,
"steps": [
{
#条件处理
"condition_processing": {
"condition": "WHERE",
"original_condition": "(`employees`.`name` > 'a')",
"steps": [
{
"transformation": "equality_propagation",
"resulting_condition": "(`employees`.`name` > 'a')"
},
{
"transformation": "constant_propagation",
"resulting_condition": "(`employees`.`name` > 'a')"
},
{
"transformation": "trivial_condition_removal",
"resulting_condition": "(`employees`.`name` > 'a')"
}
] /* steps */
} /* condition_processing */
},
{
"substitute_generated_columns": {
} /* substitute_generated_columns */
},
{
#表依赖详情
"table_dependencies": [
{
"table": "`employees`",
"row_may_be_null": false,
"map_bit": 0,
"depends_on_map_bits": [
] /* depends_on_map_bits */
}
] /* table_dependencies */
},
{
"ref_optimizer_key_uses": [
] /* ref_optimizer_key_uses */
},
{
#预估表的访问成本
"rows_estimation": [
{
"table": "`employees`",
"range_analysis": {
"table_scan": { --全表扫描情况
"rows": 93205, --扫描行数
"cost": 9394.9 --查询成本
} /* table_scan */,
#查询可能使用的索引
"potential_range_indexes": [
{
"index": "PRIMARY", --主键索引
"usable": false, -- 是否使用
"cause": "not_applicable"
},
{
#辅助索引
"index": "idx_name_age_position",
"usable": true,
"key_parts": [
"name",
"age",
"position",
"id"
] /* key_parts */
}
] /* potential_range_indexes */,
"setup_range_conditions": [
] /* setup_range_conditions */,
"group_index_range": {
"chosen": false,
"cause": "not_group_by_or_distinct"
} /* group_index_range */,
"skip_scan_range": {
"potential_skip_scan_indexes": [
{
"index": "idx_name_age_position",
"usable": false,
"cause": "query_references_nonkey_column"
}
] /* potential_skip_scan_indexes */
} /* skip_scan_range */,
#分析各个索引使用成本
"analyzing_range_alternatives": {
"range_scan_alternatives": [
{
"index": "idx_name_age_position",
"ranges": [
"a < name" --索引使用范围
] /* ranges */,
"index_dives_for_eq_ranges": true,
"rowid_ordered": false, --使用该索引获取的记录是否按照主键排序
"using_mrr": false,
"index_only": false, --是否使用覆盖索引
"rows": 46602, --索引扫描行数
"cost": 16311, --索引使用成本
"chosen": false, --是否选择该索引
"cause": "cost"
}
] /* range_scan_alternatives */,
"analyzing_roworder_intersect": {
"usable": false,
"cause": "too_few_roworder_scans"
} /* analyzing_roworder_intersect */
} /* analyzing_range_alternatives */
} /* range_analysis */
}
] /* rows_estimation */
},
{
"considered_execution_plans": [
{
"plan_prefix": [
] /* plan_prefix */,
"table": "`employees`",
"best_access_path": { --最优访问路径
"considered_access_paths": [ --最终选择的访问路径
{
"rows_to_scan": 93205,
"filtering_effect": [
] /* filtering_effect */,
"final_filtering_effect": 0.5,
"access_type": "scan", --访问类型:为scan,全表扫描
"resulting_rows": 46602,
"cost": 9392.8,
"chosen": true --确定选择
}
] /* considered_access_paths */
} /* best_access_path */,
"condition_filtering_pct": 100,
"rows_for_plan": 46602,
"cost_for_plan": 9392.8,
"chosen": true
}
] /* considered_execution_plans */
},
{
"attaching_conditions_to_tables": {
"original_condition": "(`employees`.`name` > 'a')",
"attached_conditions_computation": [
{
"table": "`employees`",
"rechecking_index_usage": {
"recheck_reason": "low_limit",
"limit": 200,
"row_estimate": 46602
} /* rechecking_index_usage */
}
] /* attached_conditions_computation */,
"attached_conditions_summary": [
{
"table": "`employees`",
"attached": "(`employees`.`name` > 'a')"
}
] /* attached_conditions_summary */
} /* attaching_conditions_to_tables */
},
{
"optimizing_distinct_group_by_order_by": {
"simplifying_order_by": {
"original_clause": "`employees`.`position`",
"items": [
{
"item": "`employees`.`position`"
}
] /* items */,
"resulting_clause_is_simple": true,
"resulting_clause": "`employees`.`position`"
} /* simplifying_order_by */
} /* optimizing_distinct_group_by_order_by */
},
{
"reconsidering_access_paths_for_index_ordering": {
"clause": "ORDER BY",
"steps": [
] /* steps */,
"index_order_summary": {
"table": "`employees`",
"index_provides_order": false,
"order_direction": "undefined",
"index": "unknown",
"plan_changed": false
} /* index_order_summary */
} /* reconsidering_access_paths_for_index_ordering */
},
{
"finalizing_table_conditions": [
{
"table": "`employees`",
"original_table_condition": "(`employees`.`name` > 'a')",
"final_table_condition ": "(`employees`.`name` > 'a')"
}
] /* finalizing_table_conditions */
},
{
"refine_plan": [
{
"table": "`employees`"
}
] /* refine_plan */
},
{
"considering_tmp_tables": [
{
"adding_sort_to_table_in_plan_at_position": 0
} /* filesort */
] /* considering_tmp_tables */
}
] /* steps */
} /* join_optimization */
},
{
#第三阶段:SQL执行阶段
"join_execution": {
"select#": 1,
"steps": [
{
"sorting_table_in_plan_at_position": 0,
"filesort_information": [
{
"direction": "asc",
"table": "`employees`",
"field": "position"
}
] /* filesort_information */,
"filesort_priority_queue_optimization": {
"limit": 200,
"chosen": true
} /* filesort_priority_queue_optimization */,
"filesort_execution": [
] /* filesort_execution */,
"filesort_summary": {
"memory_available": 262144,
"key_size": 40,
"row_size": 186,
"max_rows_per_buffer": 201,
"num_rows_estimate": 285696,
"num_rows_found": 100003,
"num_initial_chunks_spilled_to_disk": 0,
"peak_memory_used": 38994,
"sort_algorithm": "std::stable_sort",
"unpacked_addon_fields": "using_priority_queue",
"sort_mode": "<fixed_sort_key, additional_fields>"
} /* filesort_summary */
}
] /* steps */
} /* join_execution */
}
] /* steps */
}
4.常见 SQL 深入优化
4.1.Order by与Group by优化
# 案例1
explain select * from employees where name = 'Lucy' and position = 'dev' order by age;
#案例2
explain select * from employees where name = 'Lucy' order by position;
#案例3
explain select * from employees where name = 'Lucy' order by age, position;
#案例4
explain select * from employees where name = 'Lucy' order by position,age;
#案例5
explain
select * from employees
where name = 'Lucy'
and age = 22
order by position,age;
#案例6
explain select * from employees where name = 'Lucy' order byage,position desc;
#案例7
explain select * from employees where name = 'Lucy' or name = 'LiLei' order by age;
#案例8
#SQL-1
explain select * from employees where name > 'zzz' order by name;
#SQL-2
explain select * from employees where name > 'a' order by name;
select * from employees where name = 'Lucy' order by position;
"join_execution": { --Sql执行阶段
"select#": 1,
"steps": [
{
"filesort_information": [
{
"direction": "asc",
"table": "`employees`",
"field": "position"
}
] /* filesort_information */,
"filesort_priority_queue_optimization": {
"usable": false,
"cause": "not applicable (no LIMIT)"
} /* filesort_priority_queue_optimization */,
"filesort_execution": [
] /* filesort_execution */,
"filesort_summary": { --文件排序信息
"rows": 10000, --预计扫描行数
"examined_rows": 10000, --参与排序的行
"number_of_tmp_files": 3, --使用临时文件的个数,如果为0代表全部使用的sort_buffer内存排序,否则使用的磁盘文件排序
"sort_buffer_size": 262056, --排序缓存的大小,单位Byte
"sort_mode": "<sort_key, packed_additional_fields>" --排序方式,此处是路排序
} /* filesort_summary */
}
] /* steps */
} /* join_execution */
4.2.分页查询优化
select * from employees limit 10000,10
select * from employees where id > 90000 limit 5;
#优化前
select * from employees ORDER BY name limit 90000,5;
#优化后
select * from employees e
inner join (select id from employees order by name limit 90000,5) ed
on e.id = ed.id;
4.3.join关联查询优化
4.3.1.数据准备
#示例表
# 创建t1,t2表,主键id,单值索引a
CREATE TABLE `t1` (
`id` int(11) NOT NULL AUTO_INCREMENT,
`a` int(11) DEFAULT NULL,
`b` int(11) DEFAULT NULL,
PRIMARY KEY (`id`),
KEY `idx_a` (`a`)
) ENGINE=InnoDB DEFAULT CHARSET=utf8;
create table t2 like t1;
#存储过程往t1,t2表插入数据
DELIMITER $$
USE `zhebase`$$
DROP PROCEDURE IF EXISTS `batch_insert_t1`$$
CREATE PROCEDURE `batch_insert_t1`(IN `start_number` BIGINT,IN `counts` BIGINT)
BEGIN
DECLARE start_number BIGINT DEFAULT start_number;
DECLARE stop_number BIGINT DEFAULT start_number;
SET stop_number=start_number + counts;
WHILE start_number < stop_number DO
INSERT INTO t1(a,b) VALUES(start_number,start_number);
SET start_number=start_number+1;
END WHILE ;
COMMIT;
END$$
DELIMITER ;
DELIMITER $$
USE `zhebase`$$
DROP PROCEDURE IF EXISTS `batch_insert_t2`$$
CREATE PROCEDURE `batch_insert_t2`(IN `start_number` BIGINT,IN `counts` BIGINT)
BEGIN
DECLARE start_number BIGINT DEFAULT start_number;
DECLARE stop_number BIGINT DEFAULT start_number;
SET stop_number=start_number + counts;
WHILE start_number < stop_number DO
INSERT INTO t2(a,b) VALUES(start_number,start_number);
SET start_number=start_number+1;
END WHILE ;
COMMIT;
END$$
DELIMITER ;
#执行存储过程往t1表插入10000条记录,t2表插入100条记录
CALL batch_insert_t1(1,10000);
CALL batch_insert_t2(1,100);
4.3.2.MySQL 表关联常见的两种算法
explain select * from t1 inner join t2 on t1.a= t2.a;
explain select * from t1 inner join t2 on t1.b= t2.b;
select * from t2 straight_join t1 on t2.a = t1.a; 代表指定mysql选着 t2 表作为驱动表
4.4.in和exsits优化
# in 先执行括号里面的
select * from A where id in (select id from B)
#exists 先执行括号外面的
#select * 可以用 select 1 替换,没有区别
#exists 子查询内部会进行优化,并非逐条对比
#exists 子查询往往也可以用 jion 来代替,何种最优需要具体问题具体分析
select * from A where exists (select 1 from B where B.id = A.id)
4.5.count(*)查询优化
#扫描二级索引,按行累加
explain select count(1) from employees;
#扫描辅助索引按行累加(辅助索引比聚簇索引小)
explain select count(id) from employees;
#把 name 拿到内存,不为 null 就累加
explain select count(name) from employees;
#不取值,按行累加
explain select count(*) from employees;
show table status
,它是一个估值,使用该查询效率很高5.索引设计原则
The above is the detailed content of MySQL index optimization method. For more information, please follow other related articles on the PHP Chinese website!

How to effectively monitor MySQL performance? Use tools such as mysqladmin, SHOWGLOBALSTATUS, PerconaMonitoring and Management (PMM), and MySQL EnterpriseMonitor. 1. Use mysqladmin to view the number of connections. 2. Use SHOWGLOBALSTATUS to view the query number. 3.PMM provides detailed performance data and graphical interface. 4.MySQLEnterpriseMonitor provides rich monitoring functions and alarm mechanisms.
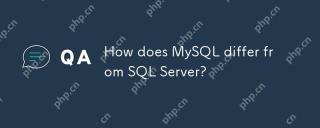
The difference between MySQL and SQLServer is: 1) MySQL is open source and suitable for web and embedded systems, 2) SQLServer is a commercial product of Microsoft and is suitable for enterprise-level applications. There are significant differences between the two in storage engine, performance optimization and application scenarios. When choosing, you need to consider project size and future scalability.
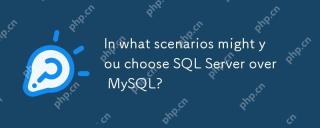
In enterprise-level application scenarios that require high availability, advanced security and good integration, SQLServer should be chosen instead of MySQL. 1) SQLServer provides enterprise-level features such as high availability and advanced security. 2) It is closely integrated with Microsoft ecosystems such as VisualStudio and PowerBI. 3) SQLServer performs excellent in performance optimization and supports memory-optimized tables and column storage indexes.
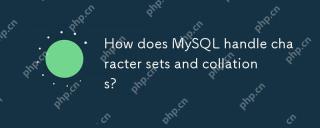
MySQLmanagescharactersetsandcollationsbyusingUTF-8asthedefault,allowingconfigurationatdatabase,table,andcolumnlevels,andrequiringcarefulalignmenttoavoidmismatches.1)Setdefaultcharactersetandcollationforadatabase.2)Configurecharactersetandcollationfor
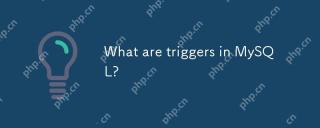
A MySQL trigger is an automatically executed stored procedure associated with a table that is used to perform a series of operations when a specific data operation is performed. 1) Trigger definition and function: used for data verification, logging, etc. 2) Working principle: It is divided into BEFORE and AFTER, and supports row-level triggering. 3) Example of use: Can be used to record salary changes or update inventory. 4) Debugging skills: Use SHOWTRIGGERS and SHOWCREATETRIGGER commands. 5) Performance optimization: Avoid complex operations, use indexes, and manage transactions.
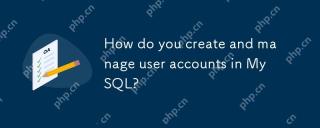
The steps to create and manage user accounts in MySQL are as follows: 1. Create a user: Use CREATEUSER'newuser'@'localhost'IDENTIFIEDBY'password'; 2. Assign permissions: Use GRANTSELECT, INSERT, UPDATEONmydatabase.TO'newuser'@'localhost'; 3. Fix permission error: Use REVOKEALLPRIVILEGESONmydatabase.FROM'newuser'@'localhost'; then reassign permissions; 4. Optimization permissions: Use SHOWGRA
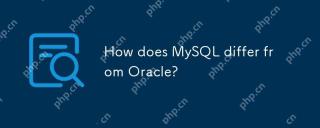
MySQL is suitable for rapid development and small and medium-sized applications, while Oracle is suitable for large enterprises and high availability needs. 1) MySQL is open source and easy to use, suitable for web applications and small and medium-sized enterprises. 2) Oracle is powerful and suitable for large enterprises and government agencies. 3) MySQL supports a variety of storage engines, and Oracle provides rich enterprise-level functions.
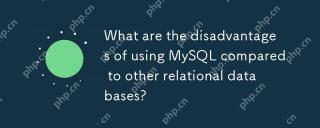
The disadvantages of MySQL compared to other relational databases include: 1. Performance issues: You may encounter bottlenecks when processing large-scale data, and PostgreSQL performs better in complex queries and big data processing. 2. Scalability: The horizontal scaling ability is not as good as Google Spanner and Amazon Aurora. 3. Functional limitations: Not as good as PostgreSQL and Oracle in advanced functions, some functions require more custom code and maintenance.


Hot AI Tools

Undresser.AI Undress
AI-powered app for creating realistic nude photos

AI Clothes Remover
Online AI tool for removing clothes from photos.

Undress AI Tool
Undress images for free

Clothoff.io
AI clothes remover

Video Face Swap
Swap faces in any video effortlessly with our completely free AI face swap tool!

Hot Article

Hot Tools

SAP NetWeaver Server Adapter for Eclipse
Integrate Eclipse with SAP NetWeaver application server.
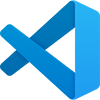
VSCode Windows 64-bit Download
A free and powerful IDE editor launched by Microsoft

SecLists
SecLists is the ultimate security tester's companion. It is a collection of various types of lists that are frequently used during security assessments, all in one place. SecLists helps make security testing more efficient and productive by conveniently providing all the lists a security tester might need. List types include usernames, passwords, URLs, fuzzing payloads, sensitive data patterns, web shells, and more. The tester can simply pull this repository onto a new test machine and he will have access to every type of list he needs.

Notepad++7.3.1
Easy-to-use and free code editor

Safe Exam Browser
Safe Exam Browser is a secure browser environment for taking online exams securely. This software turns any computer into a secure workstation. It controls access to any utility and prevents students from using unauthorized resources.