


How do you write unit tests in Python using the unittest framework?
Writing unit tests in Python using the unittest
framework involves several steps. Below is a detailed guide to creating and running unit tests:
-
Import the unittest Module: The first step is to import the
unittest
module, which provides the framework for writing and running tests.import unittest
-
Define a Test Class: Your tests will be grouped into classes that inherit from
unittest.TestCase
. This class will contain methods that define individual tests.class TestExample(unittest.TestCase):
-
Write Test Methods: Inside the
TestExample
class, you can write methods that start with the wordtest
. These methods will run as individual tests.def test_example(self): self.assertEqual(1 1, 2)
-
Set Up and Tear Down: If your tests require any setup or cleanup, you can use
setUp
andtearDown
methods.setUp
runs before each test method, andtearDown
runs after.def setUp(self): # Code here will run before every test pass def tearDown(self): # Code here will run after every test pass
-
Run the Tests: To run the tests, you can either run the script directly if it contains the tests, or use a test runner. The simplest way is to add the following code at the end of your script:
if __name__ == '__main__': unittest.main()
When you run the script, unittest
will automatically discover and execute all methods starting with test
within classes that inherit from unittest.TestCase
.
What are the best practices for structuring unit tests with Python's unittest?
Adhering to best practices when structuring unit tests in Python's unittest
framework helps ensure tests are maintainable, readable, and effective. Here are key practices to follow:
-
Test Naming Conventions: Use clear, descriptive names for your test classes and methods. For example,
TestCalculator
for a class andtest_addition
for a method. This helps quickly understand what each test is intended to verify. -
Arrange-Act-Assert Pattern: Structure your test methods using the Arrange-Act-Assert pattern:
- Arrange: Set up the conditions for the test.
- Act: Perform the action you want to test.
-
Assert: Verify the result.
def test_addition(self): # Arrange calc = Calculator() # Act result = calc.add(2, 3) # Assert self.assertEqual(result, 5)
-
Isolate Tests: Ensure that each test is independent. Use
setUp
andtearDown
methods to manage test fixtures, ensuring each test starts with a clean slate. -
Use setUp and tearDown Wisely: Use
setUp
to initialize objects andtearDown
to clean up resources if necessary. Avoid using them for actions that can be done inline with tests unless you find significant code duplication. - Group Related Tests: Group similar tests into the same test class to keep related functionality together, making your test suite more organized and easier to understand.
-
Use Descriptive Error Messages: When using assertions like
assertEqual
, you can add a custom message to clarify what went wrong, which is particularly useful when debugging failing tests.self.assertEqual(result, 5, "The addition of 2 and 3 should be 5")
How can you use assertions effectively in Python unittest to validate test results?
Assertions are crucial in unittest
to check if the output of your code meets the expected results. Here's how to use them effectively:
-
Choose the Right Assertion Method:
unittest
provides several assertion methods, each designed for specific comparisons:-
assertEqual(a, b)
: Checks ifa == b
. -
assertNotEqual(a, b)
: Checks ifa != b
. -
assertTrue(x)
: Checks ifx
is true. -
assertFalse(x)
: Checks ifx
is false. -
assertIs(a, b)
: Checks ifa
isb
(object identity). -
assertIsNot(a, b)
: Checks ifa
is notb
. -
assertIn(a, b)
: Checks ifa
is inb
. -
assertNotIn(a, b)
: Checks ifa
is not inb
.
Choose the assertion that best fits the test condition.
-
-
Use Custom Messages: For complex tests, it's helpful to provide a custom message to explain why the assertion failed.
self.assertEqual(result, 5, "Expected 5 but got {}".format(result))
-
Test for Edge Cases: Use assertions to validate not only the typical case but also edge cases and error conditions. For example, test for boundary conditions, invalid inputs, and expected exceptions.
def test_division_by_zero(self): with self.assertRaises(ZeroDivisionError): Calculator().divide(10, 0)
- Avoid Over-Assertion: Don’t overdo assertions in a single test method. If you find yourself asserting multiple, unrelated things, it might be a sign that you should split the test into multiple methods.
-
Use Context Managers for Expected Exceptions: If you're expecting a specific exception, use the
assertRaises
context manager.with self.assertRaises(ValueError): Calculator().sqrt(-1)
What are common pitfalls to avoid when writing unit tests in Python using the unittest framework?
When writing unit tests with unittest
, it's helpful to be aware of common pitfalls to avoid in order to maintain high-quality tests:
- Testing Too Much in One Test: Avoid overloading a single test method with multiple assertions that test different functionalities. It's better to write separate tests for each piece of functionality.
- Not Testing Edge Cases: Neglecting to test for edge cases, such as empty inputs, maximum and minimum values, or error conditions, can leave your code vulnerable. Always think about the boundaries and unexpected inputs.
-
Overusing setUp and tearDown: While
setUp
andtearDown
are useful, overusing them can lead to test dependencies and slower tests. Use them only when necessary to set up test fixtures or clean up resources. - Ignoring Test Isolation: Each test should be independent. Sharing state between tests can lead to unpredictable results and make it hard to diagnose failures.
- Writing Tests After Code: Writing tests after the code can lead to tests that simply confirm the code works as-is rather than ensuring it behaves correctly under all conditions. Prefer writing tests before the code (Test-Driven Development, TDD).
- Not Updating Tests with Code Changes: As your code evolves, your tests need to evolve too. Failing to update tests to reflect changes in your code can lead to false negatives or false positives.
- Neglecting to Use Mocks and Stubs: For tests that depend on external resources or complex objects, not using mocks or stubs can make tests slow and brittle. Utilize mocking libraries to isolate dependencies.
- Writing Too Few Tests: Under-testing can leave critical parts of your code untested. Aim for a high coverage, especially for complex logic and edge cases.
By avoiding these pitfalls, you can ensure that your unit tests are robust, maintainable, and effectively validate the functionality of your code.
The above is the detailed content of How do you write unit tests in Python using the unittest framework?. For more information, please follow other related articles on the PHP Chinese website!
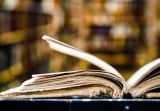
This tutorial demonstrates how to use Python to process the statistical concept of Zipf's law and demonstrates the efficiency of Python's reading and sorting large text files when processing the law. You may be wondering what the term Zipf distribution means. To understand this term, we first need to define Zipf's law. Don't worry, I'll try to simplify the instructions. Zipf's Law Zipf's law simply means: in a large natural language corpus, the most frequently occurring words appear about twice as frequently as the second frequent words, three times as the third frequent words, four times as the fourth frequent words, and so on. Let's look at an example. If you look at the Brown corpus in American English, you will notice that the most frequent word is "th
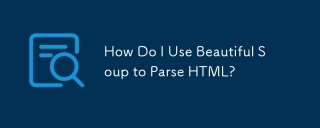
This article explains how to use Beautiful Soup, a Python library, to parse HTML. It details common methods like find(), find_all(), select(), and get_text() for data extraction, handling of diverse HTML structures and errors, and alternatives (Sel
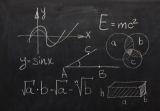
Python's statistics module provides powerful data statistical analysis capabilities to help us quickly understand the overall characteristics of data, such as biostatistics and business analysis. Instead of looking at data points one by one, just look at statistics such as mean or variance to discover trends and features in the original data that may be ignored, and compare large datasets more easily and effectively. This tutorial will explain how to calculate the mean and measure the degree of dispersion of the dataset. Unless otherwise stated, all functions in this module support the calculation of the mean() function instead of simply summing the average. Floating point numbers can also be used. import random import statistics from fracti
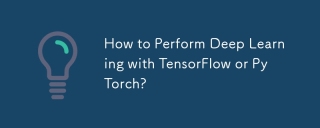
This article compares TensorFlow and PyTorch for deep learning. It details the steps involved: data preparation, model building, training, evaluation, and deployment. Key differences between the frameworks, particularly regarding computational grap
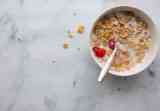
Serialization and deserialization of Python objects are key aspects of any non-trivial program. If you save something to a Python file, you do object serialization and deserialization if you read the configuration file, or if you respond to an HTTP request. In a sense, serialization and deserialization are the most boring things in the world. Who cares about all these formats and protocols? You want to persist or stream some Python objects and retrieve them in full at a later time. This is a great way to see the world on a conceptual level. However, on a practical level, the serialization scheme, format or protocol you choose may determine the speed, security, freedom of maintenance status, and other aspects of the program
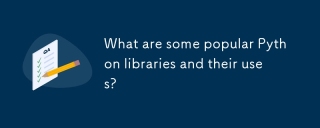
The article discusses popular Python libraries like NumPy, Pandas, Matplotlib, Scikit-learn, TensorFlow, Django, Flask, and Requests, detailing their uses in scientific computing, data analysis, visualization, machine learning, web development, and H
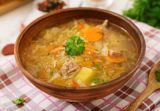
This tutorial builds upon the previous introduction to Beautiful Soup, focusing on DOM manipulation beyond simple tree navigation. We'll explore efficient search methods and techniques for modifying HTML structure. One common DOM search method is ex
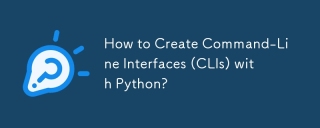
This article guides Python developers on building command-line interfaces (CLIs). It details using libraries like typer, click, and argparse, emphasizing input/output handling, and promoting user-friendly design patterns for improved CLI usability.


Hot AI Tools

Undresser.AI Undress
AI-powered app for creating realistic nude photos

AI Clothes Remover
Online AI tool for removing clothes from photos.

Undress AI Tool
Undress images for free

Clothoff.io
AI clothes remover

AI Hentai Generator
Generate AI Hentai for free.

Hot Article

Hot Tools

SublimeText3 Linux new version
SublimeText3 Linux latest version
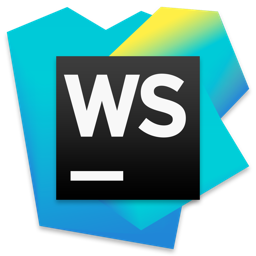
WebStorm Mac version
Useful JavaScript development tools
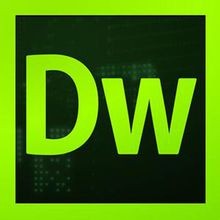
Dreamweaver CS6
Visual web development tools

SAP NetWeaver Server Adapter for Eclipse
Integrate Eclipse with SAP NetWeaver application server.

SublimeText3 Chinese version
Chinese version, very easy to use
