1. Python Cache
① Cache function
Cache is an optimization technology that can be used in applications to store recently or frequently used data Stored in memory, the speed of accessing data in this way is much higher than directly reading disk files.
Suppose we build a news aggregation website, similar to Feedly, which fetches news from different sources and then aggregates and displays it. When the user browses the news, the background program downloads the article and displays it on the user's screen. If caching technology is not used, when users switch to browse the same article multiple times, they must download it multiple times, which is inefficient and unfriendly.
A better approach is to store the content locally after obtaining each article, such as in a database; then, when the user opens the same article next time, Background programs can open content from local storage instead of downloading the source file again, a technique called caching.
② Use Python dictionary to implement caching
Take the news aggregation website as an example. It is not necessary to download the article content every time, but first check whether there is a correspondence in the cache data content, and only when there is no content, will the server download the article.
The following example program uses a Python dictionary to implement caching, using the URL of the article as the key and its content as the value; after execution, you can see that when the get_article function is executed for the second time, the Just return the result without letting the server download it:
import requests cache = dict() def get_article_from_server(url): print("Fetching article from server...") response = requests.get(url) return response.text def get_article(url): print("Getting article...") if url not in cache: cache[url] = get_article_from_server(url) return cache[url] get_article("https://www.escapelife.site/love-python.html") get_article("https://www.escapelife.site/love-python.html")
Save this code to a cache.py file, install the requests library, and then run the script:
# 安装依赖 $ pip install requests # 执行脚本 $ python python_caching.py Getting article... Fetching article from server... Getting article...
Despite calling get_article() twice (Lines 17 and 18) The string "Fetching article from server…", but still only output once. This happens because after accessing the article for the first time, its URL and content are put into the cache dictionary, and the second time the code does not need to fetch the item from the server again.
③ Disadvantages of using dictionaries for caching
There is a very big problem with the above caching implementation, that is, the content of the dictionary will grow infinitely, that is, when a large number of users continuously browse articles , the background program will continue to stuff content that needs to be stored into the dictionary, and the server memory will be crowded, eventually causing the application to crash.
There is a very big problem with the above caching implementation, that is, the content of the dictionary will grow infinitely, that is, when a large number of users continuously browse articles, the background program will continue to add data to the dictionary. Cramming in the content that needs to be stored, the server's memory is filled, eventually causing the application to crash.
To solve the above problem, we need a strategy to decide which articles should remain in memory and which articles should be deleted. These caching strategies are actually algorithms that use Used to manage cached information and choose which items to discard to make room for new items.
Of course you don't have to implement an algorithm to manage the cache, you just need to use different strategies to remove items from the cache and prevent it from growing beyond its maximum size. Five common caching algorithms are as follows:
Caching Strategy | English name | Elimination Condition | When is it most useful |
---|---|---|---|
First-in-first-out algorithm (FIFO) | First-In/First-Out | Eliminate the oldest entries | Newer entries are most likely to be reused |
Last-in-first-out algorithm (LIFO) | Last -In/First-Out | Eliminate the newest entries | Older entries are most likely to be reused |
Least Recently Used Algorithm (LRU) | Least Recently Used | Eliminate the least recently used entry | The most recently used entry is most likely to be reused |
Most recently used Usage algorithm (MRU) | Most Recently Used | Eliminate the most recently used entries | The recently unused entries are most likely to be reused |
Least Frequently Used Algorithm (LFU) | Least Frequently Used | Eliminate the least frequently accessed entries | Items with a high hit rate are more likely to be reused |
After reading the above five caching algorithms, are you a little confused when you see LRU and LFU? The main reason is that it is difficult to understand its true meaning through the corresponding explanation in Chinese. It is not difficult to understand it in English. The difference between LRU and LFU algorithms is:
LRU has an elimination rule based on access time, which eliminates data based on the historical access records of the data. If the data has been accessed recently, then in the future The probability of being accessed is also higher;
LFU elimination rules based on the number of accesses, eliminate data based on the historical access frequency of the data. If the data has been accessed many times in the past, it will be accessed in the future. The frequency is also higher;
For example, if ten minutes is used as a node and page scheduling is performed every minute, when the required page direction is 2 1 2 4 2 3 4, And when adjusting page 4, a page fault interrupt will occur; if the LRU algorithm is used, page 1 should be changed (page 1 has not been used for the longest time within ten minutes), but if the LFU algorithm is used, page 3 should be changed (page 3 is only used within ten minutes). Used once).
2. In-depth understanding of the LRU algorithm
① View the characteristics of the LRU cache
The cache implemented using the LRU strategy is sorted according to the order of use. Each time an entry is accessed, The LRU algorithm will move it to the top of the cache. In this way, the algorithm can quickly identify the entries that have not been used for the longest time by looking at the bottom of the list.
As shown below, the user requests the LRU policy storage record for the first article from the network:
How the article is cached before it is served to the user Store it in the nearest slot? As shown below, what happens when the user requests the second article, the second article is stored in the top position, that is, the second article takes the most recent position, pushing the first article down the list:
The LRU policy assumes that the newer the object being used, the more likely it is to be used in the future, so it tries to keep the object in the cache for the longest time, i.e. if If an entry is eliminated, the cache storage record of the first document will be eliminated first.
② View the structure of the LRU cache
One way to implement LRU cache in Python is to use a doubly linked list (doubly linked list) and a hash map (hash map), the head of the doubly linked list The element will point to the most recently used entry, and its tail will point to the least recently used entry. The logical structure of the LRU cache implementation is as follows:
By using a hash map, each entry can be mapped to a specific location in the doubly linked list, thereby ensuring that each entry in the cache is item access. This strategy is very fast, accessing the least recently used item and updating the cache are both O(1) operations.
Starting from Python 3.2, Python has added the @lru_cache decorator to implement the LRU strategy. From now on, you can use this decorator to decorate functions and cache their calculation results.
3. Use lru_cache decorator
① Implementation principle of @lru_cache decorator
There are many ways to achieve fast response of the application, and using cache is a very Common methods. Caching, if used correctly, can make responses faster and reduce the extra load on computing resources.
The functools module in Python comes with the @lru_cache decorator for caching, which can use the least recently used (LRU) strategy to cache the calculation results of the function. This is a simple but powerful technology:
Implement @lru_cache decorator;
Understand how the LRU strategy works;
Use @ lru_cache decorator to improve performance;
Extends the functionality of the @lru_cache decorator and makes it expire after a specific time.
Just like the previously implemented caching scheme, the @lru_cache decorator storage in Python also uses a dictionary as the storage object, which will The execution result of the function is cached in the dictionary key, which consists of the call to the function (including the parameters of the function), which means that the parameters of these functions must be hashable for the decorator to work properly.
② Fibonacci Sequence
We should all know how to calculate the Fibonacci Sequence. A common solution is to use recursion:
-
0, 1, 1, 2, 3, 5, 8, 13, 21, 34 ……;
- ##2 is the sum of the previous two items ->( 1 1);
- 3 is the sum of the previous two items -> (1 2);
- 5 is the sum of the previous two items ->(2 3).
递归的计算简洁并且直观,但是由于存在大量重复计算,实际运行效率很低,并且会占用较多的内存。但是这里并不是需要关注的重点,只是来作为演示示例而已:
# 匿名函数 fib = lambda n: 1 if n <= 1 else fib(n-1) + fib(n-2) # 将时间复杂度降低到线性 fib = lambda n, a=1, b=1: a if n == 0 else fib(n-1, b, a+b) # 保证了匿名函数的匿名性 fib = lambda n, fib: 1 if n <= 1 else fib(n-1, fib) + fib(n-2, fib)
③ 使用 @lru_cache 缓存输出结果
使用 @lru_cache 装饰器来缓存的话,可以将函数调用结果存储在内存中,以便再次请求时直接返回结果:
from functools import lru_cache @lru_cache def fib(n): if n==1 or n==2: return 1 else: return fib(n-1) + fib(n-2)
④ 限制 @lru_cache 装饰器大小
Python 的 @lru_cache 装饰器提供了一个 maxsize 属性,该属性定义了在缓存开始淘汰旧条目之前的最大条目数,默认情况下,maxsize 设置为 128。
如果将 maxsize 设置为 None 的话,则缓存将无限期增长,并且不会驱逐任何条目。
from functools import lru_cache @lru_cache(maxsize=16) def fib(n): if n==1 or n==2: return 1 else: return fib(n-1) + fib(n-2)
# 查看缓存列表 >>> print(steps_to.cache_info()) CacheInfo(hits=52, misses=30, maxsize=16, currsize=16)
⑤ 使用 @lru_cache 实现 LRU 缓存
就像在前面实现的缓存解决方案一样,@lru_cache 在底层使用一个字典,它将函数的结果缓存在一个键下,该键包含对函数的调用,包括提供的参数。这意味着这些参数必须是可哈希的,才能让 decorator 工作。
示例:玩楼梯:
想象一下,你想通过一次跳上一个、两个或三个楼梯来确定到达楼梯中的一个特定楼梯的所有不同方式,到第四个楼梯有多少条路?所有不同的组合如下所示:
可以这样描述,为了到达当前的楼梯,你可以从下面的一个、两个或三个楼梯跳下去,将能够到达这些点的跳跃组合的数量相加,便能够获得到达当前位置的所有可能方法。
例如到达第四个楼梯的组合数量将等于你到达第三、第二和第一个楼梯的不同方式的总数。如下所示,有七种不同的方法可以到达第四层楼梯:
注意给定阶梯的解是如何建立在较小子问题的答案之上的,在这种情况下,为了确定到达第四个楼梯的不同路径,可以将到达第三个楼梯的四种路径、到达第二个楼梯的两种路径以及到达第一个楼梯的一种路径相加。 这种方法称为递归,下面是一个实现这个递归的函数:
def steps_to(stair): if stair == 1: # You can reach the first stair with only a single step # from the floor. return 1 elif stair == 2: # You can reach the second stair by jumping from the # floor with a single two-stair hop or by jumping a single # stair a couple of times. return 2 elif stair == 3: # You can reach the third stair using four possible # combinations: # 1. Jumping all the way from the floor # 2. Jumping two stairs, then one # 3. Jumping one stair, then two # 4. Jumping one stair three times return 4 else: # You can reach your current stair from three different places: # 1. From three stairs down # 2. From two stairs down # 2. From one stair down # # If you add up the number of ways of getting to those # those three positions, then you should have your solution. return ( steps_to(stair - 3) + steps_to(stair - 2) + steps_to(stair - 1) ) print(steps_to(4))
将此代码保存到一个名为 stairs.py 的文件中,并使用以下命令运行它:
$ python stairs.py 7
太棒了,这个代码适用于 4 个楼梯,但是数一下要走多少步才能到达楼梯上更高的地方呢?将第 33 行中的楼梯数更改为 30,并重新运行脚本:
$ python stairs.py 53798080
可以看到结果超过 5300 万个组合,这可真的有点多。
时间代码:
当找到第 30 个楼梯的解决方案时,脚本花了相当多的时间来完成。要获得基线,可以度量代码运行的时间,要做到这一点,可以使用 Python 的 timeit module,在第 33 行之后添加以下代码:
setup_code = "from __main__ import steps_to" 36stmt = "steps_to(30)" 37times = repeat(setup=setup_code, stmt=stmt, repeat=3, number=10) 38print(f"Minimum execution time: {min(times)}")
还需要在代码的顶部导入 timeit module:
from timeit import repeat
以下是对这些新增内容的逐行解释:
第 35 行导入 steps_to() 的名称,以便 time.com .repeat() 知道如何调用它;
第 36 行用希望到达的楼梯数(在本例中为 30)准备对函数的调用,这是将要执行和计时的语句;
第 37 行使用设置代码和语句调用 time.repeat(),这将调用该函数 10 次,返回每次执行所需的秒数;
第 38 行标识并打印返回的最短时间。 现在再次运行脚本:
$ python stairs.py 53798080 Minimum execution time: 40.014977024000004
可以看到的秒数取决于特定硬件,在我的系统上,脚本花了 40 秒,这对于 30 级楼梯来说是相当慢的。
使用记忆来改进解决方案:
这种递归实现通过将其分解为相互构建的更小的步骤来解决这个问题,如下所示是一个树,其中每个节点表示对 steps_to() 的特定调用:
注意需要如何使用相同的参数多次调用 steps_to(),例如 steps_to(5) 计算两次,steps_to(4) 计算四次,steps_to(3) 计算七次,steps_to(2) 计算六次,多次调用同一个函数会增加不必要的计算周期,结果总是相同的。
为了解决这个问题,可以使用一种叫做记忆的技术,这种方法将函数的结果存储在内存中,然后在需要时引用它,从而确保函数不会为相同的输入运行多次,这个场景听起来像是使用 Python 的 @lru_cache 装饰器的绝佳机会。
只要做两个改变,就可以大大提高算法的运行时间:
从 functools module 导入 @lru_cache 装饰器;
使用 @lru_cache 装饰 steps_to()。
下面是两个更新后的脚本顶部的样子:
from functools import lru_cache from timeit import repeat @lru_cache def steps_to(stair): if stair == 1:
运行更新后的脚本产生如下结果:
$ python stairs.py 53798080 Minimum execution time: 7.999999999987184e-07
缓存函数的结果会将运行时从 40 秒降低到 0.0008 毫秒,这是一个了不起的进步。@lru_cache 装饰器存储了每个不同输入的 steps_to() 的结果,每次代码调用带有相同参数的函数时,它都直接从内存中返回正确的结果,而不是重新计算一遍答案,这解释了使用 @lru_cache 时性能的巨大提升。
⑥ 解包 @lru_cache 的功能
有了@lru_cache 装饰器,就可以将每个调用和应答存储在内存中,以便以后再次请求时进行访问,但是在内存耗尽之前,可以节省多少次调用呢?
Python 的 @lru_cache 装饰器提供了一个 maxsize 属性,它定义了在缓存开始清除旧条目之前的最大条目数,缺省情况下,maxsize 设置为 128,如果将 maxsize 设置为 None,那么缓存将无限增长,并且不会驱逐任何条目。如果在内存中存储大量不同的调用,这可能会成为一个问题。
如下是 @lru_cache 使用 maxsize 属性:
from functools import lru_cache from timeit import repeat @lru_cache(maxsize=16) def steps_to(stair): if stair == 1:
在本例中,将缓存限制为最多 16 个条目,当一个新调用传入时,decorator 的实现将会从现有的 16 个条目中删除最近最少使用的条目,为新条目腾出位置。
要查看添加到代码中的新内容会发生什么,可以使用 @lru_cache 装饰器提供的 cache_info() 来检查命中和未命中的次数以及当前缓存的大小。为了清晰起见,删除乘以函数运行时的代码,以下是修改后的最终脚本:
from functools import lru_cache from timeit import repeat @lru_cache(maxsize=16) def steps_to(stair): if stair == 1: # You can reach the first stair with only a single step # from the floor. return 1 elif stair == 2: # You can reach the second stair by jumping from the # floor with a single two-stair hop or by jumping a single # stair a couple of times. return 2 elif stair == 3: # You can reach the third stair using four possible # combinations: # 1. Jumping all the way from the floor # 2. Jumping two stairs, then one # 3. Jumping one stair, then two # 4. Jumping one stair three times return 4 else: # You can reach your current stair from three different places: # 1. From three stairs down # 2. From two stairs down # 2. From one stair down # # If you add up the number of ways of getting to those # those three positions, then you should have your solution. return ( steps_to(stair - 3) + steps_to(stair - 2) + steps_to(stair - 1) ) print(steps_to(30)) print(steps_to.cache_info())
如果再次调用脚本,可以看到如下结果:
$ python stairs.py 53798080 CacheInfo(hits=52, misses=30, maxsize=16, currsize=16)
可以使用 cache_info() 返回的信息来了解缓存是如何执行的,并对其进行微调,以找到速度和存储之间的适当平衡。下面是 cache_info() 提供的属性的详细说明:
hits=52 是 @lru_cache 直接从内存中返回的调用数,因为它们存在于缓存中;
misses =30 是被计算的不是来自内存的调用数,因为试图找到到达第 30 级楼梯的台阶数,所以每次调用都在第一次调用时错过了缓存是有道理的;
maxsize =16 是用装饰器的 maxsize 属性定义的缓存的大小;
currsize =16 是当前缓存的大小,在本例中它表明缓存已满。
如果需要从缓存中删除所有条目,那么可以使用 @lru_cache 提供的 cache_clear()。
四、添加缓存过期
假设想要开发一个脚本来监视 Real Python 并在任何包含单词 Python 的文章中打印字符数。真正的 Python 提供了一个 Atom feed,因此可以使用 feedparser 库来解析提要,并使用请求库来加载本文的内容。
如下是监控脚本的实现:
import feedparser import requests import ssl import time if hasattr(ssl, "_create_unverified_context"): ssl._create_default_https_context = ssl._create_unverified_context def get_article_from_server(url): print("Fetching article from server...") response = requests.get(url) return response.text def monitor(url): maxlen = 45 while True: print("\nChecking feed...") feed = feedparser.parse(url) for entry in feed.entries[:5]: if "python" in entry.title.lower(): truncated_title = ( entry.title[:maxlen] + "..." if len(entry.title) > maxlen else entry.title ) print( "Match found:", truncated_title, len(get_article_from_server(entry.link)), ) time.sleep(5) monitor("https://realpython.com/atom.xml")
将此脚本保存到一个名为 monitor.py 的文件中,安装 feedparser 和请求库,然后运行该脚本,它将持续运行,直到在终端窗口中按 Ctrl+C 停止它:
$ pip install feedparser requests $ python monitor.py Checking feed... Fetching article from server... The Real Python Podcast – Episode #28: Using ... 29520 Fetching article from server... Python Community Interview With David Amos 54256 Fetching article from server... Working With Linked Lists in Python 37099 Fetching article from server... Python Practice Problems: Get Ready for Your ... 164888 Fetching article from server... The Real Python Podcast – Episode #27: Prepar... 30784 Checking feed... Fetching article from server... The Real Python Podcast – Episode #28: Using ... 29520 Fetching article from server... Python Community Interview With David Amos 54256 Fetching article from server... Working With Linked Lists in Python 37099 Fetching article from server... Python Practice Problems: Get Ready for Your ... 164888 Fetching article from server... The Real Python Podcast – Episode #27: Prepar... 30784
代码解释:
第 6 行和第 7 行:当 feedparser 试图访问通过 HTTPS 提供的内容时,这是一个解决方案;
第 16 行:monitor() 将无限循环;
第 18 行:使用 feedparser,代码从真正的 Python 加载并解析提要;
第 20 行:循环遍历列表中的前 5 个条目;
第 21 到 31 行:如果单词 python 是标题的一部分,那么代码将连同文章的长度一起打印它;
第 33 行:代码在继续之前休眠了 5 秒钟;
第 35 行:这一行通过将 Real Python 提要的 URL 传递给 monitor() 来启动监视过程。
每当脚本加载一篇文章时,“Fetching article from server…”的消息就会打印到控制台,如果让脚本运行足够长的时间,那么将看到这条消息是如何反复显示的,即使在加载相同的链接时也是如此。
这是一个很好的机会来缓存文章的内容,并避免每五秒钟访问一次网络,可以使用 @lru_cache 装饰器,但是如果文章的内容被更新,会发生什么呢?第一次访问文章时,装饰器将存储文章的内容,并在以后每次返回相同的数据;如果更新了帖子,那么监视器脚本将永远无法实现它,因为它将提取存储在缓存中的旧副本。要解决这个问题,可以将缓存条目设置为过期。
from functools import lru_cache, wraps from datetime import datetime, timedelta def timed_lru_cache(seconds: int, maxsize: int = 128): def wrapper_cache(func): func = lru_cache(maxsize=maxsize)(func) func.lifetime = timedelta(seconds=seconds) func.expiration = datetime.utcnow() + func.lifetime @wraps(func) def wrapped_func(*args, **kwargs): if datetime.utcnow() >= func.expiration: func.cache_clear() func.expiration = datetime.utcnow() + func.lifetime return func(*args, **kwargs) return wrapped_func return wrapper_cache @timed_lru_cache(10) def get_article_from_server(url): ...
代码解释:
第 4 行:@timed_lru_cache 装饰器将支持缓存中条目的生命周期(以秒为单位)和缓存的最大大小;
第 6 行:代码用 lru_cache 装饰器包装了装饰函数,这允许使用 lru_cache 已经提供的缓存功能;
第 7 行和第 8 行:这两行用两个表示缓存生命周期和它将过期的实际日期的属性来修饰函数;
第 12 到 14 行:在访问缓存中的条目之前,装饰器检查当前日期是否超过了过期日期,如果是这种情况,那么它将清除缓存并重新计算生存期和过期日期。
请注意,当条目过期时,此装饰器如何清除与该函数关联的整个缓存,生存期适用于整个缓存,而不适用于单个项目,此策略的更复杂实现将根据条目的单个生存期将其逐出。
在程序中,如果想要实现不同缓存策略,可以查看 cachetools 这个库,该库提供了几个集合和修饰符,涵盖了一些最流行的缓存策略。
使用新装饰器缓存文章:
现在可以将新的 @timed_lru_cache 装饰器与监视器脚本一起使用,以防止每次访问时获取文章的内容。为了简单起见,把代码放在一个脚本中,可以得到以下结果:
import feedparser import requests import ssl import time from functools import lru_cache, wraps from datetime import datetime, timedelta if hasattr(ssl, "_create_unverified_context"): ssl._create_default_https_context = ssl._create_unverified_context def timed_lru_cache(seconds: int, maxsize: int = 128): def wrapper_cache(func): func = lru_cache(maxsize=maxsize)(func) func.lifetime = timedelta(seconds=seconds) func.expiration = datetime.utcnow() + func.lifetime @wraps(func) def wrapped_func(*args, **kwargs): if datetime.utcnow() >= func.expiration: func.cache_clear() func.expiration = datetime.utcnow() + func.lifetime return func(*args, **kwargs) return wrapped_func return wrapper_cache @timed_lru_cache(10) def get_article_from_server(url): print("Fetching article from server...") response = requests.get(url) return response.text def monitor(url): maxlen = 45 while True: print("\nChecking feed...") feed = feedparser.parse(url) for entry in feed.entries[:5]: if "python" in entry.title.lower(): truncated_title = ( entry.title[:maxlen] + "..." if len(entry.title) > maxlen else entry.title ) print( "Match found:", truncated_title, len(get_article_from_server(entry.link)), ) time.sleep(5) monitor("https://realpython.com/atom.xml")
请注意第 30 行如何使用 @timed_lru_cache 装饰 get_article_from_server() 并指定 10 秒的有效性。在获取文章后的 10 秒内,任何试图从服务器访问同一篇文章的尝试都将从缓存中返回内容,而不会到达网络。
运行脚本并查看结果:
$ python monitor.py Checking feed... Fetching article from server... Match found: The Real Python Podcast – Episode #28: Using ... 29521 Fetching article from server... Match found: Python Community Interview With David Amos 54254 Fetching article from server... Match found: Working With Linked Lists in Python 37100 Fetching article from server... Match found: Python Practice Problems: Get Ready for Your ... 164887 Fetching article from server... Match found: The Real Python Podcast – Episode #27: Prepar... 30783 Checking feed... Match found: The Real Python Podcast – Episode #28: Using ... 29521 Match found: Python Community Interview With David Amos 54254 Match found: Working With Linked Lists in Python 37100 Match found: Python Practice Problems: Get Ready for Your ... 164887 Match found: The Real Python Podcast – Episode #27: Prepar... 30783 Checking feed... Match found: The Real Python Podcast – Episode #28: Using ... 29521 Match found: Python Community Interview With David Amos 54254 Match found: Working With Linked Lists in Python 37100 Match found: Python Practice Problems: Get Ready for Your ... 164887 Match found: The Real Python Podcast – Episode #27: Prepar... 30783 Checking feed... Fetching article from server... Match found: The Real Python Podcast – Episode #28: Using ... 29521 Fetching article from server... Match found: Python Community Interview With David Amos 54254 Fetching article from server... Match found: Working With Linked Lists in Python 37099 Fetching article from server... Match found: Python Practice Problems: Get Ready for Your ... 164888 Fetching article from server... Match found: The Real Python Podcast – Episode #27: Prepar... 30783
请注意,代码在第一次访问匹配的文章时是如何打印“Fetching article from server…”这条消息的。之后,根据网络速度和计算能力,脚本将从缓存中检索文章一两次,然后再次访问服务器。
该脚本试图每 5 秒访问这些文章,缓存每 10 秒过期一次。对于实际的应用程序来说,这些时间可能太短,因此可以通过调整这些配置来获得显著的改进。
五、@lru_cache 装饰器的官方实现
简单理解,其实就是一个装饰器:
def lru_cache(maxsize=128, typed=False): if isinstance(maxsize, int): if maxsize < 0: maxsize = 0 elif callable(maxsize) and isinstance(typed, bool): user_function, maxsize = maxsize, 128 wrapper = _lru_cache_wrapper(user_function, maxsize, typed, _CacheInfo) return update_wrapper(wrapper, user_function) elif maxsize is not None: raise TypeError('Expected first argument to be an integer, a callable, or None') def decorating_function(user_function): wrapper = _lru_cache_wrapper(user_function, maxsize, typed, _CacheInfo) return update_wrapper(wrapper, user_function) return decorating_function
_CacheInfo = namedtuple("CacheInfo", ["hits", "misses", "maxsize", "currsize"]) def _lru_cache_wrapper(user_function, maxsize, typed, _CacheInfo): sentinel = object() # unique object used to signal cache misses make_key = _make_key # build a key from the function arguments PREV, NEXT, KEY, RESULT = 0, 1, 2, 3 # names for the link fields cache = {} # 存储也使用的字典 hits = misses = 0 full = False cache_get = cache.get cache_len = cache.__len__ lock = RLock() # 因为双向链表的更新不是线程安全的所以需要加锁 root = [] # 双向链表 root[:] = [root, root, None, None] # 初始化双向链表 if maxsize == 0: def wrapper(*args, **kwds): # No caching -- just a statistics update nonlocal misses misses += 1 result = user_function(*args, **kwds) return result elif maxsize is None: def wrapper(*args, **kwds): # Simple caching without ordering or size limit nonlocal hits, misses key = make_key(args, kwds, typed) result = cache_get(key, sentinel) if result is not sentinel: hits += 1 return result misses += 1 result = user_function(*args, **kwds) cache[key] = result return result else: def wrapper(*args, **kwds): # Size limited caching that tracks accesses by recency nonlocal root, hits, misses, full key = make_key(args, kwds, typed) with lock: link = cache_get(key) if link is not None: # Move the link to the front of the circular queue link_prev, link_next, _key, result = link link_prev[NEXT] = link_next link_next[PREV] = link_prev last = root[PREV] last[NEXT] = root[PREV] = link link[PREV] = last link[NEXT] = root hits += 1 return result misses += 1 result = user_function(*args, **kwds) with lock: if key in cache: pass elif full: oldroot = root oldroot[KEY] = key oldroot[RESULT] = result root = oldroot[NEXT] oldkey = root[KEY] oldresult = root[RESULT] root[KEY] = root[RESULT] = None del cache[oldkey] cache[key] = oldroot else: last = root[PREV] link = [last, root, key, result] last[NEXT] = root[PREV] = cache[key] = link full = (cache_len() >= maxsize) return result def cache_info(): """Report cache statistics""" with lock: return _CacheInfo(hits, misses, maxsize, cache_len()) def cache_clear(): """Clear the cache and cache statistics""" nonlocal hits, misses, full with lock: cache.clear() root[:] = [root, root, None, None] hits = misses = 0 full = False wrapper.cache_info = cache_info wrapper.cache_clear = cache_clear return wrapper
The above is the detailed content of How Python uses LRU cache strategy for caching. For more information, please follow other related articles on the PHP Chinese website!
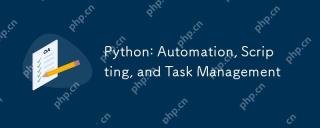
Python excels in automation, scripting, and task management. 1) Automation: File backup is realized through standard libraries such as os and shutil. 2) Script writing: Use the psutil library to monitor system resources. 3) Task management: Use the schedule library to schedule tasks. Python's ease of use and rich library support makes it the preferred tool in these areas.
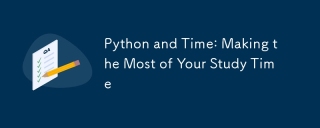
To maximize the efficiency of learning Python in a limited time, you can use Python's datetime, time, and schedule modules. 1. The datetime module is used to record and plan learning time. 2. The time module helps to set study and rest time. 3. The schedule module automatically arranges weekly learning tasks.
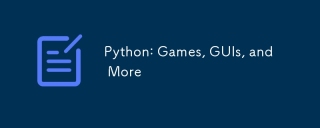
Python excels in gaming and GUI development. 1) Game development uses Pygame, providing drawing, audio and other functions, which are suitable for creating 2D games. 2) GUI development can choose Tkinter or PyQt. Tkinter is simple and easy to use, PyQt has rich functions and is suitable for professional development.
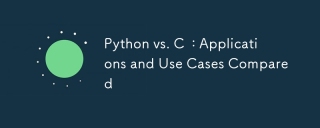
Python is suitable for data science, web development and automation tasks, while C is suitable for system programming, game development and embedded systems. Python is known for its simplicity and powerful ecosystem, while C is known for its high performance and underlying control capabilities.
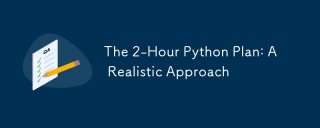
You can learn basic programming concepts and skills of Python within 2 hours. 1. Learn variables and data types, 2. Master control flow (conditional statements and loops), 3. Understand the definition and use of functions, 4. Quickly get started with Python programming through simple examples and code snippets.
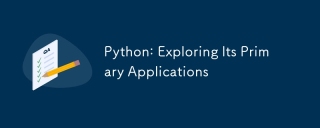
Python is widely used in the fields of web development, data science, machine learning, automation and scripting. 1) In web development, Django and Flask frameworks simplify the development process. 2) In the fields of data science and machine learning, NumPy, Pandas, Scikit-learn and TensorFlow libraries provide strong support. 3) In terms of automation and scripting, Python is suitable for tasks such as automated testing and system management.
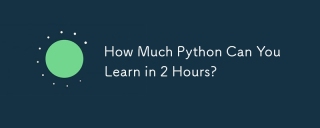
You can learn the basics of Python within two hours. 1. Learn variables and data types, 2. Master control structures such as if statements and loops, 3. Understand the definition and use of functions. These will help you start writing simple Python programs.
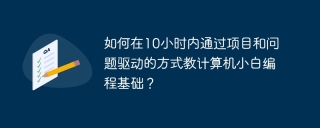
How to teach computer novice programming basics within 10 hours? If you only have 10 hours to teach computer novice some programming knowledge, what would you choose to teach...


Hot AI Tools

Undresser.AI Undress
AI-powered app for creating realistic nude photos

AI Clothes Remover
Online AI tool for removing clothes from photos.

Undress AI Tool
Undress images for free

Clothoff.io
AI clothes remover

AI Hentai Generator
Generate AI Hentai for free.

Hot Article

Hot Tools

Atom editor mac version download
The most popular open source editor

MinGW - Minimalist GNU for Windows
This project is in the process of being migrated to osdn.net/projects/mingw, you can continue to follow us there. MinGW: A native Windows port of the GNU Compiler Collection (GCC), freely distributable import libraries and header files for building native Windows applications; includes extensions to the MSVC runtime to support C99 functionality. All MinGW software can run on 64-bit Windows platforms.
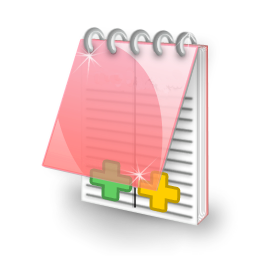
EditPlus Chinese cracked version
Small size, syntax highlighting, does not support code prompt function
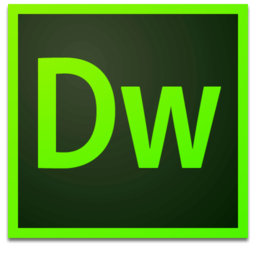
Dreamweaver Mac version
Visual web development tools

Notepad++7.3.1
Easy-to-use and free code editor