Closures in Python
Someone left a message a few days ago, and it is unclear about the use of one of the closures
and re.sub
. I searched on Script Home and found that I had never written anything related to closures, so I decided to summarize and improve the content of Python.
1. The concept of closure
First of all, we have to start with the basic concept. What is closure? Let’s take a look at the explanation on Wiki:
....
Two key points are mentioned above: free variables and functions. These two keys will be discussed later. I still have to elaborate on the meaning of "closure". As the text makes clear, it can be understood vividly as a closed package. This package is a function. Of course, there is also the corresponding logic inside the function. What is inside the package is freedom. Variables, free variables can wander around with the package. Of course, there must be a premise that this package is created.
For example:
def func(name): def inner_func(age): print 'name:', name, 'age:', age return inner_func bb = func('the5fire') bb(26) # >>> name: the5fire age: 26
When func is called here, a closure - inner_func is generated, and the closure holds the free variable - name, so this also means that when the life cycle of function func ends, the variable name still exists. Because it is referenced by the closure, it will not be recycled.
In addition, closure is not a unique concept in Python. All languages that treat functions as first-class citizens have the concept of closure. However, closures can also be used in languages like Java where classes are first-class citizens, but they must be implemented using classes or interfaces.
For more conceptual things, please refer to the reference link at the end.
2. Why use closures
Based on the above introduction, I wonder if readers feel that this thing is somewhat similar to classes. The similarity is that they both provide data encapsulation. The difference is that the closure itself is a method. Just like classes, we often abstract common things into classes when programming (of course, as well as modeling the real world-business) to reuse common functions. The same is true for closures. When we need function-granular abstraction, closures are a good choice.
At this point, a closure can be understood as a read-only object. You can pass a property to it, but it can only provide you with an execution interface. Therefore, in programs we often need such a function object - closure, to help us complete a common function, such as the decorator which will be mentioned later.
3. Use closures
The first scenario, a very important and common usage scenario in Python is the decorator. Python provides a very friendly "syntax sugar" for the decorator - @, which allows us to use the decorator very conveniently. I won’t elaborate too much on the principle of decoration. In short, if you add @decorator_func to a function func, it is equivalent to decorator_func(func):
def decorator_func(func): def wrapper(*args, **kwargs): return func(*args, **kwargs) return wrapper @decorator_func def func(name): print 'my name is', name # 等价于 decorator_func(func)
In this example of the decorator, the closure (wrapper) holds the external func parameter and can accept external parameters. The accepted parameters are passed to func intact and the execution result is returned.
This is a simple example. If it is slightly more complicated, you can have multiple closures, such as the frequently used LRUCache decorator. The decorator can accept parameters like @lru_cache(expire=500). The implementation is the nesting of two closures:
def lru_cache(expire=5): # 默认5s超时 def func_wrapper(func): def inner(*args, **kwargs): # cache 处理 bala bala bala return func(*args, **kwargs) return inner return func_wrapper @lru_cache(expire=10*60) def get(request, pk) # 省略具体代码 return response()
Students who don’t know much about closures must be able to understand the above code. This is an interview question we often asked in previous interviews.
The second scenario is based on a feature of closure-"lazy evaluation". This application is more common when accessing the database, for example:
# 伪代码示意 class QuerySet(object): def __init__(self, sql): self.sql = sql self.db = Mysql.connect().corsor() # 伪代码 def __call__(self): return db.execute(self.sql) def query(sql): return QuerySet(sql) result = query("select name from user_app") if time > now: print result # 这时才执行数据库访问
The above inappropriate example shows the function of lazy evaluation through closure, but the result returned by the above query is not a function, but a class with functional functions. If you are interested, you can take a look at the implementation of Django's queryset. The principle is similar.
The third scenario is the situation where the parameters of a certain function need to be assigned values in advance. Of course, there is already a good solution in Python to access functools.parial, but it can also be achieved using closures.
def partial(**outer_kwargs): def wrapper(func): def inner(*args, **kwargs): for k, v in outer_kwargs.items(): kwargs[k] = v return func(*args, **kwargs) return inner return wrapper @partial(age=15) def say(name=None, age=None): print name, age say(name="the5fire") # 当然用functools比这个简单多了 # 只需要: functools.partial(say, age=15)(name='the5fire')
It seems like this is another far-fetched example, but it can be regarded as a practical application of closures.
Finally, to summarize, closures are easy to understand and are widely used in Python. This article is a summary of closures. If you have any questions, please leave a message.
4. References
Wikipedia - Closures
http://stackoverflow.com/questions/4020419/closures-in-python
http://www.shutupandship.com/2012/01/python-closures-explained.html
http://stackoverflow.com/questions/141642/what-limitations-have-closures-in-python-compared-to-language-x-closures
http://mrevelle.blogspot.com/2006/10/closure-on-closures.html
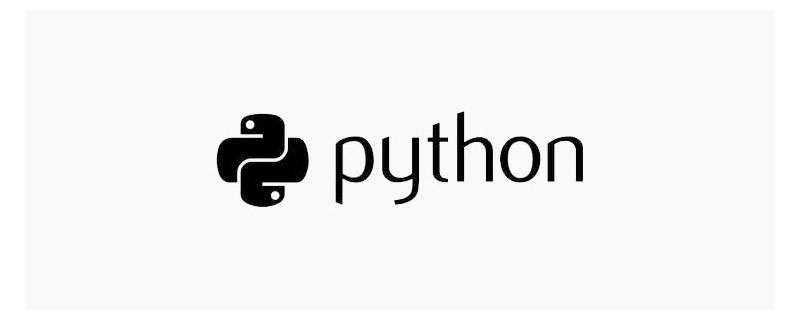
本篇文章给大家带来了关于Python的相关知识,其中主要介绍了关于Seaborn的相关问题,包括了数据可视化处理的散点图、折线图、条形图等等内容,下面一起来看一下,希望对大家有帮助。
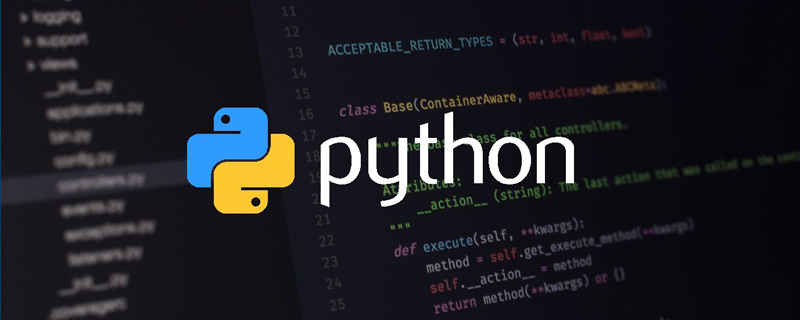
本篇文章给大家带来了关于Python的相关知识,其中主要介绍了关于进程池与进程锁的相关问题,包括进程池的创建模块,进程池函数等等内容,下面一起来看一下,希望对大家有帮助。
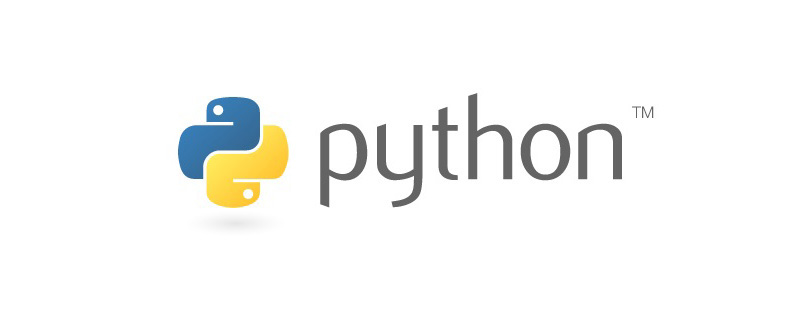
本篇文章给大家带来了关于Python的相关知识,其中主要介绍了关于简历筛选的相关问题,包括了定义 ReadDoc 类用以读取 word 文件以及定义 search_word 函数用以筛选的相关内容,下面一起来看一下,希望对大家有帮助。
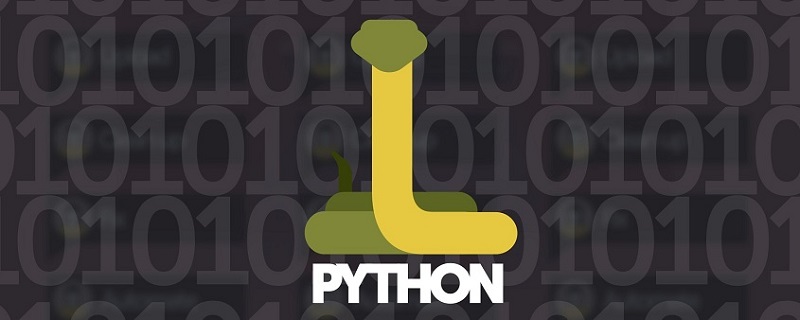
本篇文章给大家带来了关于Python的相关知识,其中主要介绍了关于数据类型之字符串、数字的相关问题,下面一起来看一下,希望对大家有帮助。
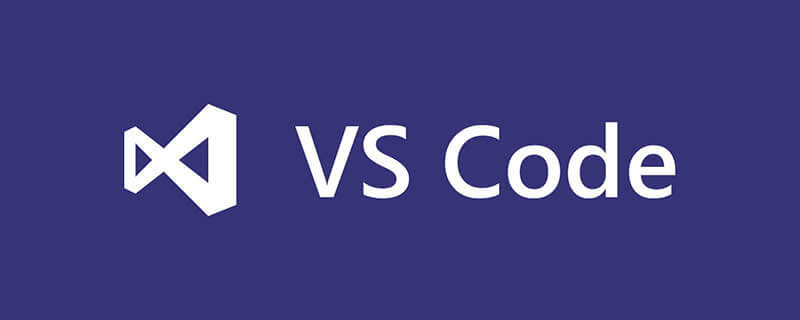
VS Code的确是一款非常热门、有强大用户基础的一款开发工具。本文给大家介绍一下10款高效、好用的插件,能够让原本单薄的VS Code如虎添翼,开发效率顿时提升到一个新的阶段。
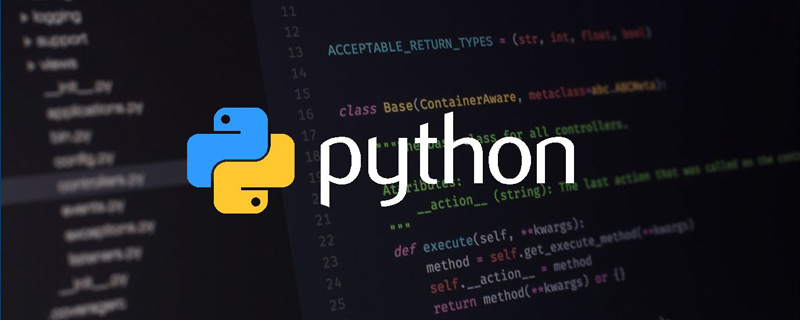
本篇文章给大家带来了关于Python的相关知识,其中主要介绍了关于numpy模块的相关问题,Numpy是Numerical Python extensions的缩写,字面意思是Python数值计算扩展,下面一起来看一下,希望对大家有帮助。
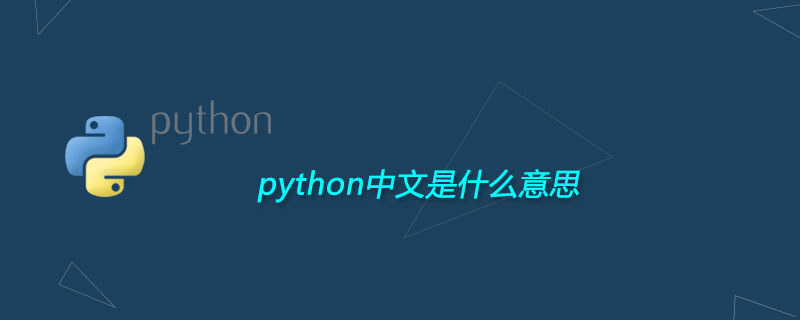
pythn的中文意思是巨蟒、蟒蛇。1989年圣诞节期间,Guido van Rossum在家闲的没事干,为了跟朋友庆祝圣诞节,决定发明一种全新的脚本语言。他很喜欢一个肥皂剧叫Monty Python,所以便把这门语言叫做python。


Hot AI Tools

Undresser.AI Undress
AI-powered app for creating realistic nude photos

AI Clothes Remover
Online AI tool for removing clothes from photos.

Undress AI Tool
Undress images for free

Clothoff.io
AI clothes remover

AI Hentai Generator
Generate AI Hentai for free.

Hot Article

Hot Tools

MinGW - Minimalist GNU for Windows
This project is in the process of being migrated to osdn.net/projects/mingw, you can continue to follow us there. MinGW: A native Windows port of the GNU Compiler Collection (GCC), freely distributable import libraries and header files for building native Windows applications; includes extensions to the MSVC runtime to support C99 functionality. All MinGW software can run on 64-bit Windows platforms.
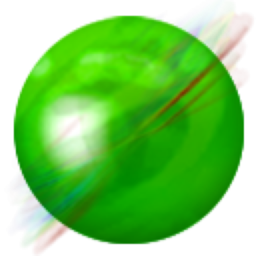
ZendStudio 13.5.1 Mac
Powerful PHP integrated development environment
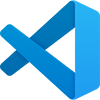
VSCode Windows 64-bit Download
A free and powerful IDE editor launched by Microsoft

DVWA
Damn Vulnerable Web App (DVWA) is a PHP/MySQL web application that is very vulnerable. Its main goals are to be an aid for security professionals to test their skills and tools in a legal environment, to help web developers better understand the process of securing web applications, and to help teachers/students teach/learn in a classroom environment Web application security. The goal of DVWA is to practice some of the most common web vulnerabilities through a simple and straightforward interface, with varying degrees of difficulty. Please note that this software

mPDF
mPDF is a PHP library that can generate PDF files from UTF-8 encoded HTML. The original author, Ian Back, wrote mPDF to output PDF files "on the fly" from his website and handle different languages. It is slower than original scripts like HTML2FPDF and produces larger files when using Unicode fonts, but supports CSS styles etc. and has a lot of enhancements. Supports almost all languages, including RTL (Arabic and Hebrew) and CJK (Chinese, Japanese and Korean). Supports nested block-level elements (such as P, DIV),