


Complete code demonstration of document parsing using Python and OCR (code attached)
Document parsing involves examining the data in the document and extracting useful information. It can reduce a lot of manual work through automation. A popular parsing strategy is to convert documents into images and use computer vision for recognition. While Document Image Analysis refers to the technology of obtaining information from the pixel data of an image of a document, in some cases there is no clear answer to what the expected results should be (text, images, charts, numbers, Tables, formulas...).
OCR (Optical Character Recognition) is the process of detecting and extracting text in images through computer vision. It was invented during World War I, when Israeli scientist Emanuel Goldberg created a machine that could read characters and convert them into telegraph codes. By now the field has reached a very sophisticated level, mixing image processing, text localization, character segmentation and character recognition. Basically an object detection technique for text.
In this article I will show how to use OCR for document parsing. I'll show some useful Python code that can be easily used in other similar situations (just copy, paste, run) and provide a full source code download.
Here we will take the financial statements in PDF format of a listed company as an example (link below).
https://s2.q4cdn.com/470004039/files/doc_financials/2021/q4/_10-K-2021-(As-Filed).pdf
Detect and extract text, graphics, and tables from this PDF
Environment settings
The annoying part of document parsing is , there are so many tools for different types of data (text, graphics, tables) but none of them work perfectly. Here are some of the most popular methods and packages:
- Process documents as text: use PyPDF2 to extract text, Camelot or TabulaPy to extract tables, and PyMuPDF to extract graphics.
- Convert documents to images (OCR): use pdf2image for conversion, PyTesseract and many other libraries to extract data, or just use LayoutParser.
Maybe you will ask: "Why not process the PDF file directly, but convert the pages into images?" You can do this. The main disadvantage of this strategy is the encoding issue: documents can be in multiple encodings (i.e. UTF-8, ASCII, Unicode), so conversion to text may result in data loss. So in order to avoid this problem, I will use OCR and convert the page to an image with pdf2image. Note that the PDF rendering library Poppler is required.
# with pip pip install python-poppler # with conda conda install -c conda-forge poppler
You can read the file easily:
# READ AS IMAGE import pdf2imagedoc = pdf2image.convert_from_path("doc_apple.pdf") len(doc) #<-- check num pages doc[0] #<-- visualize a page
It is exactly the same as our screenshot. If you want to save the page image locally, you can use the following code:
# Save imgs import osfolder = "doc" if folder not in os.listdir(): os.makedirs(folder)p = 1 for page in doc: image_name = "page_"+str(p)+".jpg" page.save(os.path.join(folder, image_name), "JPEG") p = p+1
Finally, we need to set up the CV engine we will use. LayoutParser appears to be the first general-purpose package for OCR based on deep learning. It uses two well-known models to complete the task:
Detection: Facebook's most advanced object detection library (the second version Detectron2 will be used here).
pip install layoutparser torchvision && pip install "git+https://github.com/facebookresearch/detectron2.git@v0.5#egg=detectron2"
Tesseract: The most famous OCR system was created by HP in 1985 and is currently developed by Google.
pip install "layoutparser[ocr]"
Now you are ready to start the OCR program for information detection and extraction.
import layoutparser as lp import cv2 import numpy as np import io import pandas as pd import matplotlib.pyplot as plt
Detection
(Target) Detection is the process of finding pieces of information in an image and then surrounding them with a rectangular border. For document parsing, the information is titles, text, graphics, tables...
Let's look at a complex page that contains a few things:
This page starts with a title, has a text block, then a figure and a table, so we need a trained model to recognize these objects. Luckily Detectron is able to do this, we just need to select a model from here and specify its path in code.
#The model I am going to use can only detect 4 objects (text, title, list, table, graph). Therefore, if you need to identify other things (like equations), you have to use other models.
## load pre-trained model model = lp.Detectron2LayoutModel( "lp://PubLayNet/mask_rcnn_X_101_32x8d_FPN_3x/config", extra_config=["MODEL.ROI_HEADS.SCORE_THRESH_TEST", 0.8], label_map={0:"Text", 1:"Title", 2:"List", 3:"Table", 4:"Figure"}) ## turn img into array i = 21 img = np.asarray(doc[i]) ## predict detected = model.detect(img) ## plot lp.draw_box(img, detected, box_width=5, box_alpha=0.2, show_element_type=True)
结果包含每个检测到的布局的细节,例如边界框的坐标。根据页面上显示的顺序对输出进行排序是很有用的:
## sort new_detected = detected.sort(key=lambda x: x.coordinates[1]) ## assign ids detected = lp.Layout([block.set(id=idx) for idx,block in enumerate(new_detected)])## check for block in detected: print("---", str(block.id)+":", block.type, "---") print(block, end='nn')
完成OCR的下一步是正确提取检测到内容中的有用信息。
提取
我们已经对图像完成了分割,然后就需要使用另外一个模型处理分段的图像,并将提取的输出保存到字典中。
由于有不同类型的输出(文本,标题,图形,表格),所以这里准备了一个函数用来显示结果。
''' {'0-Title': '...', '1-Text': '...', '2-Figure': array([[ [0,0,0], ...]]), '3-Table': pd.DataFrame, } ''' def parse_doc(dic): for k,v in dic.items(): if "Title" in k: print('x1b[1;31m'+ v +'x1b[0m') elif "Figure" in k: plt.figure(figsize=(10,5)) plt.imshow(v) plt.show() else: print(v) print(" ")
首先看看文字:
# load model model = lp.TesseractAgent(languages='eng') dic_predicted = {} for block in [block for block in detected if block.type in ["Title","Text"]]: ## segmentation segmented = block.pad(left=15, right=15, top=5, bottom=5).crop_image(img) ## extraction extracted = model.detect(segmented) ## save dic_predicted[str(block.id)+"-"+block.type] = extracted.replace('n',' ').strip() # check parse_doc(dic_predicted)
再看看图形报表
for block in [block for block in detected if block.type == "Figure"]: ## segmentation segmented = block.pad(left=15, right=15, top=5, bottom=5).crop_image(img) ## save dic_predicted[str(block.id)+"-"+block.type] = segmented # check parse_doc(dic_predicted)
上面两个看着很不错,那是因为这两种类型相对简单,但是表格就要复杂得多。尤其是我们上看看到的的这个,因为它的行和列都是进行了合并后产生的。
for block in [block for block in detected if block.type == "Table"]: ## segmentation segmented = block.pad(left=15, right=15, top=5, bottom=5).crop_image(img) ## extraction extracted = model.detect(segmented) ## save dic_predicted[str(block.id)+"-"+block.type] = pd.read_csv( io.StringIO(extracted) ) # check parse_doc(dic_predicted)
正如我们的预料提取的表格不是很好。好在Python有专门处理表格的包,我们可以直接处理而不将其转换为图像。这里使用TabulaPy 包:
import tabula tables = tabula.read_pdf("doc_apple.pdf", pages=i+1) tables[0]
结果要好一些,但是名称仍然错了,但是效果要比直接OCR好的多。
总结
本文是一个简单教程,演示了如何使用OCR进行文档解析。使用Layoutpars软件包进行了整个检测和提取过程。并展示了如何处理PDF文档中的文本,数字和表格。
The above is the detailed content of Complete code demonstration of document parsing using Python and OCR (code attached). For more information, please follow other related articles on the PHP Chinese website!
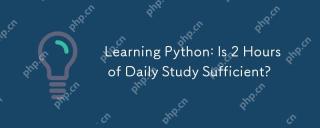
Is it enough to learn Python for two hours a day? It depends on your goals and learning methods. 1) Develop a clear learning plan, 2) Select appropriate learning resources and methods, 3) Practice and review and consolidate hands-on practice and review and consolidate, and you can gradually master the basic knowledge and advanced functions of Python during this period.
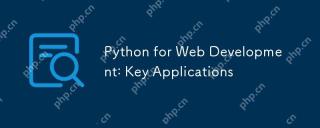
Key applications of Python in web development include the use of Django and Flask frameworks, API development, data analysis and visualization, machine learning and AI, and performance optimization. 1. Django and Flask framework: Django is suitable for rapid development of complex applications, and Flask is suitable for small or highly customized projects. 2. API development: Use Flask or DjangoRESTFramework to build RESTfulAPI. 3. Data analysis and visualization: Use Python to process data and display it through the web interface. 4. Machine Learning and AI: Python is used to build intelligent web applications. 5. Performance optimization: optimized through asynchronous programming, caching and code
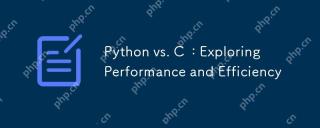
Python is better than C in development efficiency, but C is higher in execution performance. 1. Python's concise syntax and rich libraries improve development efficiency. 2.C's compilation-type characteristics and hardware control improve execution performance. When making a choice, you need to weigh the development speed and execution efficiency based on project needs.
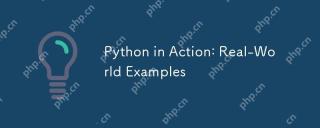
Python's real-world applications include data analytics, web development, artificial intelligence and automation. 1) In data analysis, Python uses Pandas and Matplotlib to process and visualize data. 2) In web development, Django and Flask frameworks simplify the creation of web applications. 3) In the field of artificial intelligence, TensorFlow and PyTorch are used to build and train models. 4) In terms of automation, Python scripts can be used for tasks such as copying files.
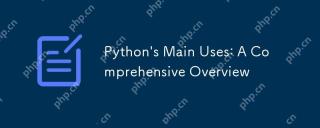
Python is widely used in data science, web development and automation scripting fields. 1) In data science, Python simplifies data processing and analysis through libraries such as NumPy and Pandas. 2) In web development, the Django and Flask frameworks enable developers to quickly build applications. 3) In automated scripts, Python's simplicity and standard library make it ideal.
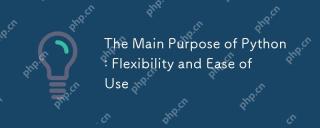
Python's flexibility is reflected in multi-paradigm support and dynamic type systems, while ease of use comes from a simple syntax and rich standard library. 1. Flexibility: Supports object-oriented, functional and procedural programming, and dynamic type systems improve development efficiency. 2. Ease of use: The grammar is close to natural language, the standard library covers a wide range of functions, and simplifies the development process.
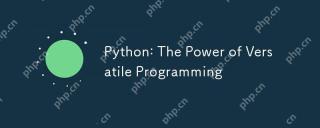
Python is highly favored for its simplicity and power, suitable for all needs from beginners to advanced developers. Its versatility is reflected in: 1) Easy to learn and use, simple syntax; 2) Rich libraries and frameworks, such as NumPy, Pandas, etc.; 3) Cross-platform support, which can be run on a variety of operating systems; 4) Suitable for scripting and automation tasks to improve work efficiency.
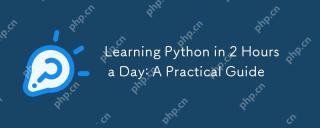
Yes, learn Python in two hours a day. 1. Develop a reasonable study plan, 2. Select the right learning resources, 3. Consolidate the knowledge learned through practice. These steps can help you master Python in a short time.


Hot AI Tools

Undresser.AI Undress
AI-powered app for creating realistic nude photos

AI Clothes Remover
Online AI tool for removing clothes from photos.

Undress AI Tool
Undress images for free

Clothoff.io
AI clothes remover

AI Hentai Generator
Generate AI Hentai for free.

Hot Article

Hot Tools

Atom editor mac version download
The most popular open source editor

SecLists
SecLists is the ultimate security tester's companion. It is a collection of various types of lists that are frequently used during security assessments, all in one place. SecLists helps make security testing more efficient and productive by conveniently providing all the lists a security tester might need. List types include usernames, passwords, URLs, fuzzing payloads, sensitive data patterns, web shells, and more. The tester can simply pull this repository onto a new test machine and he will have access to every type of list he needs.

PhpStorm Mac version
The latest (2018.2.1) professional PHP integrated development tool

SAP NetWeaver Server Adapter for Eclipse
Integrate Eclipse with SAP NetWeaver application server.
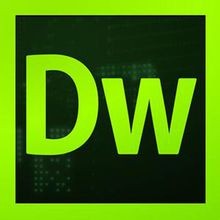
Dreamweaver CS6
Visual web development tools