


What are the different types of indexes in MongoDB (single, compound, multi-key, geospatial)?
This article explains MongoDB's index types: single, compound, multi-key, and geospatial. It details how to choose the right index based on query patterns and field cardinality, highlighting performance benefits and limitations of each type, includi
What are the different types of indexes in MongoDB (single, compound, multi-key, geospatial)?
MongoDB offers several index types to optimize query performance. Understanding these types is crucial for efficient database operations. Let's break down the common ones:
-
Single Indexes: These are the simplest type of index. They index a single field in a collection. For example, an index on the
name
field would allow for quick lookups of documents based on their name. This is ideal for queries that frequently filter on a single field. The query optimizer can use this index to quickly locate relevant documents without scanning the entire collection. -
Compound Indexes: These indexes span multiple fields. For example, an index on
{ age: 1, city: 1 }
would index documents based on a combination of age and city. The order of fields in a compound index is significant. The query optimizer will use this index efficiently if the query filters on the fields in the same order and direction (ascending or descending) as defined in the index. Queries filtering only on the leading fields (e.g.,age
) will also benefit from this index. -
Multi-key Indexes: These indexes allow indexing of arrays. If a field contains an array of values (e.g.,
tags: ["programming", "mongodb"]
), a multi-key index on that field allows for efficient queries that search for documents containing specific elements within the array. For instance, finding documents with the tag "mongodb" would be significantly faster with a multi-key index. - Geospatial Indexes: These indexes are designed specifically for geospatial data, allowing efficient querying of locations based on proximity, distance, and geographic shapes. They utilize special data types like GeoJSON to represent points, lines, and polygons. Common geospatial queries include finding documents within a certain radius of a given point or intersecting a given polygon. MongoDB provides two main types of geospatial indexes: 2dsphere (for spherical coordinates, suitable for global data) and 2d (for planar coordinates, suitable for smaller areas).
How do I choose the right index type for my MongoDB queries?
Selecting the appropriate index type hinges on understanding your query patterns. Analyze your application's common queries to identify the fields frequently used in filtering and sorting operations.
-
Analyze Query Patterns: Examine your application's log files or use monitoring tools to pinpoint the most frequent queries. Note the fields involved in
$eq
,$gt
,$lt
,$in
,$nin
, and geospatial operators. - Consider Field Cardinality: High-cardinality fields (fields with many unique values) generally benefit more from indexing than low-cardinality fields (fields with few unique values). Indexing a field with only a few unique values might not significantly improve performance.
-
Prioritize Frequently Used Fields: Index fields that are frequently used in
$eq
,$gt
,$lt
and similar operators in your queries, especially inWHERE
clauses. - Compound Indexes for Multiple Filters: If your queries frequently filter on multiple fields, consider a compound index. Remember the order of fields matters for optimal performance.
- Multi-key Indexes for Arrays: If your data involves arrays, a multi-key index is essential for efficient queries on array elements.
- Geospatial Indexes for Location Data: For applications dealing with location data, geospatial indexes are indispensable for efficient proximity searches.
- Index Coverage: Aim for indexes that cover as many fields in your queries as possible to minimize the need for collection scans.
- Experiment and Monitor: After creating an index, monitor its performance using profiling tools. You might need to adjust your indexes based on observed performance.
What are the performance benefits of using indexes in MongoDB?
Indexes dramatically improve query performance by allowing MongoDB to avoid full collection scans. The benefits include:
- Faster Query Execution: Indexes allow MongoDB to quickly locate relevant documents without examining every document in the collection. This translates to significantly faster query response times.
- Reduced I/O Operations: Indexes minimize the number of disk reads required to retrieve data, leading to lower I/O overhead and improved overall system performance.
- Improved Scalability: By optimizing query performance, indexes enhance the scalability of your MongoDB deployments, enabling them to handle larger datasets and higher query loads more efficiently.
- Enhanced Concurrency: Faster queries free up resources, improving concurrency and allowing the database to handle multiple requests simultaneously without performance degradation.
What are the potential drawbacks or limitations of using different MongoDB index types?
While indexes greatly benefit performance, they also have limitations:
- Storage Overhead: Indexes consume additional storage space. The size of the index depends on the indexed fields and the size of the collection.
- Update Overhead: Inserting, updating, and deleting documents incur an additional overhead due to index maintenance. This overhead is generally small but can become noticeable with extremely high write loads.
- Index Size Limits: There are limits to the size of indexes. Excessively large indexes can impact performance.
-
Index Fragmentation: Over time, indexes can become fragmented, reducing their efficiency. Regularly running
db.collection.reIndex()
can help mitigate this. - Complexity of Compound and Multi-key Indexes: Designing efficient compound and multi-key indexes requires careful consideration of query patterns and field order. Improperly designed indexes can be less effective than expected.
- Geospatial Index Limitations: Geospatial indexes are optimized for specific types of queries. They might not be as efficient for queries that don't leverage their spatial capabilities. Choosing the correct geospatial index type (2dsphere vs. 2d) is crucial for optimal performance.
Remember that judicious index selection is key. Over-indexing can lead to unnecessary storage overhead and write performance degradation. Regularly review and optimize your indexes based on your application's evolving query patterns.
The above is the detailed content of What are the different types of indexes in MongoDB (single, compound, multi-key, geospatial)?. For more information, please follow other related articles on the PHP Chinese website!
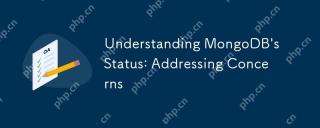
MongoDB is suitable for project needs, but it needs to be used optimized. 1) Performance: Optimize indexing strategies and use sharding technology. 2) Security: Enable authentication and data encryption. 3) Scalability: Use replica sets and sharding technologies.
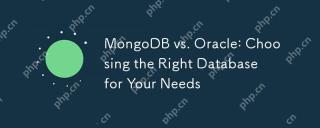
MongoDB is suitable for unstructured data and high scalability requirements, while Oracle is suitable for scenarios that require strict data consistency. 1.MongoDB flexibly stores data in different structures, suitable for social media and the Internet of Things. 2. Oracle structured data model ensures data integrity and is suitable for financial transactions. 3.MongoDB scales horizontally through shards, and Oracle scales vertically through RAC. 4.MongoDB has low maintenance costs, while Oracle has high maintenance costs but is fully supported.
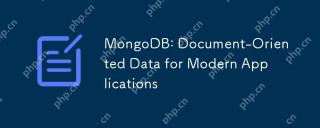
MongoDB has changed the way of development with its flexible documentation model and high-performance storage engine. Its advantages include: 1. Patternless design, allowing fast iteration; 2. The document model supports nesting and arrays, enhancing data structure flexibility; 3. The automatic sharding function supports horizontal expansion, suitable for large-scale data processing.
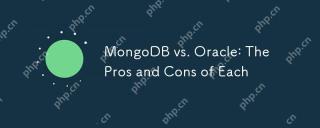
MongoDB is suitable for projects that iterate and process large-scale unstructured data quickly, while Oracle is suitable for enterprise-level applications that require high reliability and complex transaction processing. MongoDB is known for its flexible document storage and efficient read and write operations, suitable for modern web applications and big data analysis; Oracle is known for its strong data management capabilities and SQL support, and is widely used in industries such as finance and telecommunications.
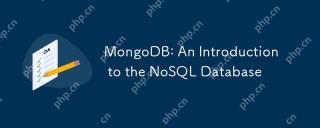
MongoDB is a document-based NoSQL database that uses BSON format to store data, suitable for processing complex and unstructured data. 1) Its document model is flexible and suitable for frequently changing data structures. 2) MongoDB uses WiredTiger storage engine and query optimizer to support efficient data operations and queries. 3) Basic operations include inserting, querying, updating and deleting documents. 4) Advanced usage includes using an aggregation framework for complex data analysis. 5) Common errors include connection problems, query performance problems, and data consistency problems. 6) Performance optimization and best practices include index optimization, data modeling, sharding, caching, monitoring and tuning.
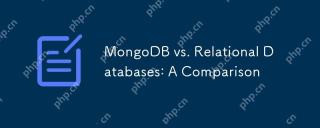
MongoDB is suitable for scenarios that require flexible data models and high scalability, while relational databases are more suitable for applications that complex queries and transaction processing. 1) MongoDB's document model adapts to the rapid iterative modern application development. 2) Relational databases support complex queries and financial systems through table structure and SQL. 3) MongoDB achieves horizontal scaling through sharding, which is suitable for large-scale data processing. 4) Relational databases rely on vertical expansion and are suitable for scenarios where queries and indexes need to be optimized.
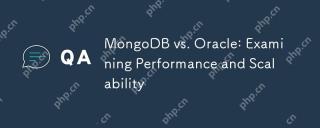
MongoDB performs excellent in performance and scalability, suitable for high scalability and flexibility requirements; Oracle performs excellent in requiring strict transaction control and complex queries. 1.MongoDB achieves high scalability through sharding technology, suitable for large-scale data and high concurrency scenarios. 2. Oracle relies on optimizers and parallel processing to improve performance, suitable for structured data and transaction control needs.
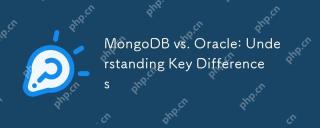
MongoDB is suitable for handling large-scale unstructured data, and Oracle is suitable for enterprise-level applications that require transaction consistency. 1.MongoDB provides flexibility and high performance, suitable for processing user behavior data. 2. Oracle is known for its stability and powerful functions and is suitable for financial systems. 3.MongoDB uses document models, and Oracle uses relational models. 4.MongoDB is suitable for social media applications, while Oracle is suitable for enterprise-level applications.


Hot AI Tools

Undresser.AI Undress
AI-powered app for creating realistic nude photos

AI Clothes Remover
Online AI tool for removing clothes from photos.

Undress AI Tool
Undress images for free

Clothoff.io
AI clothes remover

Video Face Swap
Swap faces in any video effortlessly with our completely free AI face swap tool!

Hot Article

Hot Tools

Notepad++7.3.1
Easy-to-use and free code editor

DVWA
Damn Vulnerable Web App (DVWA) is a PHP/MySQL web application that is very vulnerable. Its main goals are to be an aid for security professionals to test their skills and tools in a legal environment, to help web developers better understand the process of securing web applications, and to help teachers/students teach/learn in a classroom environment Web application security. The goal of DVWA is to practice some of the most common web vulnerabilities through a simple and straightforward interface, with varying degrees of difficulty. Please note that this software

MantisBT
Mantis is an easy-to-deploy web-based defect tracking tool designed to aid in product defect tracking. It requires PHP, MySQL and a web server. Check out our demo and hosting services.

SublimeText3 Chinese version
Chinese version, very easy to use

mPDF
mPDF is a PHP library that can generate PDF files from UTF-8 encoded HTML. The original author, Ian Back, wrote mPDF to output PDF files "on the fly" from his website and handle different languages. It is slower than original scripts like HTML2FPDF and produces larger files when using Unicode fonts, but supports CSS styles etc. and has a lot of enhancements. Supports almost all languages, including RTL (Arabic and Hebrew) and CJK (Chinese, Japanese and Korean). Supports nested block-level elements (such as P, DIV),