Sumy: Your AI-Powered Summarization Assistant
Tired of sifting through endless documents? Sumy, a powerful Python library, offers a streamlined solution for automatic text summarization. This article explores Sumy's capabilities, guiding you through installation, key functionalities, and various summarization algorithms. Transform information overload into actionable insights with ease.
Learning Objectives
- Grasp the advantages of using the Sumy library.
- Master Sumy's installation via PyPI and GitHub.
- Learn to create custom tokenizers and stemmers.
- Implement different summarization algorithms: Luhn, Edmundson, and LSA.
This article is part of the Data Science Blogathon.
Table of Contents
- What is the Sumy Library?
- Installing Sumy
- Building a Tokenizer
- Creating a Stemmer
- Summarization Algorithms
- Luhn Summarizer
- Edmundson Summarizer
- LSA Summarizer
- Frequently Asked Questions
What is the Sumy Library?
Sumy is a Python Natural Language Processing (NLP) library specializing in automatic text summarization. It leverages diverse algorithms including Luhn, Edmundson, LSA, LexRank, and KL-summarizers to generate concise summaries from lengthy texts. Its ease of use and integration with other NLP tools make it ideal for large-scale summarization tasks.
Benefits of Sumy
- Offers a range of summarization algorithms for flexible choices.
- Integrates seamlessly with other NLP libraries.
- Simple installation and usage.
- Efficiently handles lengthy documents.
- Customizable to meet specific summarization requirements.
Installing Sumy
Install via PyPI using your terminal:
pip install sumy
For Jupyter Notebook, Kaggle, or Google Colab, prefix the command with !
:
!pip install sumy
Building a Tokenizer
Tokenization (splitting text into sentences and words) is crucial for effective summarization. Sumy simplifies this process.
from sumy.nlp.tokenizers import Tokenizer import nltk nltk.download('punkt') tokenizer = Tokenizer("en") sentences = tokenizer.to_sentences("Sample text...") #Insert your sample text here for sentence in sentences: print(tokenizer.to_words(sentence))
Output:
Creating a Stemmer
Stemming (reducing words to their root form) improves summarization accuracy by treating variations of the same word as identical.
from sumy.nlp.stemmers import Stemmer stemmer = Stemmer("en") stem = stemmer("Blogging") print(stem)
Output:
Overview of Summarization Algorithms
Luhn Summarizer
This algorithm uses frequency analysis to rank sentences based on significant word frequency.
# ... (Luhn Summarizer code as in original article) ...
Output:
Edmundson Summarizer
This algorithm allows for a more customized approach using bonus, stigma, and null words to influence the summary.
# ... (Edmundson Summarizer code as in original article) ...
Output:
LSA Summarizer
LSA (Latent Semantic Analysis) identifies patterns and relationships between words for contextually richer summaries.
# ... (LSA Summarizer code as in original article) ...
Output:
Conclusion
Sumy is a versatile library for efficient text summarization. Its diverse algorithms and ease of use make it a valuable tool for data scientists and anyone working with large volumes of text.
Key Takeaways
- Sumy provides algorithm selection for tailored summarization.
- Easily creates tokenizers and stemmers.
- Offers various summarization algorithms with unique benefits.
- Efficiently handles extensive text documents.
Frequently Asked Questions
Q1. What is Sumy? A. A Python library for automatic text summarization.
Q2. What algorithms does Sumy support? A. Luhn, Edmundson, LSA, LexRank, and KL-summarizers.
Q3. What is tokenization in Sumy? A. Dividing text into sentences and words for improved accuracy.
Q4. What is stemming in Sumy? A. Reducing words to root forms for better summarization.
(Note: Images are assumed to be present as in the original input.)
The above is the detailed content of Automated Text Summarization with Sumy Library. For more information, please follow other related articles on the PHP Chinese website!
![[Ghibli-style images with AI] Introducing how to create free images with ChatGPT and copyright](https://img.php.cn/upload/article/001/242/473/174707263295098.jpg?x-oss-process=image/resize,p_40)
The latest model GPT-4o released by OpenAI not only can generate text, but also has image generation functions, which has attracted widespread attention. The most eye-catching feature is the generation of "Ghibli-style illustrations". Simply upload the photo to ChatGPT and give simple instructions to generate a dreamy image like a work in Studio Ghibli. This article will explain in detail the actual operation process, the effect experience, as well as the errors and copyright issues that need to be paid attention to. For details of the latest model "o3" released by OpenAI, please click here⬇️ Detailed explanation of OpenAI o3 (ChatGPT o3): Features, pricing system and o4-mini introduction Please click here for the English version of Ghibli-style article⬇️ Create Ji with ChatGPT
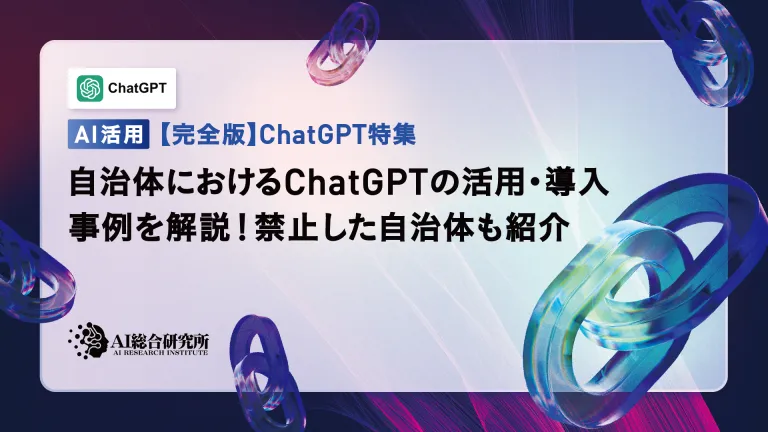
As a new communication method, the use and introduction of ChatGPT in local governments is attracting attention. While this trend is progressing in a wide range of areas, some local governments have declined to use ChatGPT. In this article, we will introduce examples of ChatGPT implementation in local governments. We will explore how we are achieving quality and efficiency improvements in local government services through a variety of reform examples, including supporting document creation and dialogue with citizens. Not only local government officials who aim to reduce staff workload and improve convenience for citizens, but also all interested in advanced use cases.
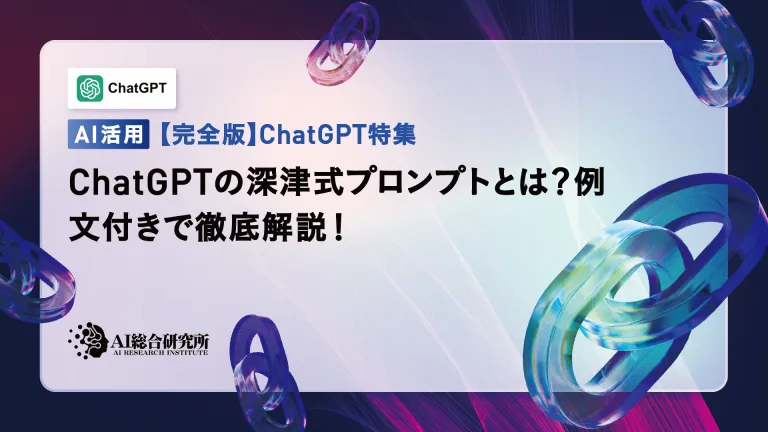
Have you heard of a framework called the "Fukatsu Prompt System"? Language models such as ChatGPT are extremely excellent, but appropriate prompts are essential to maximize their potential. Fukatsu prompts are one of the most popular prompt techniques designed to improve output accuracy. This article explains the principles and characteristics of Fukatsu-style prompts, including specific usage methods and examples. Furthermore, we have introduced other well-known prompt templates and useful techniques for prompt design, so based on these, we will introduce C.
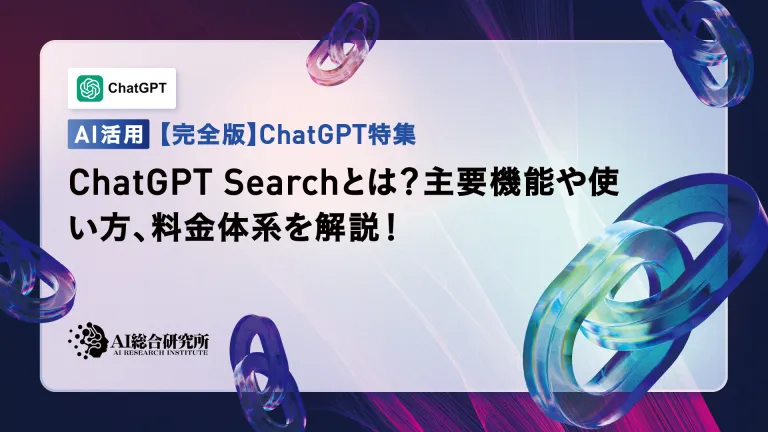
ChatGPT Search: Get the latest information efficiently with an innovative AI search engine! In this article, we will thoroughly explain the new ChatGPT feature "ChatGPT Search," provided by OpenAI. Let's take a closer look at the features, usage, and how this tool can help you improve your information collection efficiency with reliable answers based on real-time web information and intuitive ease of use. ChatGPT Search provides a conversational interactive search experience that answers user questions in a comfortable, hidden environment that hides advertisements
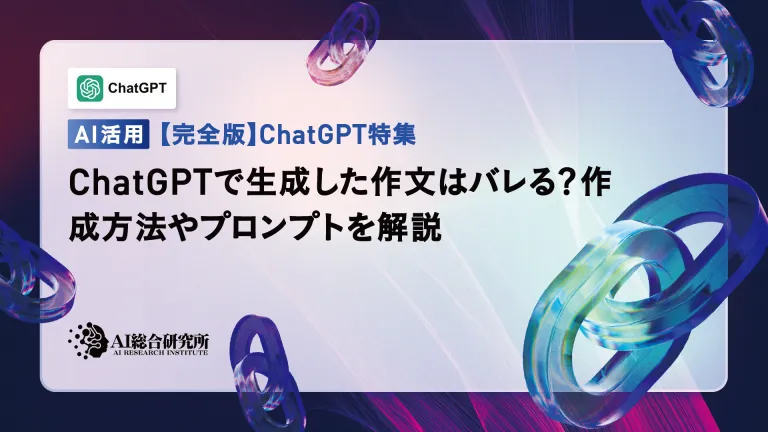
In a modern society with information explosion, it is not easy to create compelling articles. How to use creativity to write articles that attract readers within a limited time and energy requires superb skills and rich experience. At this time, as a revolutionary writing aid, ChatGPT attracted much attention. ChatGPT uses huge data to train language generation models to generate natural, smooth and refined articles. This article will introduce how to effectively use ChatGPT and efficiently create high-quality articles. We will gradually explain the writing process of using ChatGPT, and combine specific cases to elaborate on its advantages and disadvantages, applicable scenarios, and safe use precautions. ChatGPT will be a writer to overcome various obstacles,
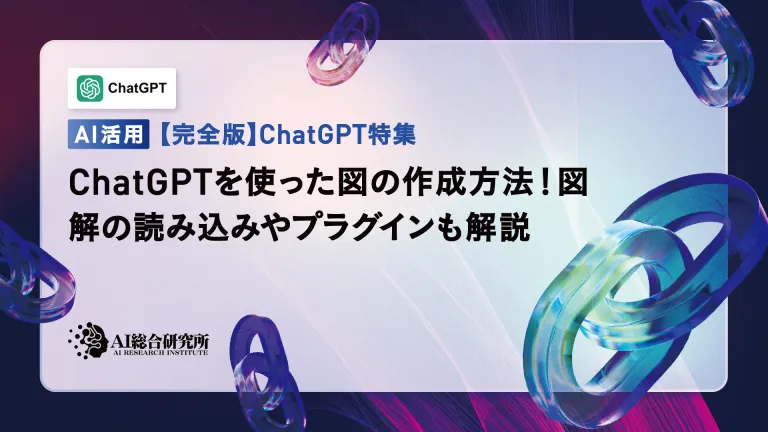
An efficient guide to creating charts using AI Visual materials are essential to effectively conveying information, but creating it takes a lot of time and effort. However, the chart creation process is changing dramatically due to the rise of AI technologies such as ChatGPT and DALL-E 3. This article provides detailed explanations on efficient and attractive diagram creation methods using these cutting-edge tools. It covers everything from ideas to completion, and includes a wealth of information useful for creating diagrams, from specific steps, tips, plugins and APIs that can be used, and how to use the image generation AI "DALL-E 3."
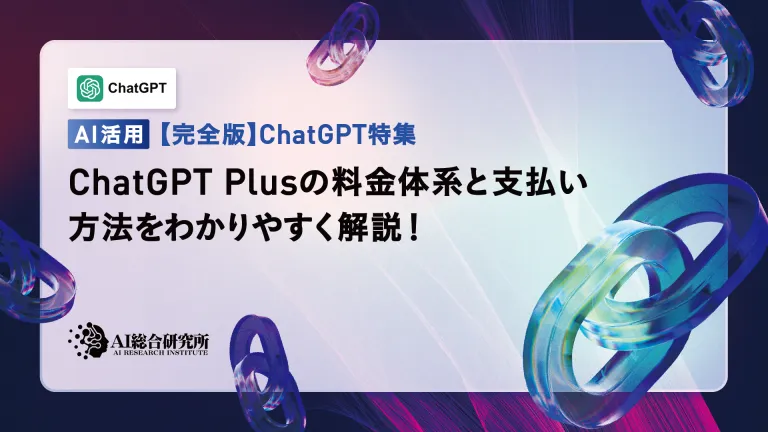
Unlock ChatGPT Plus: Fees, Payment Methods and Upgrade Guide ChatGPT, a world-renowned generative AI, has been widely used in daily life and business fields. Although ChatGPT is basically free, the paid version of ChatGPT Plus provides a variety of value-added services, such as plug-ins, image recognition, etc., which significantly improves work efficiency. This article will explain in detail the charging standards, payment methods and upgrade processes of ChatGPT Plus. For details of OpenAI's latest image generation technology "GPT-4o image generation" please click: Detailed explanation of GPT-4o image generation: usage methods, prompt word examples, commercial applications and differences from other AIs Table of contents ChatGPT Plus Fees Ch
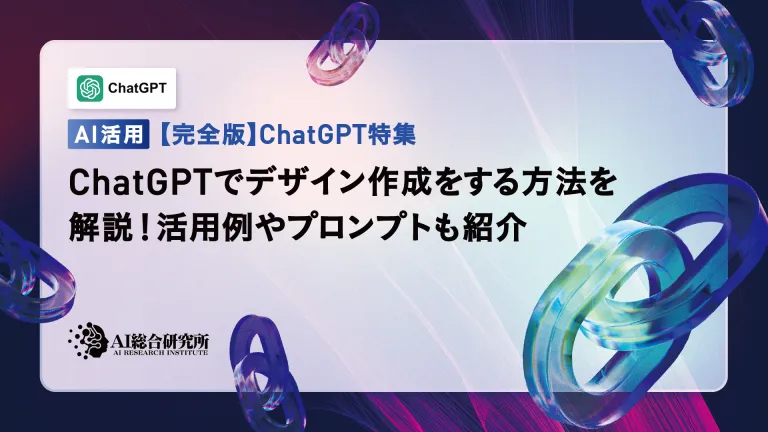
How to use ChatGPT to streamline your design work and increase creativity This article will explain in detail how to create a design using ChatGPT. We will introduce examples of using ChatGPT in various design fields, such as ideas, text generation, and web design. We will also introduce points that will help you improve the efficiency and quality of a variety of creative work, such as graphic design, illustration, and logo design. Please take a look at how AI can greatly expand your design possibilities. table of contents ChatGPT: A powerful tool for design creation


Hot AI Tools

Undresser.AI Undress
AI-powered app for creating realistic nude photos

AI Clothes Remover
Online AI tool for removing clothes from photos.

Undress AI Tool
Undress images for free

Clothoff.io
AI clothes remover

Video Face Swap
Swap faces in any video effortlessly with our completely free AI face swap tool!

Hot Article

Hot Tools

SecLists
SecLists is the ultimate security tester's companion. It is a collection of various types of lists that are frequently used during security assessments, all in one place. SecLists helps make security testing more efficient and productive by conveniently providing all the lists a security tester might need. List types include usernames, passwords, URLs, fuzzing payloads, sensitive data patterns, web shells, and more. The tester can simply pull this repository onto a new test machine and he will have access to every type of list he needs.

SublimeText3 English version
Recommended: Win version, supports code prompts!

Safe Exam Browser
Safe Exam Browser is a secure browser environment for taking online exams securely. This software turns any computer into a secure workstation. It controls access to any utility and prevents students from using unauthorized resources.
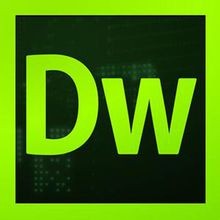
Dreamweaver CS6
Visual web development tools

Atom editor mac version download
The most popular open source editor
