


Implement a function to find the longest common subsequence of two strings.
To implement a function that finds the longest common subsequence (LCS) of two strings, we'll use dynamic programming, which is the most efficient approach for this problem. Here is a step-by-step implementation in Python:
def longest_common_subsequence(str1, str2): m, n = len(str1), len(str2) # Create a table to store results of subproblems dp = [[0] * (n 1) for _ in range(m 1)] # Build the dp table for i in range(1, m 1): for j in range(1, n 1): if str1[i-1] == str2[j-1]: dp[i][j] = dp[i-1][j-1] 1 else: dp[i][j] = max(dp[i-1][j], dp[i][j-1]) # The last cell contains length of LCS return dp[m][n] # Test the function str1 = "AGGTAB" str2 = "GXTXAYB" print("Length of LCS is", longest_common_subsequence(str1, str2)) # Output: Length of LCS is 4
This function uses a 2D dynamic programming table to efficiently compute the length of the LCS between str1
and str2
. The time complexity is O(mn), and the space complexity is O(mn), where m and n are the lengths of the input strings.
What are the key algorithms used to solve the longest common subsequence problem?
The key algorithms used to solve the longest common subsequence problem are:
-
Dynamic Programming: This is the most commonly used and efficient method. It involves creating a table to store the results of subproblems and building the solution iteratively. The basic idea is to fill a matrix where
dp[i][j]
represents the length of the LCS of the substringsstr1[0..i-1]
andstr2[0..j-1]
. - Recursion: A naive approach to the LCS problem is through recursion, but it's inefficient due to repeated computation of the same subproblems. The recursive approach follows the principle of breaking down the problem into smaller subproblems, but without storing intermediate results, it results in exponential time complexity.
- Memoization: This is an optimization over the recursive approach, where the results of subproblems are stored to avoid redundant calculations. Memoization effectively turns the recursive solution into a dynamic programming solution, reducing the time complexity to polynomial.
- Backtracking: While not typically used alone for solving the LCS problem due to its inefficiency, backtracking can be used to actually reconstruct the LCS once its length is known through dynamic programming or memoization.
How can the efficiency of the longest common subsequence function be improved?
The efficiency of the longest common subsequence function can be improved in several ways:
-
Space Optimization: The original implementation uses O(m*n) space, but it is possible to reduce the space complexity to O(n) by only keeping track of two rows of the dynamic programming table at any given time.
def optimized_lcs(str1, str2): m, n = len(str1), len(str2) prev = [0] * (n 1) curr = [0] * (n 1) for i in range(1, m 1): for j in range(1, n 1): if str1[i-1] == str2[j-1]: curr[j] = prev[j-1] 1 else: curr[j] = max(curr[j-1], prev[j]) prev, curr = curr, prev # Swap the rows return prev[n]
- Using Hirschberg's Algorithm: If we need to find the actual LCS rather than just its length, Hirschberg's algorithm can be used to find the LCS in O(m*n) time and O(min(m,n)) space, which is more space-efficient than the traditional dynamic programming approach.
- Parallelization: The computation of the dynamic programming table can be parallelized to some extent, particularly if you're working with large strings, by dividing the work among multiple processors or threads.
- Specialized Algorithms: For specific types of strings, more specialized algorithms might be more efficient, for example, when dealing with DNA sequences, certain bioinformatics algorithms optimized for these inputs could be used.
What are common applications of finding the longest common subsequence in real-world scenarios?
Finding the longest common subsequence is a versatile algorithm used in various real-world applications, including:
- Bioinformatics: In genetics and molecular biology, LCS is used to compare DNA sequences to find similarities and differences. For example, it can help in aligning genetic sequences to identify mutations or similarities in different species.
- Text Comparison and Version Control: LCS is fundamental in tools used for file comparison, such as diff tools in version control systems like Git. It helps in identifying changes and merging different versions of source code or documents.
- Plagiarism Detection: By finding the LCS between two documents, it's possible to identify the longest common segments that might indicate plagiarism.
- Data Compression: In data compression algorithms, LCS can be used to identify redundant data sequences that can be represented more efficiently.
- Speech Recognition: LCS can be employed to align and compare spoken word sequences, which is useful in improving the accuracy of speech-to-text conversion.
- Natural Language Processing: LCS is used in NLP tasks such as text similarity measurement, which can be applied to search engine optimization, sentiment analysis, and machine translation.
These applications leverage the power of LCS to solve complex problems by efficiently identifying similarities in sequences, thereby providing valuable insights and facilitating advanced processing techniques.
The above is the detailed content of Implement a function to find the longest common subsequence of two strings.. For more information, please follow other related articles on the PHP Chinese website!
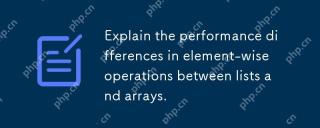
Arraysarebetterforelement-wiseoperationsduetofasteraccessandoptimizedimplementations.1)Arrayshavecontiguousmemoryfordirectaccess,enhancingperformance.2)Listsareflexiblebutslowerduetopotentialdynamicresizing.3)Forlargedatasets,arrays,especiallywithlib
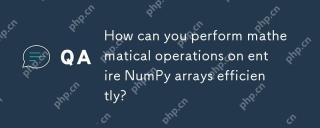
Mathematical operations of the entire array in NumPy can be efficiently implemented through vectorized operations. 1) Use simple operators such as addition (arr 2) to perform operations on arrays. 2) NumPy uses the underlying C language library, which improves the computing speed. 3) You can perform complex operations such as multiplication, division, and exponents. 4) Pay attention to broadcast operations to ensure that the array shape is compatible. 5) Using NumPy functions such as np.sum() can significantly improve performance.
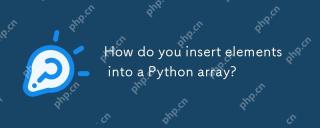
In Python, there are two main methods for inserting elements into a list: 1) Using the insert(index, value) method, you can insert elements at the specified index, but inserting at the beginning of a large list is inefficient; 2) Using the append(value) method, add elements at the end of the list, which is highly efficient. For large lists, it is recommended to use append() or consider using deque or NumPy arrays to optimize performance.
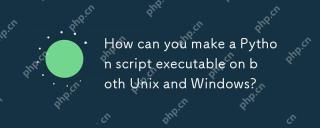
TomakeaPythonscriptexecutableonbothUnixandWindows:1)Addashebangline(#!/usr/bin/envpython3)andusechmod xtomakeitexecutableonUnix.2)OnWindows,ensurePythonisinstalledandassociatedwith.pyfiles,oruseabatchfile(run.bat)torunthescript.
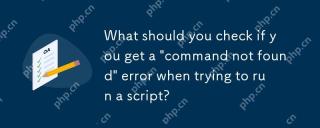
When encountering a "commandnotfound" error, the following points should be checked: 1. Confirm that the script exists and the path is correct; 2. Check file permissions and use chmod to add execution permissions if necessary; 3. Make sure the script interpreter is installed and in PATH; 4. Verify that the shebang line at the beginning of the script is correct. Doing so can effectively solve the script operation problem and ensure the coding process is smooth.
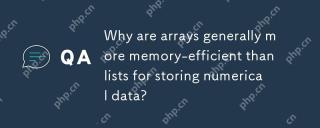
Arraysaregenerallymorememory-efficientthanlistsforstoringnumericaldataduetotheirfixed-sizenatureanddirectmemoryaccess.1)Arraysstoreelementsinacontiguousblock,reducingoverheadfrompointersormetadata.2)Lists,oftenimplementedasdynamicarraysorlinkedstruct
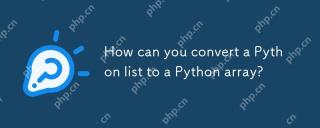
ToconvertaPythonlisttoanarray,usethearraymodule:1)Importthearraymodule,2)Createalist,3)Usearray(typecode,list)toconvertit,specifyingthetypecodelike'i'forintegers.Thisconversionoptimizesmemoryusageforhomogeneousdata,enhancingperformanceinnumericalcomp
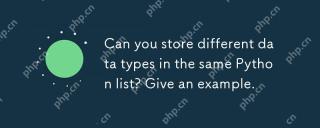
Python lists can store different types of data. The example list contains integers, strings, floating point numbers, booleans, nested lists, and dictionaries. List flexibility is valuable in data processing and prototyping, but it needs to be used with caution to ensure the readability and maintainability of the code.


Hot AI Tools

Undresser.AI Undress
AI-powered app for creating realistic nude photos

AI Clothes Remover
Online AI tool for removing clothes from photos.

Undress AI Tool
Undress images for free

Clothoff.io
AI clothes remover

Video Face Swap
Swap faces in any video effortlessly with our completely free AI face swap tool!

Hot Article

Hot Tools

Notepad++7.3.1
Easy-to-use and free code editor
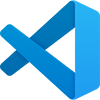
VSCode Windows 64-bit Download
A free and powerful IDE editor launched by Microsoft

Zend Studio 13.0.1
Powerful PHP integrated development environment

SecLists
SecLists is the ultimate security tester's companion. It is a collection of various types of lists that are frequently used during security assessments, all in one place. SecLists helps make security testing more efficient and productive by conveniently providing all the lists a security tester might need. List types include usernames, passwords, URLs, fuzzing payloads, sensitive data patterns, web shells, and more. The tester can simply pull this repository onto a new test machine and he will have access to every type of list he needs.
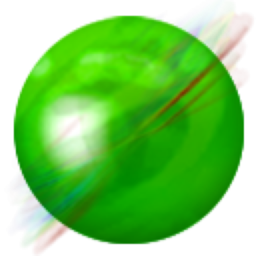
ZendStudio 13.5.1 Mac
Powerful PHP integrated development environment
