


Use 2D images to create a 3D human body. You can wear any clothes and change your movements.
Thanks to the differentiable rendering provided by NeRF, recent 3D generative models have achieved stunning results on stationary objects. However, in a more complex and deformable category such as the human body, 3D generation still poses great challenges. This paper proposes an efficient combined NeRF representation of the human body, enabling high-resolution (512x256) 3D human body generation without the use of super-resolution models. EVA3D has significantly surpassed existing solutions on four large-scale human body data sets, and the code has been open source.
- ##Thesis name: EVA3D: Compositional 3D Human Generation from 2D image Collections
- Paper address: https://arxiv.org/abs/2210.04888
- Project homepage: https://hongfz16.github.io/projects/EVA3D.html
- Open source code: https://github.com/hongfz16/EVA3D
- Colab Demo: https://colab.research.google. com/github/hongfz16/EVA3D/blob/main/notebook/EVA3D_Demo.ipynb
- Hugging Face Demo: https://huggingface.co/spaces/hongfz16/EVA3D
#Background
In order to solve this problem, this paper proposes an efficient combined 3D human body NeRF representation to achieve high-resolution (512x256) 3D human body GAN training and generation. The human NeRF representation proposed in this article and the three-dimensional human GAN training framework will be introduced below.
Efficient Human NeRF Representation
The human NeRF proposed in this article is based on the parametric human model SMPL, which provides convenient control of human posture and shape. When doing NeRF modeling, as shown in the figure below, this article divides the human body into 16 parts. Each part corresponds to a small NeRF network for local modeling. When rendering each part, this paper only needs to reason about the local NeRF. This sparse rendering method can also achieve native high-resolution rendering with lower computing resources.For example, when rendering a human body whose body and action parameters are inverse linear blend skinning), convert the sampling points in posed space into canonical space. Then it is calculated that the sampling points in the Canonical space belong to the bounding box of one or several local NeRFs, and then the NeRF model is inferred to obtain the color and density corresponding to each sampling point; when a certain sampling point falls into multiple local NeRFs In the overlapping area, each NeRF model will be inferred, and multiple results will be interpolated using the window function; finally, this information will be used for light integration to obtain the final rendering.
Three-dimensional human body GAN framework
Based on the proposed efficient human NeRF expression, this article implements a three-dimensional human body GAN training framework. In each training iteration, this paper first samples an SMPL parameter and camera parameters from the data set, and randomly generates a Gaussian noise z. Using the human body NeRF proposed in this article, this article can render the sampled parameters into a two-dimensional human body picture as a fake sample. Using real samples in the data set, this article conducts adversarial training of GAN.
Extremely imbalanced data sets
Two-dimensional human body data sets, such as DeepFashion, are usually It is prepared for two-dimensional vision tasks, so the posture diversity of the human body is very limited. To quantify the degree of imbalance, this paper counts the frequency of model face orientations in DeepFashion. As shown in the figure below, the orange line represents the distribution of face orientations in DeepFashion. It can be seen that it is extremely unbalanced, which makes it difficult to learn three-dimensional human body representation. To alleviate this problem, we propose a sampling method guided by human posture to flatten the distribution curve, as shown by the other colored lines in the figure below. This allows the model during training to see more diverse and larger angle images of the human body, thereby helping to learn three-dimensional human geometry. We conducted an experimental analysis of the sampling parameters. As can be seen from the table below, after adding the human posture guidance sampling method, although the image quality (FID) will be slightly reduced, the learned three-dimensional geometry (Depth) is significantly better.
High-quality generation results
The following figure shows some EVA3D generation results. EVA3D can randomly sample human body appearance, and can control rendering camera parameters and human postures. and body shape.
This paper conducts experiments on four large-scale human data sets, namely DeepFashion, SHHQ, UBCFashion, and AIST . This study compares the state-of-the-art static 3D object generation algorithm EG3D with StyleSDF. At the same time, the researchers also compared the algorithm ENARF-GAN specifically for 3D human generation. In the selection of indicators, this article takes into account the evaluation of rendering quality (FID/KID), the accuracy of human body control (PCK) and the quality of geometry generation (Depth). As shown in the figure below, this article significantly surpasses previous solutions in all data sets and all indicators.
Application potential
Finally, this article also shows some application potential of EVA3D. First, the study tested differencing in the latent space. As shown in the figure below, this article is able to make smooth changes between two three-dimensional people, and the intermediate results maintain high quality. In addition, this article also conducted experiments on GAN inversion. The researchers used Pivotal Tuning Inversion, an algorithm commonly used in two-dimensional GAN inversion. As shown in the right figure below, this method can better restore the appearance of the reconstructed target, but a lot of details are lost in the geometric part. It can be seen that the inversion of three-dimensional GAN is still a very challenging task.
Conclusion
This paper proposes the first high-definition three-dimensional human NeRF generation algorithm EVA3D, and only needs It can be trained using 2D human body image data. EVA3D achieves state-of-the-art performance on multiple large-scale human datasets and shows potential for application on downstream tasks. The training and testing codes of EVA3D have been open sourced, and everyone is welcome to try it!
The above is the detailed content of Use 2D images to create a 3D human body. You can wear any clothes and change your movements.. For more information, please follow other related articles on the PHP Chinese website!
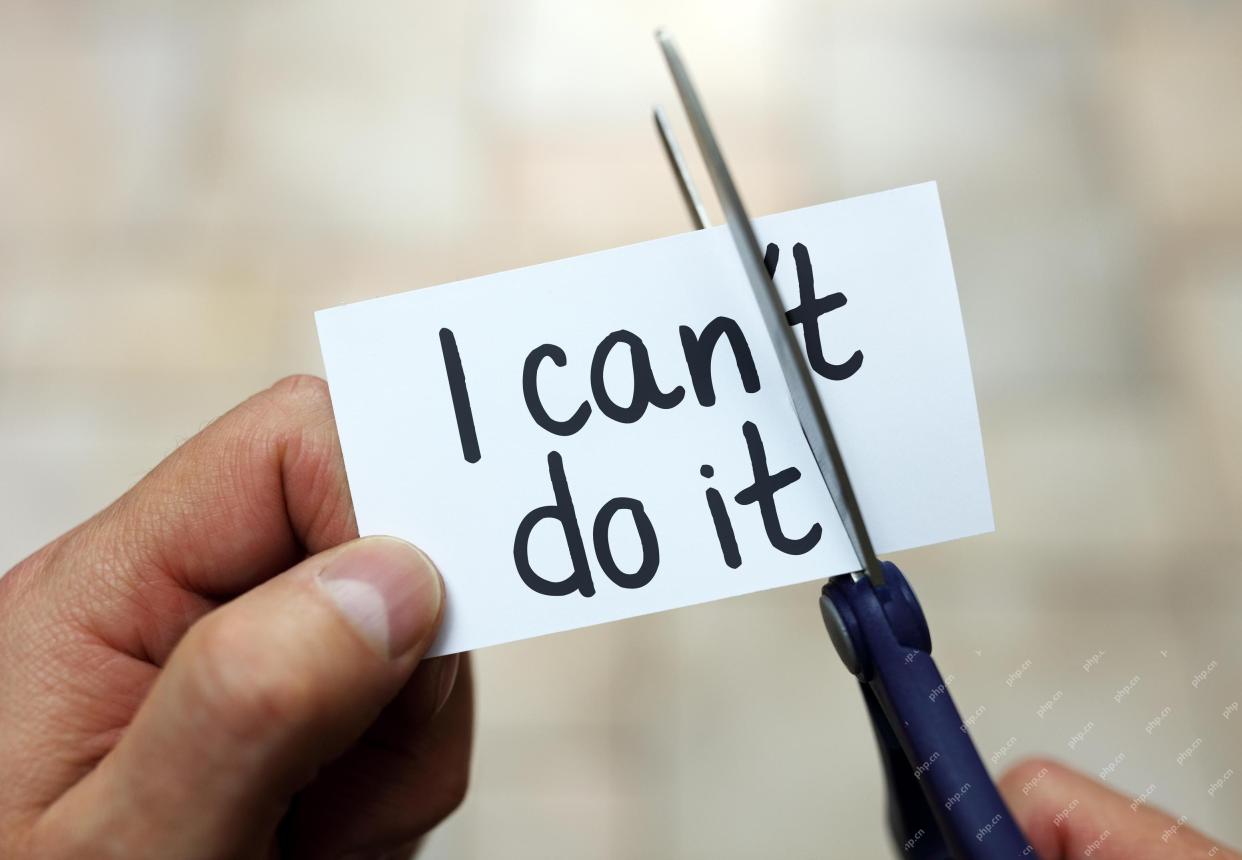
This article explores the growing concern of "AI agency decay"—the gradual decline in our ability to think and decide independently. This is especially crucial for business leaders navigating the increasingly automated world while retainin
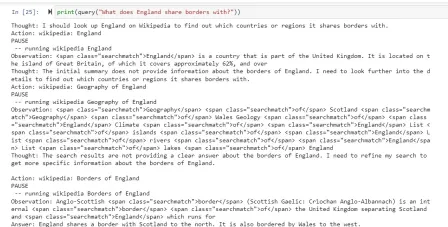
Ever wondered how AI agents like Siri and Alexa work? These intelligent systems are becoming more important in our daily lives. This article introduces the ReAct pattern, a method that enhances AI agents by combining reasoning an
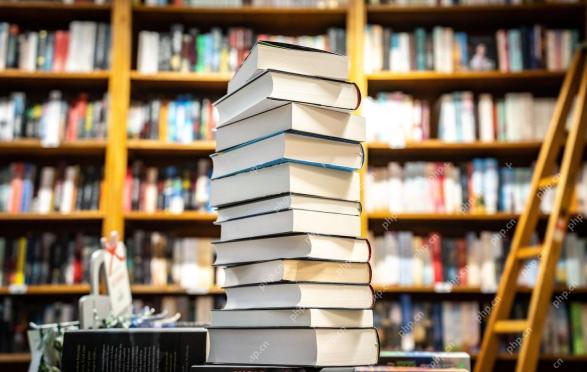
"I think AI tools are changing the learning opportunities for college students. We believe in developing students in core courses, but more and more people also want to get a perspective of computational and statistical thinking," said University of Chicago President Paul Alivisatos in an interview with Deloitte Nitin Mittal at the Davos Forum in January. He believes that people will have to become creators and co-creators of AI, which means that learning and other aspects need to adapt to some major changes. Digital intelligence and critical thinking Professor Alexa Joubin of George Washington University described artificial intelligence as a “heuristic tool” in the humanities and explores how it changes
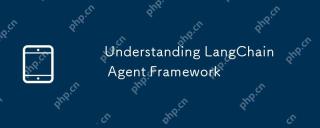
LangChain is a powerful toolkit for building sophisticated AI applications. Its agent architecture is particularly noteworthy, allowing developers to create intelligent systems capable of independent reasoning, decision-making, and action. This expl
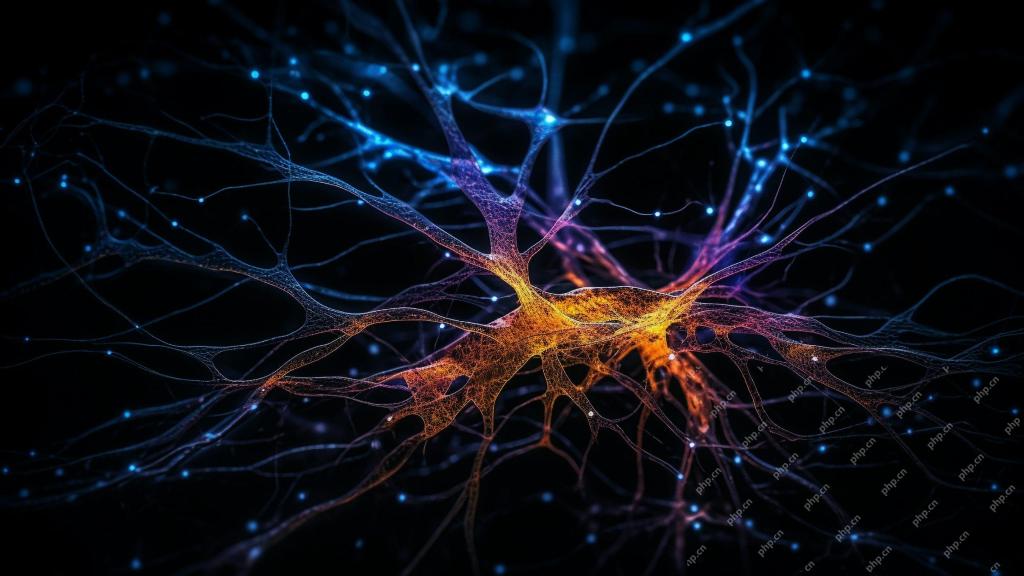
Radial Basis Function Neural Networks (RBFNNs): A Comprehensive Guide Radial Basis Function Neural Networks (RBFNNs) are a powerful type of neural network architecture that leverages radial basis functions for activation. Their unique structure make
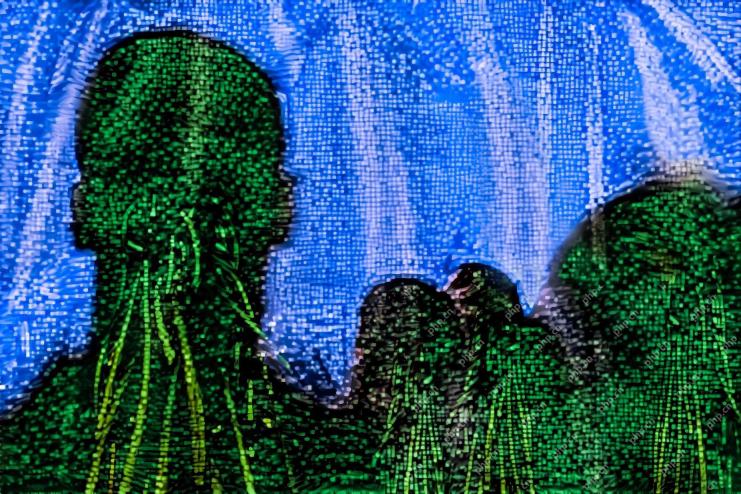
Brain-computer interfaces (BCIs) directly link the brain to external devices, translating brain impulses into actions without physical movement. This technology utilizes implanted sensors to capture brain signals, converting them into digital comman
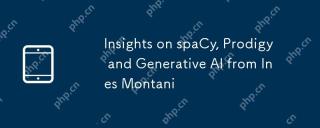
This "Leading with Data" episode features Ines Montani, co-founder and CEO of Explosion AI, and co-developer of spaCy and Prodigy. Ines offers expert insights into the evolution of these tools, Explosion's unique business model, and the tr
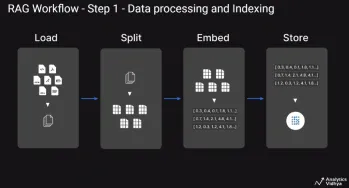
This article explores Retrieval Augmented Generation (RAG) systems and how AI agents can enhance their capabilities. Traditional RAG systems, while useful for leveraging custom enterprise data, suffer from limitations such as a lack of real-time dat


Hot AI Tools

Undresser.AI Undress
AI-powered app for creating realistic nude photos

AI Clothes Remover
Online AI tool for removing clothes from photos.

Undress AI Tool
Undress images for free

Clothoff.io
AI clothes remover

Video Face Swap
Swap faces in any video effortlessly with our completely free AI face swap tool!

Hot Article

Hot Tools

SecLists
SecLists is the ultimate security tester's companion. It is a collection of various types of lists that are frequently used during security assessments, all in one place. SecLists helps make security testing more efficient and productive by conveniently providing all the lists a security tester might need. List types include usernames, passwords, URLs, fuzzing payloads, sensitive data patterns, web shells, and more. The tester can simply pull this repository onto a new test machine and he will have access to every type of list he needs.

DVWA
Damn Vulnerable Web App (DVWA) is a PHP/MySQL web application that is very vulnerable. Its main goals are to be an aid for security professionals to test their skills and tools in a legal environment, to help web developers better understand the process of securing web applications, and to help teachers/students teach/learn in a classroom environment Web application security. The goal of DVWA is to practice some of the most common web vulnerabilities through a simple and straightforward interface, with varying degrees of difficulty. Please note that this software

SAP NetWeaver Server Adapter for Eclipse
Integrate Eclipse with SAP NetWeaver application server.

MinGW - Minimalist GNU for Windows
This project is in the process of being migrated to osdn.net/projects/mingw, you can continue to follow us there. MinGW: A native Windows port of the GNU Compiler Collection (GCC), freely distributable import libraries and header files for building native Windows applications; includes extensions to the MSVC runtime to support C99 functionality. All MinGW software can run on 64-bit Windows platforms.

Safe Exam Browser
Safe Exam Browser is a secure browser environment for taking online exams securely. This software turns any computer into a secure workstation. It controls access to any utility and prevents students from using unauthorized resources.