


Explain the concept of context switching in concurrent programming.
Context switching in concurrent programming refers to the process by which a computer operating system or a runtime environment switches the CPU's attention from one task, thread, or process to another. In concurrent systems, multiple tasks can be executed seemingly simultaneously by rapidly switching the CPU's focus among them.
When a context switch occurs, the state of the currently executing task (including its CPU registers, program counter, and memory management information) is saved, and the state of the next task to be executed is loaded. This allows the CPU to resume the execution of the new task from where it was previously paused. Context switching is essential in multitasking environments, enabling the system to handle multiple tasks efficiently and provide a responsive user experience.
However, context switching involves overhead because it requires time to save and restore the state of tasks. This overhead becomes particularly noticeable in systems with high concurrency and frequent task switching.
What are the performance impacts of frequent context switching in a system?
Frequent context switching can significantly impact system performance in several ways:
- Increased Overhead: Each context switch consumes time to save and restore task states, which can lead to reduced CPU efficiency. In systems where tasks are switched frequently, a substantial portion of CPU time may be spent on context switching rather than on actual computation.
- Cache Inefficiency: When the CPU switches context, the data in the CPU cache, which is optimized for the previous task, may no longer be relevant to the new task. This leads to cache thrashing, where the CPU spends more time reloading the cache with data relevant to the new task, further reducing performance.
- Increased Memory Usage: Context switching requires memory to store the state of each task. In systems with high concurrency, this can lead to increased memory consumption, which might cause memory pressure and slower performance due to increased paging and swapping.
- Reduced Throughput: Due to the time spent on context switching and the inefficiencies mentioned above, the overall throughput of the system, or the amount of work completed in a given time, can decrease.
- Increased Latency: Frequent context switching can also increase the latency of individual tasks, as each task may spend more time waiting for its turn to execute on the CPU.
Understanding these impacts is crucial for developers designing concurrent systems, as it helps them make informed decisions about task scheduling and resource management.
How can developers minimize the overhead of context switching in their applications?
To minimize the overhead of context switching, developers can employ several strategies:
- Minimize Task Switching: Where possible, reduce the frequency of context switches by designing tasks that execute for longer periods before yielding control. This can be achieved by grouping related operations into larger tasks.
- Use Efficient Scheduling Algorithms: Implement scheduling algorithms that reduce unnecessary context switches. For example, using a priority-based scheduler can ensure that high-priority tasks are less likely to be preempted by lower-priority ones.
- Optimize Thread Pool Sizes: In applications using thread pools, carefully tune the size of the pool to balance between resource utilization and context switching. An excessively large pool can lead to frequent context switches, while a small pool may underutilize CPU resources.
- Leverage Asynchronous Programming: Use asynchronous programming techniques, such as non-blocking I/O, to allow tasks to yield control without causing a context switch. This can improve performance in I/O-bound applications.
- Cache-Friendly Design: Design data structures and algorithms to maximize cache utilization, reducing the performance hit from cache thrashing during context switches.
- Affinity and Binding: Use CPU affinity and thread binding to keep tasks running on the same CPU core, minimizing the overhead of context switching and improving cache performance.
- Profiling and Optimization: Use profiling tools to identify hotspots and bottlenecks related to context switching, and optimize accordingly. This might involve restructuring code to minimize the number of context switches or to improve the efficiency of task execution.
Implementing these strategies can help developers reduce the performance impact of context switching and enhance the overall efficiency of their concurrent applications.
What tools or techniques can be used to monitor and analyze context switching in concurrent programs?
To monitor and analyze context switching in concurrent programs, developers can use various tools and techniques:
-
Operating System Profiling Tools:
-
Linux: Tools like
perf
andtop
can provide insights into context switching.perf
can record and analyze context switch events, whiletop
shows the number of context switches over time. - Windows: The Windows Performance Monitor and Resource Monitor can display context switch rates and help identify performance bottlenecks.
-
Linux: Tools like
-
Application Profiling Tools:
- Visual Studio: Offers profiling capabilities that include monitoring context switches and thread execution patterns.
- Java VisualVM: A tool for monitoring and troubleshooting Java applications, which can display thread activity and context switch information.
- Intel VTune Amplifier: A powerful profiling tool that can analyze context switching and provide detailed performance metrics.
-
Tracing and Logging:
- Implementing logging within the application to record when context switches occur can help in analyzing the frequency and impact of these switches. Tools like DTrace on Solaris/Linux or ETW (Event Tracing for Windows) can be used for system-level tracing.
-
Custom Monitoring:
- Developers can create custom monitoring solutions by adding instrumentation to their code to track context switches. This might involve using timers or counters to measure the frequency and duration of context switches.
-
Analytical Tools:
- GDB (GNU Debugger): Can be used to step through a program and observe context switches, particularly useful for debugging concurrent applications.
- Thread Sanitizer: A tool for detecting data races and other concurrency issues, which can also provide insights into context switching behavior.
By using these tools and techniques, developers can gain a deeper understanding of context switching in their applications, allowing them to identify and address performance issues related to concurrency.
The above is the detailed content of Explain the concept of context switching in concurrent programming.. For more information, please follow other related articles on the PHP Chinese website!
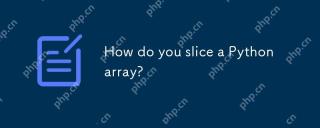
The basic syntax for Python list slicing is list[start:stop:step]. 1.start is the first element index included, 2.stop is the first element index excluded, and 3.step determines the step size between elements. Slices are not only used to extract data, but also to modify and invert lists.
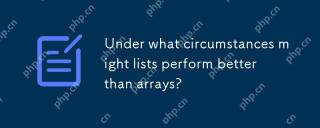
Listsoutperformarraysin:1)dynamicsizingandfrequentinsertions/deletions,2)storingheterogeneousdata,and3)memoryefficiencyforsparsedata,butmayhaveslightperformancecostsincertainoperations.
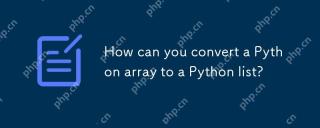
ToconvertaPythonarraytoalist,usethelist()constructororageneratorexpression.1)Importthearraymoduleandcreateanarray.2)Uselist(arr)or[xforxinarr]toconvertittoalist,consideringperformanceandmemoryefficiencyforlargedatasets.
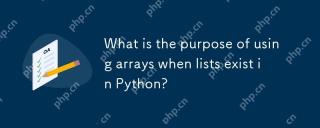
ChoosearraysoverlistsinPythonforbetterperformanceandmemoryefficiencyinspecificscenarios.1)Largenumericaldatasets:Arraysreducememoryusage.2)Performance-criticaloperations:Arraysofferspeedboostsfortaskslikeappendingorsearching.3)Typesafety:Arraysenforc
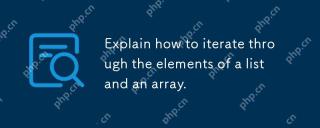
In Python, you can use for loops, enumerate and list comprehensions to traverse lists; in Java, you can use traditional for loops and enhanced for loops to traverse arrays. 1. Python list traversal methods include: for loop, enumerate and list comprehension. 2. Java array traversal methods include: traditional for loop and enhanced for loop.
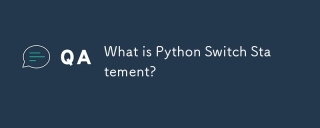
The article discusses Python's new "match" statement introduced in version 3.10, which serves as an equivalent to switch statements in other languages. It enhances code readability and offers performance benefits over traditional if-elif-el
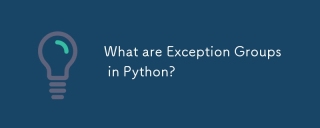
Exception Groups in Python 3.11 allow handling multiple exceptions simultaneously, improving error management in concurrent scenarios and complex operations.
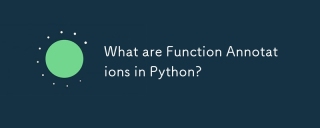
Function annotations in Python add metadata to functions for type checking, documentation, and IDE support. They enhance code readability, maintenance, and are crucial in API development, data science, and library creation.


Hot AI Tools

Undresser.AI Undress
AI-powered app for creating realistic nude photos

AI Clothes Remover
Online AI tool for removing clothes from photos.

Undress AI Tool
Undress images for free

Clothoff.io
AI clothes remover

Video Face Swap
Swap faces in any video effortlessly with our completely free AI face swap tool!

Hot Article

Hot Tools

SublimeText3 Chinese version
Chinese version, very easy to use

Zend Studio 13.0.1
Powerful PHP integrated development environment

PhpStorm Mac version
The latest (2018.2.1) professional PHP integrated development tool
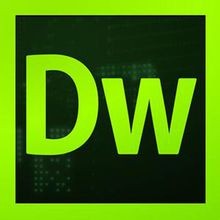
Dreamweaver CS6
Visual web development tools

SecLists
SecLists is the ultimate security tester's companion. It is a collection of various types of lists that are frequently used during security assessments, all in one place. SecLists helps make security testing more efficient and productive by conveniently providing all the lists a security tester might need. List types include usernames, passwords, URLs, fuzzing payloads, sensitive data patterns, web shells, and more. The tester can simply pull this repository onto a new test machine and he will have access to every type of list he needs.
