A Groundbreaking Paper on Dataset Diversity in Machine Learning
The machine learning (ML) community is abuzz over a recent ICML 2024 Best Paper Award winner that challenges the often-unsubstantiated claims of "diversity" in datasets. Researchers Dora Zhao, Jerone T. A. Andrews, Orestis Papakyriakopoulos, and Alice Xiang's work, "Measure Dataset Diversity, Don't Just Claim It," provides a much-needed framework for rigorously assessing dataset diversity.
This isn't just another paper on dataset diversity; it's a call to action. The authors critique the loose use of terms like "diversity," "quality," and "bias" without proper validation. Their solution? A structured approach using measurement theory principles to define, measure, and evaluate diversity in ML datasets.
The paper's framework involves three crucial steps:
- Conceptualization: Defining "diversity" within the specific context of the dataset.
- Operationalization: Developing concrete methods to quantify the defined aspects of diversity.
- Evaluation: Assessing the reliability and validity of the diversity measurements.
Key findings from their analysis of 135 image and text datasets reveal significant shortcomings: a lack of clear definitions of diversity, insufficient documentation of data collection, reliability concerns, and challenges in validating diversity claims. The researchers offer practical recommendations to address these issues, including using inter-annotator agreement and employing techniques from construct validity.
A case study of the Segment Anything dataset (SA-1B) highlights the framework's practical application, identifying both strengths and areas for improvement in its diversity considerations.
The implications are far-reaching: the paper challenges the assumption that larger datasets automatically equate to greater diversity, emphasizing the need for intentional curation. It also acknowledges the increased documentation burden but advocates for systemic changes in how data work is valued within the ML research community. Furthermore, it highlights the importance of considering how diversity constructs evolve over time.
Read the full paper: Position: Measure Dataset Diversity, Don't Just Claim It
The conclusion emphasizes the need for more rigorous, transparent, and reproducible research in ML. The authors' framework provides essential tools for ensuring that claims of dataset diversity are not merely rhetoric but demonstrably meaningful contributions to fairer and more robust AI systems. This work serves as a critical step toward improving dataset curation and documentation, ultimately leading to more reliable and equitable machine learning models.
While the increased rigor may seem demanding, the authors convincingly argue that building AI on shaky foundations is unacceptable. This paper isn't just about better datasets; it's about a more trustworthy and accountable field of machine learning.
Frequently Asked Questions:
- Q1: Why is measuring dataset diversity important? A1: It ensures diverse representation, reduces bias, improves model generalizability, and promotes fairness in AI.
- Q2: How does dataset diversity impact ML model performance? A2: It improves robustness and accuracy by reducing overfitting and enhancing performance across different populations and conditions.
- Q3: What are common challenges in measuring dataset diversity? A3: Defining diversity, operationalizing definitions, validating claims, and ensuring transparent and reproducible documentation.
- Q4: What are practical steps for improving dataset diversity? A4: Clearly defining diversity goals, collecting data from diverse sources, using standardized measurement methods, continuous evaluation, and implementing robust validation.
The above is the detailed content of This Research Paper Won the ICML 2024 Best Paper Award. For more information, please follow other related articles on the PHP Chinese website!
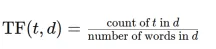
This article explains the Term Frequency-Inverse Document Frequency (TF-IDF) technique, a crucial tool in Natural Language Processing (NLP) for analyzing textual data. TF-IDF surpasses the limitations of basic bag-of-words approaches by weighting te
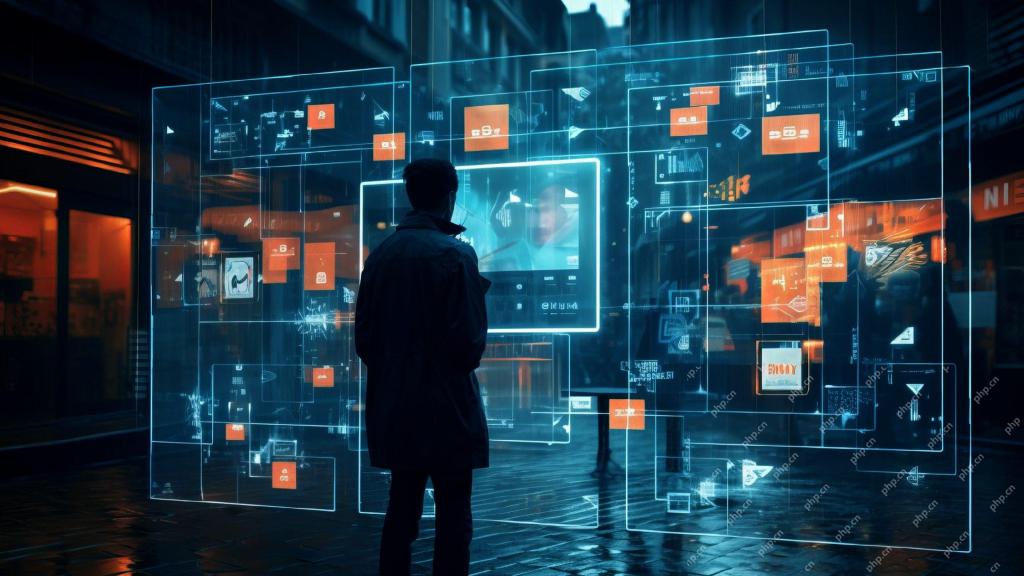
Unleash the Power of AI Agents with LangChain: A Beginner's Guide Imagine showing your grandmother the wonders of artificial intelligence by letting her chat with ChatGPT – the excitement on her face as the AI effortlessly engages in conversation! Th
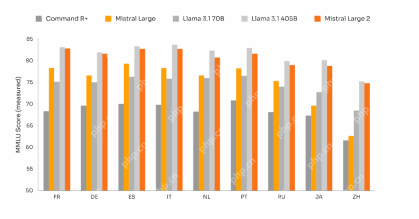
Mistral Large 2: A Deep Dive into Mistral AI's Powerful Open-Source LLM Meta AI's recent release of the Llama 3.1 family of models was quickly followed by Mistral AI's unveiling of its largest model to date: Mistral Large 2. This 123-billion paramet
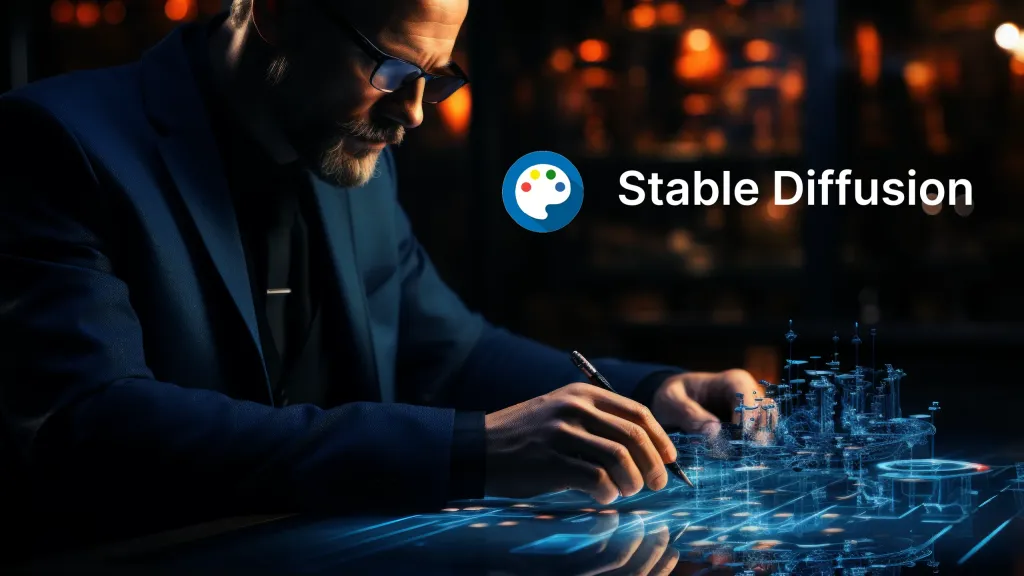
Understanding Noise Schedules in Diffusion Models: A Comprehensive Guide Have you ever been captivated by the stunning visuals of digital art generated by AI and wondered about the underlying mechanics? A key element is the "noise schedule,&quo
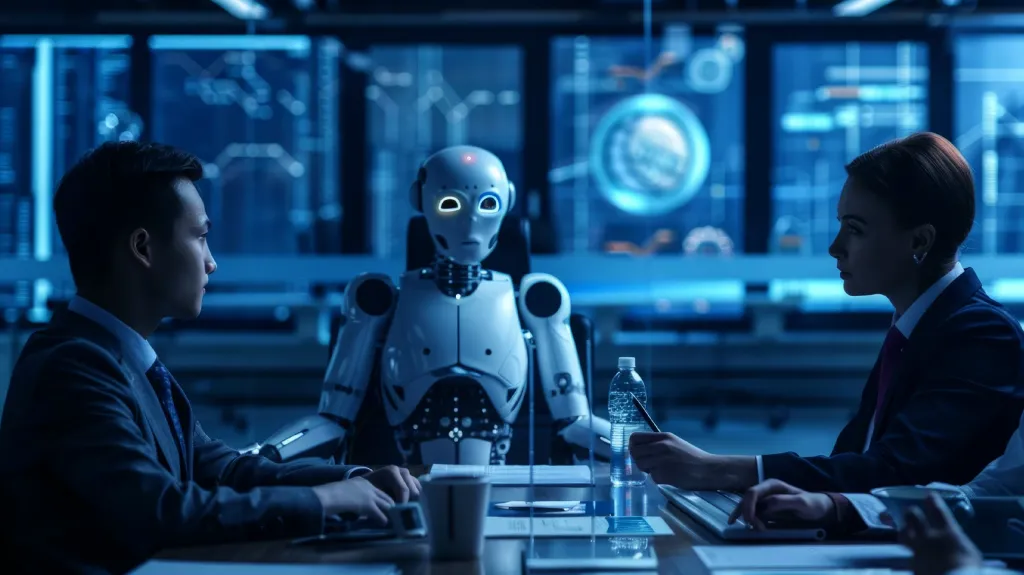
Building a Contextual Chatbot with GPT-4o: A Comprehensive Guide In the rapidly evolving landscape of AI and NLP, chatbots have become indispensable tools for developers and organizations. A key aspect of creating truly engaging and intelligent chat
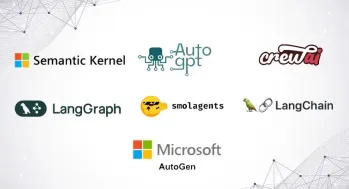
This article explores seven leading frameworks for building AI agents – autonomous software entities that perceive, decide, and act to achieve goals. These agents, surpassing traditional reinforcement learning, leverage advanced planning and reasoni
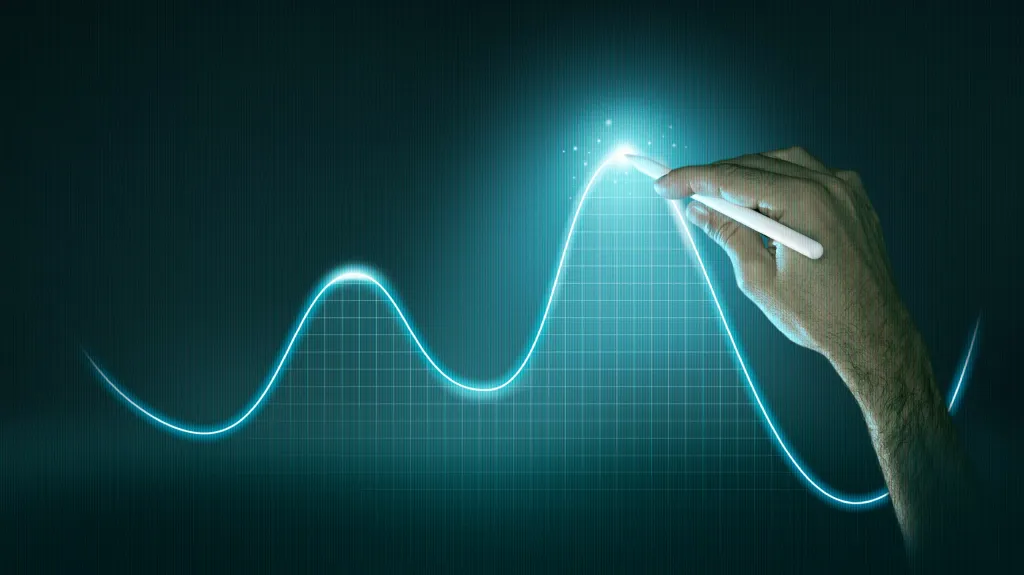
Understanding Type I and Type II Errors in Statistical Hypothesis Testing Imagine a clinical trial testing a new blood pressure medication. The trial concludes the drug significantly lowers blood pressure, but in reality, it doesn't. This is a Type

Sumy: Your AI-Powered Summarization Assistant Tired of sifting through endless documents? Sumy, a powerful Python library, offers a streamlined solution for automatic text summarization. This article explores Sumy's capabilities, guiding you throug


Hot AI Tools

Undresser.AI Undress
AI-powered app for creating realistic nude photos

AI Clothes Remover
Online AI tool for removing clothes from photos.

Undress AI Tool
Undress images for free

Clothoff.io
AI clothes remover

AI Hentai Generator
Generate AI Hentai for free.

Hot Article

Hot Tools

MinGW - Minimalist GNU for Windows
This project is in the process of being migrated to osdn.net/projects/mingw, you can continue to follow us there. MinGW: A native Windows port of the GNU Compiler Collection (GCC), freely distributable import libraries and header files for building native Windows applications; includes extensions to the MSVC runtime to support C99 functionality. All MinGW software can run on 64-bit Windows platforms.

SublimeText3 English version
Recommended: Win version, supports code prompts!

SublimeText3 Chinese version
Chinese version, very easy to use

SAP NetWeaver Server Adapter for Eclipse
Integrate Eclipse with SAP NetWeaver application server.

PhpStorm Mac version
The latest (2018.2.1) professional PHP integrated development tool