


Has deep learning hit a wall after ten years? Hinton, LeCun, and Li Feifei don't think so.
It has been 10 years since the breakthrough of deep learning technology represented by AlexNet in 2012.
Ten years later, Geoffrey Hinton and Yann LeCun, who are now Turing Award winners, and the main initiator and promoter of the ImageNet Challenge, how does Li Feifei view the AI technology breakthroughs of the past decade? What is your judgment on the technological development in the next ten years?
Recently, an exclusive interview article by overseas media VentureBeat made the AI community begin to discuss these issues.
In LeCun’s view, the most important achievements in the past decade include self-supervised learning, ResNets, gate-attention-dynamic connection graphs, differentiable storage and Permutation equivariant modules, such as multi-head self-attention-Transformer.
Hinton believes that the rapid development momentum in the field of AI will continue to accelerate. Previously, he and other well-known figures in the AI field refuted the view that "deep learning has hit a wall." Hinton said, "We are seeing huge advances in robotics, with flexible, agile and more compliant robots doing things more efficiently and gently than humans."
GeoffreyHinton. Image source: https://www.thestar.com/
LeCun and Li Feifei agree with Hinton that a series of groundbreaking research based on the ImageNet data set in 2012 opened up computer vision, especially depth. Major advances in the field of learning have pushed deep learning into the mainstream and triggered an unstoppable momentum of development. Li Feifei said that the deep learning changes since 2012 were beyond her dreams.
李飞飞
However, success often leads to criticism. Recently, many opinions have pointed out the limitations of deep learning, believing that its success is limited to a small range. These views argue that deep learning cannot achieve the fundamental breakthrough it claims to ultimately help humans achieve their desired general artificial intelligence, in which AI’s reasoning capabilities are truly human-like.
Gary Marcus, a well-known AI scholar and founder of Robust.AI, published an article "Deep Learning Hits a Wall" in March this year. He believes that pure end-to-end deep learning has almost come to an end. , the entire AI field must find a new way out. Later, both Hinton and LeCun refuted his views, which triggered heated discussions in the circle.
#Although the voices of criticism continue, they cannot deny that great progress has been made in key applications such as computer vision and language. Thousands of businesses have also seen the power of deep learning and achieved remarkable results in recommendation engines, translation software, chatbots, and more.
It’s 2022, and when we look back on the past ten years of booming AI, what can we learn from the progress of deep learning? Will this transformative technology that changes the world be better or go downhill in the future? Hinton, LeCun, Li Feifei and others expressed their opinions on this.
In 2012, the deep learning revolution began
Hinton has always believed in the arrival of the deep learning revolution. In 1986, Hinton et al.'s paper "Learning representations by back-propagating errors" proposed a backpropagation algorithm for training multi-layer neural networks, and he firmly believed that this was the future of artificial intelligence. Later, LeCun, who pioneered the use of backpropagation and convolutional neural networks in 1989, agreed.
Hinton and LeCun and others believe that deep learning architectures such as multi-layer neural networks can be applied to areas such as computer vision, speech recognition, natural language processing and machine translation, and generate results that rival or even surpass human experts. result. At the same time, Li Feifei also put forward his firmly believed hypothesis that as long as the algorithm is correct, the ImageNet data set will become the key to advancing computer vision and deep learning research.
In 2012, the paper "ImageNet Classification with Deep Convolutional Neural Networks" by Alex Krizhevsky, Ilya Sutskever and Hinton came out, using the ImageNet data set to create the AlexNet neural network architecture that everyone is very familiar with today, and Won the ImageNet competition championship that year. This groundbreaking architecture at the time was far more accurate at classifying different images than previous methods.
Paper address: https://proceedings.neurips.cc/paper/2012/file/c399862d3b9d6b76c8436e924a68c45b-Paper.pdf
It can be said that this research is more powerful in the ImageNet data set and With the support of GPU hardware, it has directly contributed to the major AI success stories of the next decade, such as Google Photos, Google Translate, Amazon Alexa, OpenAI DALL-E and DeepMind AlphaFold.
In 2012, when AlexNet was launched, other people and institutions began to turn to the field of deep learning research. The Google
Meanwhile, Jeffrey Dean and Andrew Ng are also conducting groundbreaking work in the field of large-scale image recognition. Dan Ciregan et al.'s mid-submission CVPR 2012 paper significantly improves the state-of-the-art performance of convolutional neural networks on multiple image datasets.
Paper address: https://arxiv.org/pdf/1202.2745.pdf
In short, by 2013, " Almost all computer vision research turned to neural networks," said Hinton, who has since divided his time between Google Research and the University of Toronto. He added that since as recently as 2007, there has been almost a revolution in artificial intelligence, and back then, "it would not even have been appropriate to publish two papers on deep learning at one conference."
Ten Years of Progress in Deep Learning
Li Feifei said that she was deeply involved in the breakthrough of deep learning - personally announcing the victory of the ImageNet competition at the 2012 Florence Conference in Italy - It’s no surprise that people recognized the importance of that moment.
"ImageNet was a vision that started in 2006 with almost no support," Li said, adding that it later "actually paid off in such a historic and significant way."
Since 2012, deep learning has developed at an astonishing pace and with an impressive depth.
“There are some barriers that are being cleared at an incredible pace,” LeCun said, citing advances in natural language understanding, text generative translation and image synthesis.
In some areas progress is even faster than expected. For Hinton, that progress includes the use of neural networks in machine translation, which made huge strides in 2014. "I thought it would be many years," he said.
Li Feifei also admitted that advances in computer vision - such as DALL-E - "are faster than I thought."
Rejecting Deep Learning Critics
However, not everyone agrees that the progress of deep learning is jaw-dropping. In November 2012, Gary Marcus wrote an article for The New Yorker, saying: “To paraphrase the old fable, Hinton builds a better ladder, but a better ladder doesn’t necessarily get you up. To the moon."
Marcus believes that deep learning is no closer to the "moon" than it was ten years ago, where the moon refers to general artificial intelligence or human-level artificial intelligence.
"Of course there is progress, but in order to go to the moon, you have to solve for causal understanding and natural language understanding and reasoning," he said. “There hasn’t been much progress on these things.”
Marcus believes that hybrid models that combine neural networks with symbolic AI (the branch of AI that dominated the field before the rise of deep learning) are adversarial neural networks. The ultimate way forward. But Hinton and LeCun both dismissed Marcus' criticism.
“Deep learning hasn’t hit a wall — if you look at the recent progress, it’s been amazing,” Hinton said, although he has acknowledged that deep learning is limited in the range of problems it can solve. of.
LeCun added, “There’s no wall to hit.” "I think there are some hurdles that need to be cleared, and the solutions to those hurdles are not entirely clear," he said. "But I don't see progress slowing down at all... progress is accelerating."
However, Bender is not convinced. "To some extent, they're just talking about progress in classifying images based on labels provided by benchmarks like ImageNet, and it looks like there were some breakthroughs in 2012. But if they're talking about something bigger than that, that's not the case. It’s hype.”
AI bias and ethics issues are looming
In other respects, Bender also believes that the fields of artificial intelligence and deep learning have gone too far.
"I do think that the ability to process very large data sets into systems that can generate synthetic text and images (computationally efficient algorithms) has derailed us in several ways," she explain. For example, people seem to be stuck in a cycle: they find that the model is biased and propose to try to remove the bias, but the accepted result is that there is no completely debiased data set or model.
Additionally, she expressed a desire to see the field held to real standards of accountability, whether for real-world testing or product safety—“For that, we need the public at large to understand and how to see through the AI hype narratives that are at risk.” , we will need effective regulation."
However, LeCun noted that these are complex and important issues that people tend to simplify, and many people "have malicious assumptions." He insists that most companies "actually want to do the right thing."
In addition, he also complained about those who are not involved in artificial intelligence technology and research. "It's a whole ecosystem, but some people are shooting from the stands," he said, "basically just seeking attention."
The debate over deep learning will certainly continue
Although the debate seems heated, Li Feifei emphasized that these are all part of science. "Science is not truth, science is a journey to find truth. It is a journey of discovery and improvement - so debate, criticism, celebration are all part of it."
However, some debates and criticisms allow Li Feifei feels that it is "a bit contrived." Whether it is saying that AI is wrong or that AGI is coming, these are extreme cases. "I think this is a relatively popular version of a scientific debate that is deeper, more nuanced, more nuanced, and more dimensional."
Of course, Li Feifei pointed out that in the past decade, artificial intelligence has Progress has been disappointing – and it’s not always about technology.
The Future of Artificial Intelligence and Deep Learning
LeCun acknowledged that some AI challenges that people have invested significant resources in have not yet been solved, such as autonomous driving. "I would say others underestimate the complexity of it," he said, adding that he didn't put himself in that category.
"I know it's hard and it's going to take a long time," he claimed. "I disagree with some people who say we basically have it figured out... it's just a matter of making these models bigger." In fact, LeCun recently released a report creating A blueprint for "autonomous machine intelligence", which also shows that he believes that current artificial intelligence methods cannot achieve human-level artificial intelligence.
But he also sees the huge potential of deep learning in the future, saying that he is most excited about making machines learn more efficiently and more like animals and humans.
LeCun said the big question for him personally is what are the basic principles of animal learning, which is one of the reasons he has been advocating for things like self-supervised learning.
"This progress will allow us to build things that are currently out of reach, like intelligent systems that can power our daily lives as if they were human assistants. This is what we are going to need. , because everyone will be wearing AR glasses and we will have to interact with them."
Hinton agreed that more progress is being made in deep learning. In addition to advances in robotics, he also believes there will be another breakthrough in neural network computing infrastructure, because current facilities only perform numerical calculations with accelerators that are very good at doing matrix multipliers. For backpropagation, he said, analog signals need to be converted into digital signals.
Li Feifei believes that the most important thing for the future of deep learning is communication and education. “At Stanford HAI, we actually spend a disproportionate amount of energy reaching out to business leaders, government, policymakers, the media, reporters and journalists, and society at large and creating symposiums, conferences, workshops, publishing policy briefs, Industry briefing."
How 10 years of deep learning will be remembered
For Hinton, deep learning has been more successful than imagined in the past decade, but he also emphasized , this huge progress should be attributed to "the advancement of computer hardware."
Marcus is the role of a critic who believes that while deep learning has made some progress, it may later appear to be a misfortune.
"I think people in 2050 are going to look at these systems starting in 2022 and say: Yes, they're brave, but they don't really work."
But Li Feifei hopes that the past decade will be remembered as "the beginning of the great digital revolution": "It has made life and work better for everyone, not just a few or some of humanity."
She also added that as a scientist, "I would never think that today's deep learning is the end of artificial intelligence exploration."
On a social level, she said she hopes to Artificial Intelligence is viewed as “an incredible technological tool that is being developed and used in the most human-centered way possible – we must recognize the far-reaching impact of this tool and embrace a human-centered thinking framework as well as designing and deploying AI ."
Finally, Li Feifei said: "How we are remembered depends on what we are doing now."
The above is the detailed content of Has deep learning hit a wall after ten years? Hinton, LeCun, and Li Feifei don't think so.. For more information, please follow other related articles on the PHP Chinese website!
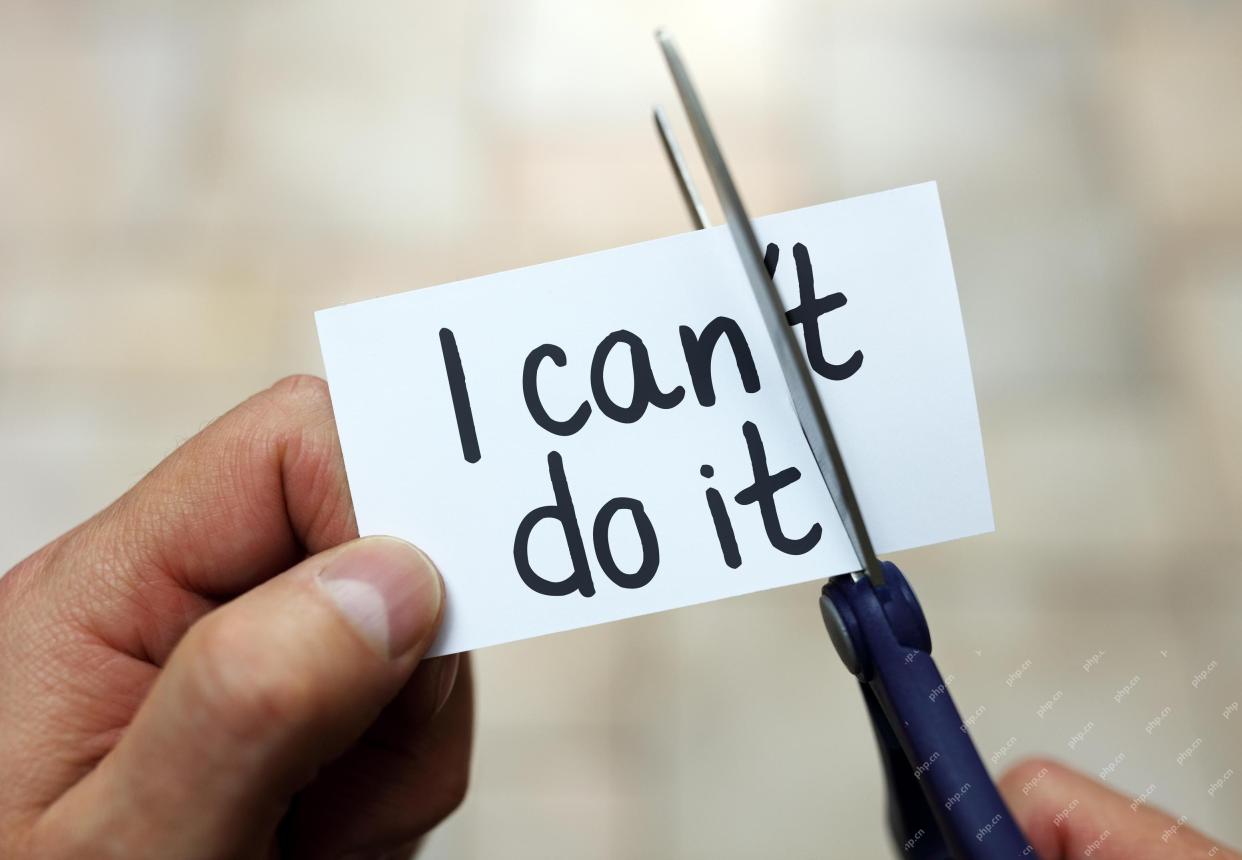
This article explores the growing concern of "AI agency decay"—the gradual decline in our ability to think and decide independently. This is especially crucial for business leaders navigating the increasingly automated world while retainin
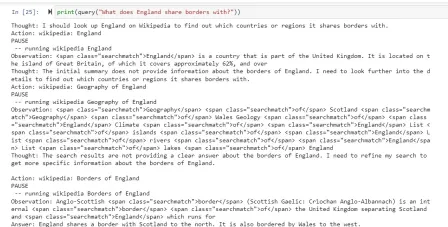
Ever wondered how AI agents like Siri and Alexa work? These intelligent systems are becoming more important in our daily lives. This article introduces the ReAct pattern, a method that enhances AI agents by combining reasoning an
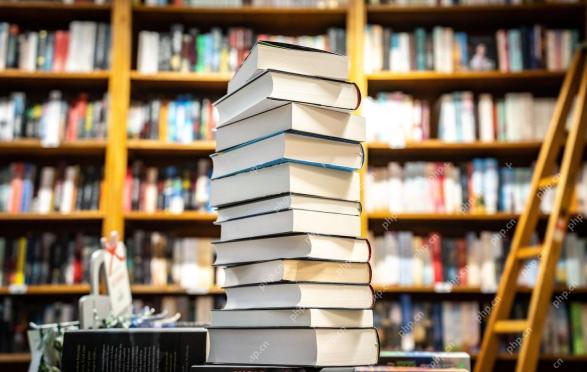
"I think AI tools are changing the learning opportunities for college students. We believe in developing students in core courses, but more and more people also want to get a perspective of computational and statistical thinking," said University of Chicago President Paul Alivisatos in an interview with Deloitte Nitin Mittal at the Davos Forum in January. He believes that people will have to become creators and co-creators of AI, which means that learning and other aspects need to adapt to some major changes. Digital intelligence and critical thinking Professor Alexa Joubin of George Washington University described artificial intelligence as a “heuristic tool” in the humanities and explores how it changes
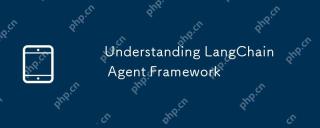
LangChain is a powerful toolkit for building sophisticated AI applications. Its agent architecture is particularly noteworthy, allowing developers to create intelligent systems capable of independent reasoning, decision-making, and action. This expl
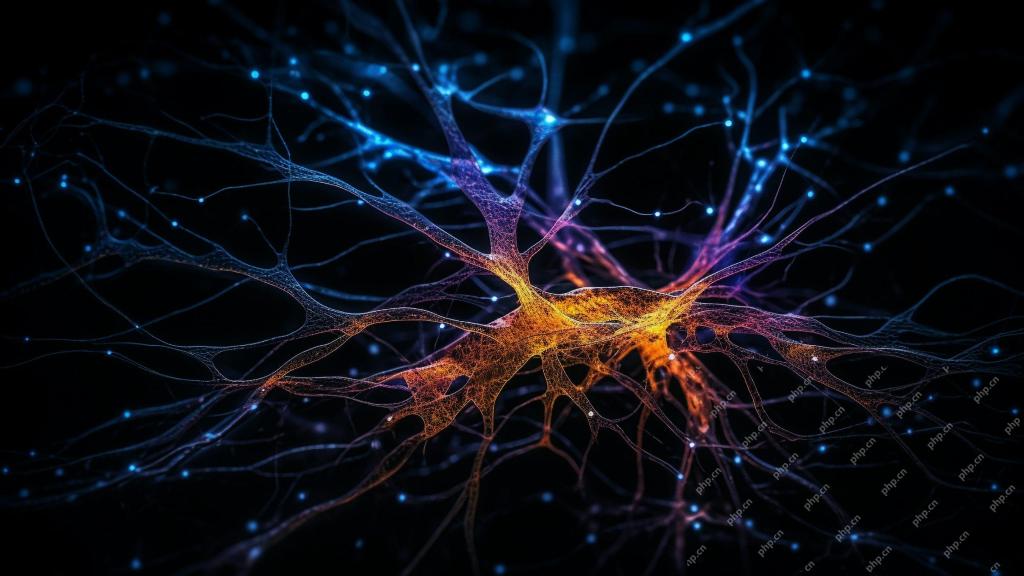
Radial Basis Function Neural Networks (RBFNNs): A Comprehensive Guide Radial Basis Function Neural Networks (RBFNNs) are a powerful type of neural network architecture that leverages radial basis functions for activation. Their unique structure make
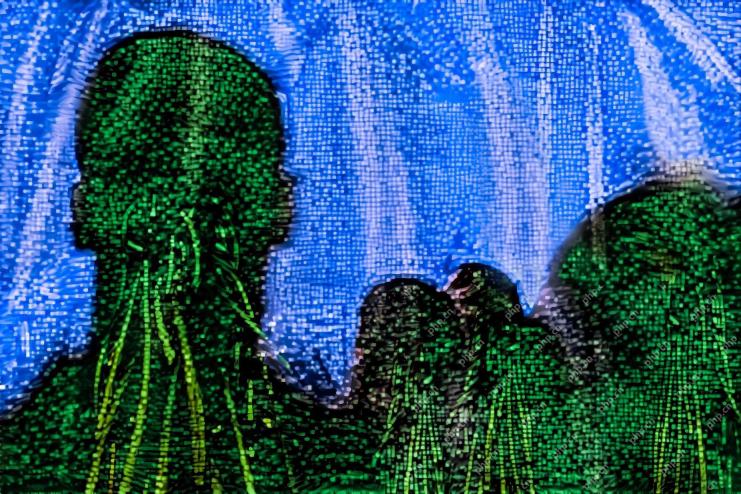
Brain-computer interfaces (BCIs) directly link the brain to external devices, translating brain impulses into actions without physical movement. This technology utilizes implanted sensors to capture brain signals, converting them into digital comman
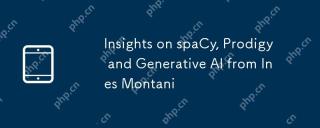
This "Leading with Data" episode features Ines Montani, co-founder and CEO of Explosion AI, and co-developer of spaCy and Prodigy. Ines offers expert insights into the evolution of these tools, Explosion's unique business model, and the tr
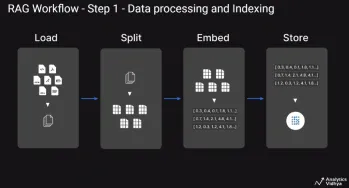
This article explores Retrieval Augmented Generation (RAG) systems and how AI agents can enhance their capabilities. Traditional RAG systems, while useful for leveraging custom enterprise data, suffer from limitations such as a lack of real-time dat


Hot AI Tools

Undresser.AI Undress
AI-powered app for creating realistic nude photos

AI Clothes Remover
Online AI tool for removing clothes from photos.

Undress AI Tool
Undress images for free

Clothoff.io
AI clothes remover

Video Face Swap
Swap faces in any video effortlessly with our completely free AI face swap tool!

Hot Article

Hot Tools

SecLists
SecLists is the ultimate security tester's companion. It is a collection of various types of lists that are frequently used during security assessments, all in one place. SecLists helps make security testing more efficient and productive by conveniently providing all the lists a security tester might need. List types include usernames, passwords, URLs, fuzzing payloads, sensitive data patterns, web shells, and more. The tester can simply pull this repository onto a new test machine and he will have access to every type of list he needs.

DVWA
Damn Vulnerable Web App (DVWA) is a PHP/MySQL web application that is very vulnerable. Its main goals are to be an aid for security professionals to test their skills and tools in a legal environment, to help web developers better understand the process of securing web applications, and to help teachers/students teach/learn in a classroom environment Web application security. The goal of DVWA is to practice some of the most common web vulnerabilities through a simple and straightforward interface, with varying degrees of difficulty. Please note that this software

SAP NetWeaver Server Adapter for Eclipse
Integrate Eclipse with SAP NetWeaver application server.

MinGW - Minimalist GNU for Windows
This project is in the process of being migrated to osdn.net/projects/mingw, you can continue to follow us there. MinGW: A native Windows port of the GNU Compiler Collection (GCC), freely distributable import libraries and header files for building native Windows applications; includes extensions to the MSVC runtime to support C99 functionality. All MinGW software can run on 64-bit Windows platforms.

Safe Exam Browser
Safe Exam Browser is a secure browser environment for taking online exams securely. This software turns any computer into a secure workstation. It controls access to any utility and prevents students from using unauthorized resources.