Design a system for processing large log files
To design an effective system for processing large log files, a multi-tiered architecture can be implemented, incorporating various components to handle data ingestion, processing, storage, and analysis. Here’s a step-by-step breakdown of the system design:
-
Data Ingestion Layer:
- This layer is responsible for collecting logs from various sources such as servers, applications, and devices. A scalable message queue system like Apache Kafka can be utilized to efficiently buffer incoming logs. This ensures that the system can handle high volumes of data without loss.
-
Processing Layer:
- Logs collected in the data ingestion layer are then processed using a distributed computing framework like Apache Spark or Hadoop. These frameworks can perform data cleansing, normalization, and initial analysis, transforming the logs into a structured format suitable for deeper analysis.
-
Storage Layer:
- Processed data needs to be stored for future retrieval and analysis. A distributed file system like HDFS (Hadoop Distributed File System) or a NoSQL database like Apache Cassandra can be employed. These solutions offer scalability and fault tolerance, making them ideal for large data volumes.
-
Analysis Layer:
- This layer is where advanced analytics and machine learning models are applied to the data. Tools like Elasticsearch can be used for full-text search and real-time analytics, while machine learning platforms like TensorFlow or PyTorch can be integrated for predictive analysis.
-
Visualization and Reporting Layer:
- To make the processed and analyzed data actionable, a visualization tool like Kibana or Tableau can be integrated. These tools help in creating dashboards and reports that can be easily interpreted by stakeholders.
-
Security and Compliance Layer:
- Ensuring data security and compliance with regulations is crucial. Implement encryption for data at rest and in transit, along with access control mechanisms to safeguard the data.
This architecture ensures that the system can scale, perform real-time processing, and handle large volumes of log data efficiently.
What are the key features needed in a system to efficiently process large log files?
Key features necessary for efficiently processing large log files include:
-
Scalability:
- The system must be able to handle increasing volumes of log data without performance degradation. This includes horizontal scaling capabilities, where additional nodes can be added to the system to handle more data.
-
Real-Time Processing:
- Efficient processing of logs in real-time is essential for timely insights and decision-making. Stream processing capabilities should be included to analyze data as it arrives.
-
Data Parsing and Normalization:
- Log files often come in different formats and structures. The system should have capabilities to parse and normalize this data into a uniform format to facilitate analysis.
-
Distributed Processing:
- Utilizing distributed computing frameworks can help in parallelizing the data processing tasks, thereby speeding up the analysis.
-
Storage Optimization:
- Efficient storage solutions should be implemented to manage the large volumes of data generated by logs. This includes compression techniques and data tiering to store frequently accessed data in faster storage.
-
Security:
- Ensuring the logs are processed securely and in compliance with data protection regulations. Features like encryption and access control are vital.
-
Fault Tolerance and High Availability:
- The system must be designed to be fault-tolerant, ensuring that it can continue to operate even if some of its components fail. This is critical for maintaining data integrity and system reliability.
-
Analytics and Visualization:
- Integration with advanced analytics tools and visualization platforms to derive insights from the processed data and present them in an easily understandable format.
How can a system be optimized to handle the real-time analysis of large log files?
Optimizing a system for real-time analysis of large log files involves several strategies:
-
Stream Processing:
- Implementing stream processing technologies like Apache Kafka Streams or Apache Flink can enable real-time data processing. These tools can ingest and analyze data as it streams in, reducing latency.
-
In-Memory Computing:
- Use in-memory data processing frameworks like Apache Ignite or Redis to reduce data access times. In-memory computing can significantly speed up the analysis process.
-
Microservices Architecture:
- Adopting a microservices architecture can enhance the system's responsiveness. Each microservice can handle a specific aspect of log processing and analysis, allowing for better resource utilization and easier scaling.
-
Edge Computing:
- For distributed environments, edge computing can be used to preprocess logs at the source before sending them to the central system. This reduces the amount of data that needs to be transferred and processed centrally.
-
Optimized Data Models:
- Designing efficient data models that facilitate quick queries and analysis can improve real-time processing. This includes using appropriate indexing and data structures.
-
Asynchronous Processing:
- Implementing asynchronous data processing can help manage real-time analysis more effectively. Non-blocking operations can be used to process data without waiting for previous operations to complete.
-
Load Balancing:
- Distribute the incoming logs across multiple nodes using load balancing techniques to ensure even distribution of work and prevent bottlenecks.
-
Caching:
- Use caching mechanisms to store frequently accessed data or intermediate results. This can significantly reduce the time needed for data retrieval and processing.
By integrating these strategies, a system can be optimized to perform real-time analysis of large log files effectively.
What scalability measures should be implemented in a system designed for processing large log files?
To ensure a system designed for processing large log files can scale effectively, the following measures should be implemented:
-
Horizontal Scaling:
- The system should support the addition of more nodes to handle increased data volume. This can be achieved by designing components that can be easily replicated and distributed across multiple machines.
-
Load Balancing:
- Implement load balancing mechanisms to evenly distribute the workload across nodes. This prevents any single node from becoming a bottleneck and ensures efficient resource utilization.
-
Data Partitioning:
- Partitioning data across different nodes can improve performance and scalability. Techniques like sharding can be used to distribute data evenly, reducing the load on any single node.
-
Elastic Resources:
- Utilize cloud technologies that allow for elastic scaling of resources. Cloud providers like AWS or Google Cloud can dynamically allocate additional resources based on demand.
-
Stateless Design:
- Designing the system to be stateless where possible can facilitate easier scaling. Stateless components can be replicated without concern for managing state across multiple instances.
-
Automated Scaling Policies:
- Implement automated scaling policies that can trigger the addition or removal of resources based on predefined metrics such as CPU usage, memory consumption, or data throughput.
-
Efficient Data Storage:
- Use scalable storage solutions like distributed file systems or NoSQL databases that can grow with the data volume. Implement data lifecycle management to archive or delete old logs, freeing up space for new data.
-
Optimized Network Architecture:
- Ensure the network architecture supports high throughput and low latency. This includes using content delivery networks (CDNs) for faster data transfer and reducing network congestion.
-
Monitoring and Performance Tuning:
- Continuous monitoring of system performance and regular tuning can help identify and address scalability issues before they impact the system. Tools like Prometheus or Grafana can be used for monitoring.
By implementing these scalability measures, a system designed for processing large log files can effectively handle growing data volumes and maintain performance.
The above is the detailed content of Design a system for processing large log files.. For more information, please follow other related articles on the PHP Chinese website!
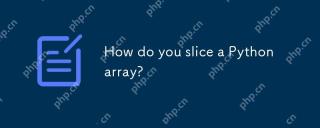
The basic syntax for Python list slicing is list[start:stop:step]. 1.start is the first element index included, 2.stop is the first element index excluded, and 3.step determines the step size between elements. Slices are not only used to extract data, but also to modify and invert lists.
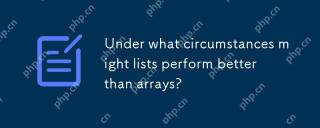
Listsoutperformarraysin:1)dynamicsizingandfrequentinsertions/deletions,2)storingheterogeneousdata,and3)memoryefficiencyforsparsedata,butmayhaveslightperformancecostsincertainoperations.
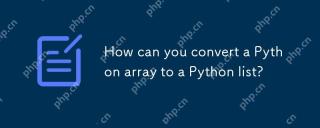
ToconvertaPythonarraytoalist,usethelist()constructororageneratorexpression.1)Importthearraymoduleandcreateanarray.2)Uselist(arr)or[xforxinarr]toconvertittoalist,consideringperformanceandmemoryefficiencyforlargedatasets.
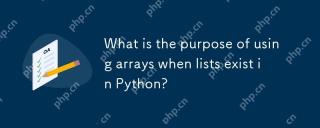
ChoosearraysoverlistsinPythonforbetterperformanceandmemoryefficiencyinspecificscenarios.1)Largenumericaldatasets:Arraysreducememoryusage.2)Performance-criticaloperations:Arraysofferspeedboostsfortaskslikeappendingorsearching.3)Typesafety:Arraysenforc
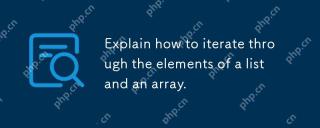
In Python, you can use for loops, enumerate and list comprehensions to traverse lists; in Java, you can use traditional for loops and enhanced for loops to traverse arrays. 1. Python list traversal methods include: for loop, enumerate and list comprehension. 2. Java array traversal methods include: traditional for loop and enhanced for loop.
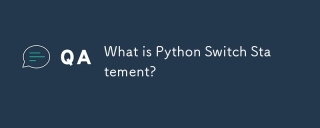
The article discusses Python's new "match" statement introduced in version 3.10, which serves as an equivalent to switch statements in other languages. It enhances code readability and offers performance benefits over traditional if-elif-el
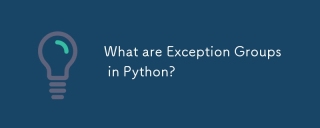
Exception Groups in Python 3.11 allow handling multiple exceptions simultaneously, improving error management in concurrent scenarios and complex operations.
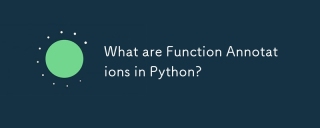
Function annotations in Python add metadata to functions for type checking, documentation, and IDE support. They enhance code readability, maintenance, and are crucial in API development, data science, and library creation.


Hot AI Tools

Undresser.AI Undress
AI-powered app for creating realistic nude photos

AI Clothes Remover
Online AI tool for removing clothes from photos.

Undress AI Tool
Undress images for free

Clothoff.io
AI clothes remover

Video Face Swap
Swap faces in any video effortlessly with our completely free AI face swap tool!

Hot Article

Hot Tools
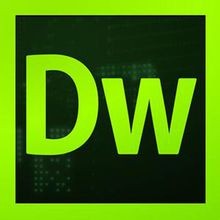
Dreamweaver CS6
Visual web development tools
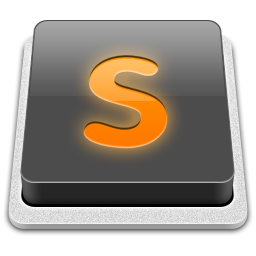
SublimeText3 Mac version
God-level code editing software (SublimeText3)
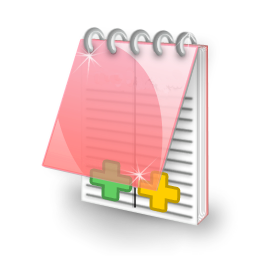
EditPlus Chinese cracked version
Small size, syntax highlighting, does not support code prompt function
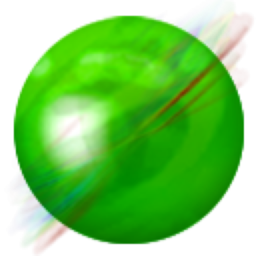
ZendStudio 13.5.1 Mac
Powerful PHP integrated development environment

Notepad++7.3.1
Easy-to-use and free code editor
