This article explains the Term Frequency-Inverse Document Frequency (TF-IDF) technique, a crucial tool in Natural Language Processing (NLP) for analyzing textual data. TF-IDF surpasses the limitations of basic bag-of-words approaches by weighting terms based on their frequency within a document and their rarity across a collection of documents. This enhanced weighting improves text classification and boosts the analytical capabilities of machine learning models. We'll demonstrate how to construct a TF-IDF model from scratch in Python and perform numerical calculations.
Table of Contents
- Key Terms in TF-IDF
- Term Frequency (TF) Explained
- Document Frequency (DF) Explained
- Inverse Document Frequency (IDF) Explained
- Understanding TF-IDF
- Numerical TF-IDF Calculation
- Step 1: Calculating Term Frequency (TF)
- Step 2: Calculating Inverse Document Frequency (IDF)
- Step 3: Calculating TF-IDF
- Python Implementation using a Built-in Dataset
- Step 1: Installing Necessary Libraries
- Step 2: Importing Libraries
- Step 3: Loading the Dataset
- Step 4: Initializing
TfidfVectorizer
- Step 5: Fitting and Transforming Documents
- Step 6: Examining the TF-IDF Matrix
- Conclusion
- Frequently Asked Questions
Key Terms in TF-IDF
Before proceeding, let's define key terms:
- t: term (individual word)
- d: document (a set of words)
- N: total number of documents in the corpus
- corpus: the entire collection of documents
Term Frequency (TF) Explained
Term Frequency (TF) quantifies how often a term appears in a specific document. A higher TF indicates greater importance within that document. The formula is:
Document Frequency (DF) Explained
Document Frequency (DF) measures the number of documents within the corpus containing a particular term. Unlike TF, it counts the presence of a term, not its occurrences. The formula is:
DF(t) = Number of documents containing term t
Inverse Document Frequency (IDF) Explained
Inverse Document Frequency (IDF) assesses the informativeness of a word. While TF treats all terms equally, IDF downweights common words (like stop words) and upweights rarer terms. The formula is:
where N is the total number of documents and DF(t) is the number of documents containing term t.
Understanding TF-IDF
TF-IDF combines Term Frequency and Inverse Document Frequency to determine a term's significance within a document relative to the entire corpus. The formula is:
Numerical TF-IDF Calculation
Let's illustrate numerical TF-IDF calculation with example documents:
Documents:
- “The sky is blue.”
- “The sun is bright today.”
- “The sun in the sky is bright.”
- “We can see the shining sun, the bright sun.”
Following the steps outlined in the original text, we calculate TF, IDF, and then TF-IDF for each term in each document. (The detailed calculations are omitted here for brevity, but they mirror the original example.)
Python Implementation using a Built-in Dataset
This section demonstrates TF-IDF calculation using scikit-learn's TfidfVectorizer
and the 20 Newsgroups dataset.
Step 1: Installing Necessary Libraries
pip install scikit-learn
Step 2: Importing Libraries
import pandas as pd from sklearn.datasets import fetch_20newsgroups from sklearn.feature_extraction.text import TfidfVectorizer
Step 3: Loading the Dataset
newsgroups = fetch_20newsgroups(subset='train')
Step 4: Initializing TfidfVectorizer
vectorizer = TfidfVectorizer(stop_words='english', max_features=1000)
Step 5: Fitting and Transforming Documents
tfidf_matrix = vectorizer.fit_transform(newsgroups.data)
Step 6: Examining the TF-IDF Matrix
df_tfidf = pd.DataFrame(tfidf_matrix.toarray(), columns=vectorizer.get_feature_names_out()) df_tfidf.head()
Conclusion
Using the 20 Newsgroups dataset and TfidfVectorizer
, we efficiently transform text documents into a TF-IDF matrix. This matrix represents the importance of each term, enabling various NLP tasks like text classification and clustering. Scikit-learn's TfidfVectorizer
simplifies this process significantly.
Frequently Asked Questions
The FAQs section remains largely unchanged, addressing the logarithmic nature of IDF, scalability to large datasets, limitations of TF-IDF (ignoring word order and context), and common applications (search engines, text classification, clustering, summarization).
The above is the detailed content of Convert Text Documents to a TF-IDF Matrix with tfidfvectorizer. For more information, please follow other related articles on the PHP Chinese website!
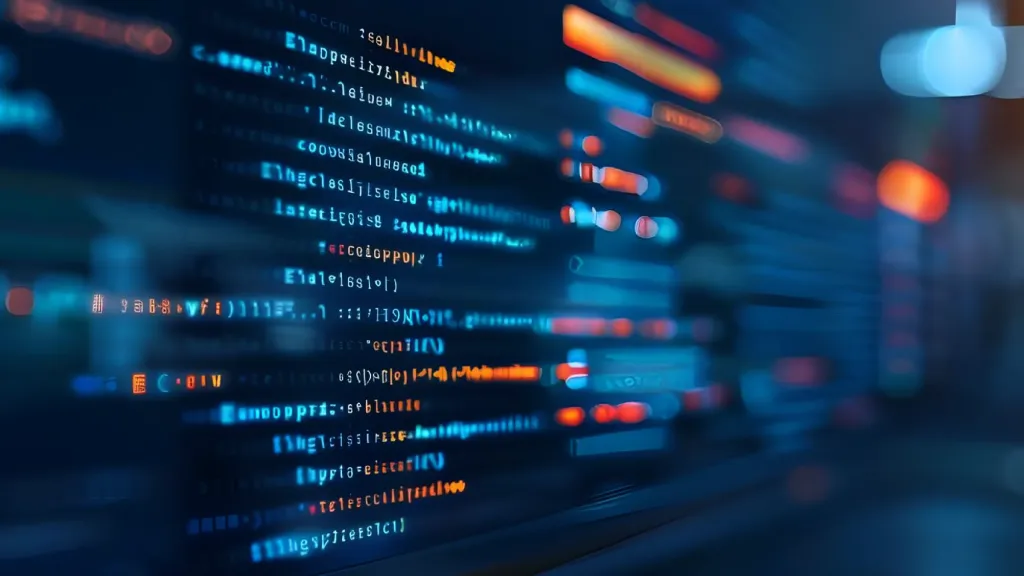
Running large language models at home with ease: LM Studio User Guide In recent years, advances in software and hardware have made it possible to run large language models (LLMs) on personal computers. LM Studio is an excellent tool to make this process easy and convenient. This article will dive into how to run LLM locally using LM Studio, covering key steps, potential challenges, and the benefits of having LLM locally. Whether you are a tech enthusiast or are curious about the latest AI technologies, this guide will provide valuable insights and practical tips. Let's get started! Overview Understand the basic requirements for running LLM locally. Set up LM Studi on your computer
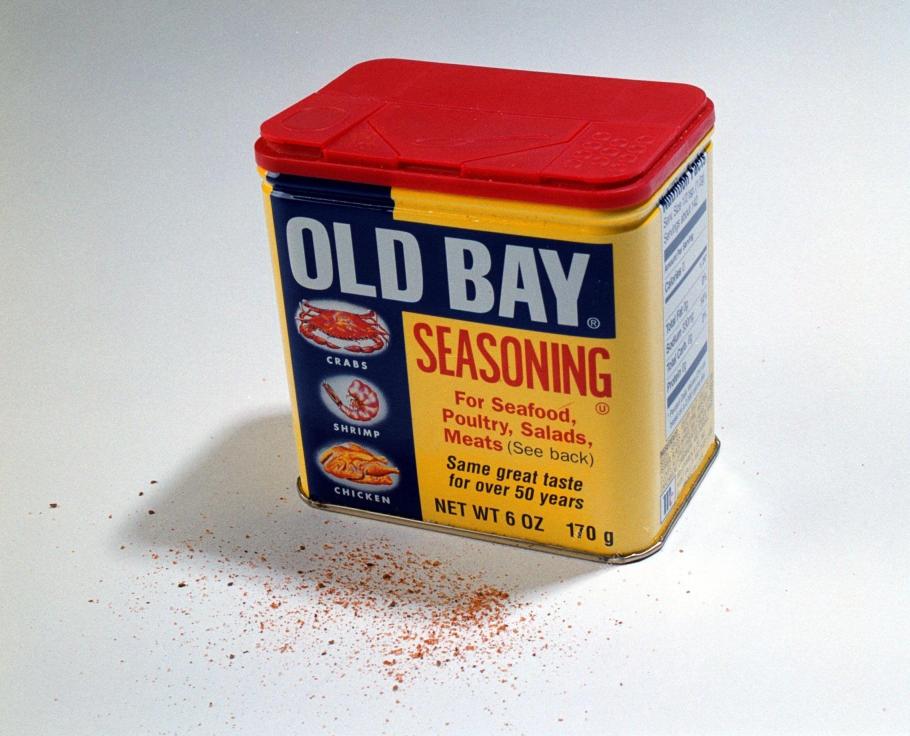
Guy Peri is McCormick’s Chief Information and Digital Officer. Though only seven months into his role, Peri is rapidly advancing a comprehensive transformation of the company’s digital capabilities. His career-long focus on data and analytics informs
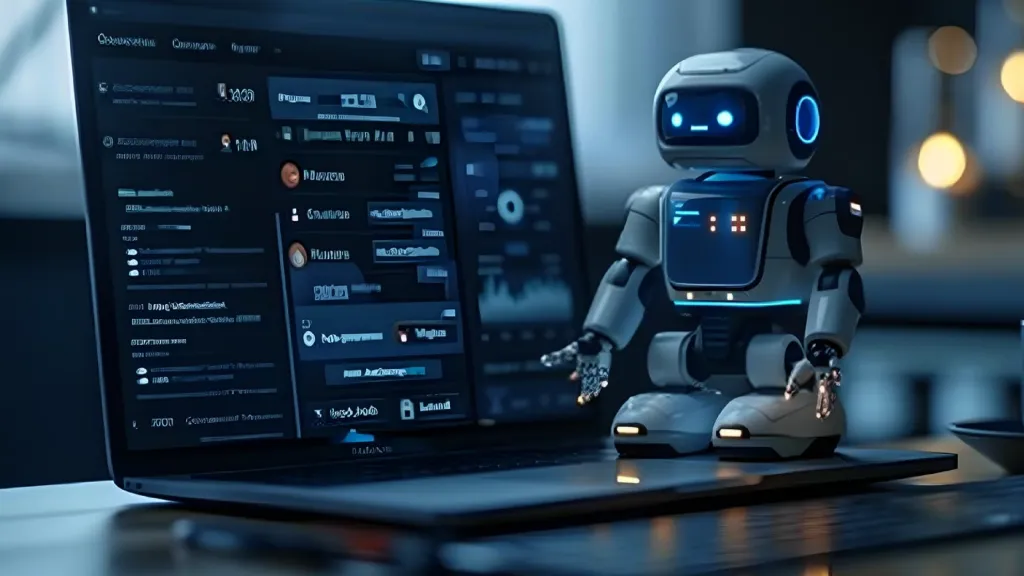
Introduction Artificial intelligence (AI) is evolving to understand not just words, but also emotions, responding with a human touch. This sophisticated interaction is crucial in the rapidly advancing field of AI and natural language processing. Th
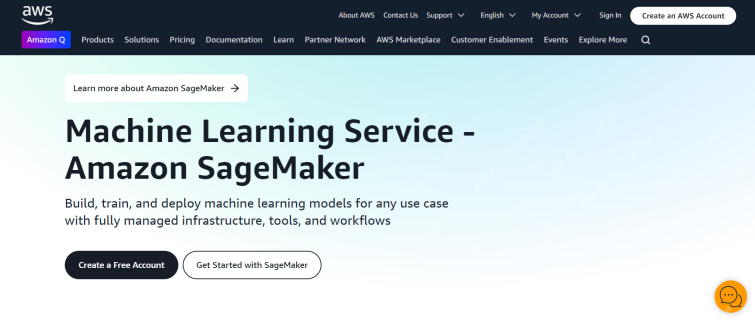
Introduction In today's data-centric world, leveraging advanced AI technologies is crucial for businesses seeking a competitive edge and enhanced efficiency. A range of powerful tools empowers data scientists, analysts, and developers to build, depl
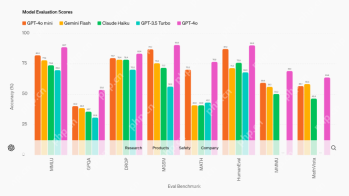
This week's AI landscape exploded with groundbreaking releases from industry giants like OpenAI, Mistral AI, NVIDIA, DeepSeek, and Hugging Face. These new models promise increased power, affordability, and accessibility, fueled by advancements in tr
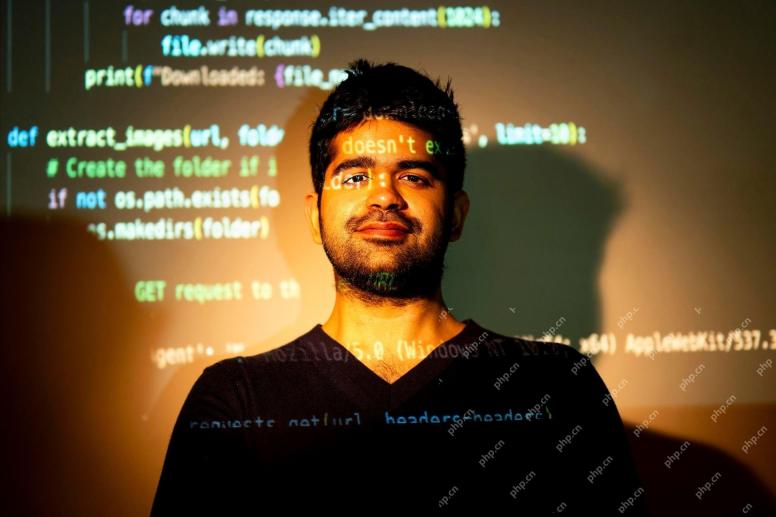
But the company’s Android app, which offers not only search capabilities but also acts as an AI assistant, is riddled with a host of security issues that could expose its users to data theft, account takeovers and impersonation attacks from malicious
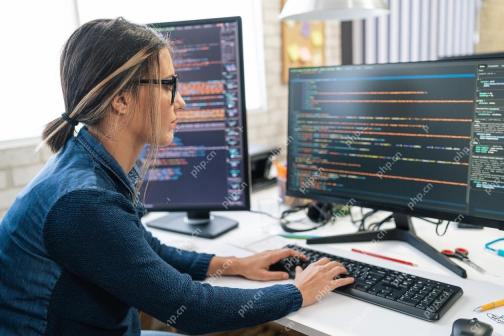
You can look at what’s happening in conferences and at trade shows. You can ask engineers what they’re doing, or consult with a CEO. Everywhere you look, things are changing at breakneck speed. Engineers, and Non-Engineers What’s the difference be
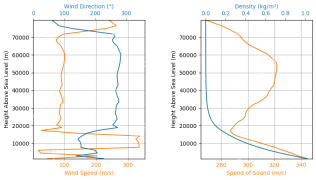
Simulate Rocket Launches with RocketPy: A Comprehensive Guide This article guides you through simulating high-power rocket launches using RocketPy, a powerful Python library. We'll cover everything from defining rocket components to analyzing simula


Hot AI Tools

Undresser.AI Undress
AI-powered app for creating realistic nude photos

AI Clothes Remover
Online AI tool for removing clothes from photos.

Undress AI Tool
Undress images for free

Clothoff.io
AI clothes remover

AI Hentai Generator
Generate AI Hentai for free.

Hot Article

Hot Tools

SecLists
SecLists is the ultimate security tester's companion. It is a collection of various types of lists that are frequently used during security assessments, all in one place. SecLists helps make security testing more efficient and productive by conveniently providing all the lists a security tester might need. List types include usernames, passwords, URLs, fuzzing payloads, sensitive data patterns, web shells, and more. The tester can simply pull this repository onto a new test machine and he will have access to every type of list he needs.
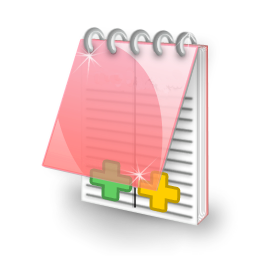
EditPlus Chinese cracked version
Small size, syntax highlighting, does not support code prompt function

Zend Studio 13.0.1
Powerful PHP integrated development environment

SublimeText3 English version
Recommended: Win version, supports code prompts!

PhpStorm Mac version
The latest (2018.2.1) professional PHP integrated development tool