In this tutorial you'll learn how to handle error conditions in Python from a whole system point of view. Error handling is a critical aspect of design, and it crosses from the lowest levels (sometimes the hardware) all the way to the end users. If you don't have a consistent strategy in place, your system will be unreliable, the user experience will be poor, and you'll have a lot of challenges debugging and troubleshooting.
The key to success is being aware of all these interlocking aspects, considering them explicitly, and forming a solution that addresses each point.
Status Codes vs. Exceptions
There are two main error handling models: status codes and exceptions. Status codes can be used by any programming language. Exceptions require language/runtime support.
Python supports exceptions. Python and its standard library use exceptions liberally to report on many exceptional situations like IO errors, divide by zero, out of bounds indexing, and also some not so exceptional situations like end of iteration (although it is hidden). Most libraries follow suit and raise exceptions.
That means your code will have to handle the exceptions raised by Python and libraries anyway, so you may as well raise exceptions from your code when necessary and not rely on status codes.
Quick Example
Before diving into the inner sanctum of Python exceptions and error handling best practices, let's see some exception handling in action:
def f():<br> return 4 / 0<br>def g():<br> raise Exception("Don't call us. We'll call you")<br>def h():<br> try:<br> f()<br> except Exception as e:<br> print(e)<br> <br> try:<br> g()<br> except Exception as e:<br> print(e)<br>
Here is the output when calling try clause. If no exception occurs, the program skips the except clause.
If you input a whole number, the program works as expected. However, if you enter a float or a string, the program stops executing.
Please enter a number: 10.3<br>Oops! That was no valid number. Try again...<br>Please enter a number: hello<br>Oops! That was no valid number. Try again...<br>Please enter a number: 10.0<br>Oops! That was no valid number. Try again...<br>Please enter a number: <br>
When you catch an exception, you have three options:
- Swallow it quietly (handle it and keep running).
- Do something like logging, but raise the same exception again to let higher levels handle it.
- Raise a different exception instead of the original.
Swallow the Exception
You should swallow the exception if you know how to handle it and can fully recover.
For example, if you receive an input file that may be in different formats (JSON, YAML), you may try parsing it using different parsers. If the JSON parser raised an exception that the file is not a valid JSON file, you swallow it and try with the YAML parser. If the YAML parser failed too then you let the exception propagate out.
import json<br>import yaml<br><br>def parse_file(filename):<br> try:<br> return json.load(open(filename))<br> except json.JSONDecodeError<br> return yaml.load(open(filename))<br>
Note that other exceptions (eg. file not found or no read permissions) will propagate out and will not be caught by the specific except clause. This is a good policy in this case where you want to try the YAML parsing only if the JSON parsing failed due to a JSON encoding issue.
If you want to handle all exceptions then just use except Exception<code>except Exception
. For example:
def f():<br> return 4 / 0<br>def g():<br> raise Exception("Don't call us. We'll call you")<br>def h():<br> try:<br> f()<br> except Exception as e:<br> print(e)<br> <br> try:<br> g()<br> except Exception as e:<br> print(e)<br>
Note that by adding as e
, you bind the exception object to the name e
available in your except clause.
Raise the Same Exception Again
To raise the exception again, just add raise
with no arguments inside your handler. This lets you perform some local handling, but still lets upper levels handle it too. Here, the invoke_function()
function prints the type of exception to the console and then raises the exception again.
Please enter a number: 10.3<br>Oops! That was no valid number. Try again...<br>Please enter a number: hello<br>Oops! That was no valid number. Try again...<br>Please enter a number: 10.0<br>Oops! That was no valid number. Try again...<br>Please enter a number: <br>
Raise a Different Exception
There are several cases where you would want to raise a different exception. Sometimes you want to group multiple different low-level exceptions into a single category that is handled uniformly by higher-level code. In order cases, you need to transform the exception to the user level and provide some application-specific context.
Finally Clause
Sometimes you want to ensure some cleanup code executes even if an exception was raised somewhere along the way. For example, you may have a database connection that you want to close once you're done. Here is the wrong way to do it:
import json<br>import yaml<br><br>def parse_file(filename):<br> try:<br> return json.load(open(filename))<br> except json.JSONDecodeError<br> return yaml.load(open(filename))<br>
If the query()
function raises an exception then the call to close_db_connection()
will never execute and the DB connection will remain open. The finally
clause always executes after a try all exception handler is executed. Here is how to do it correctly:
def print_exception_type(func, *args, **kwargs):<br> try:<br> return func(*args, **kwargs)<br> except Exception as e:<br> print(type(e))<br>
The call to open_db_connection()
may not return a connection or raise an exception itself. In this case there is no need to close the DB connection.
When using finally
, you have to be careful not to raise any exceptions there because they will mask the original exception.
Context Managers
Context managers provide another mechanism to wrap resources like files or DB connections in cleanup code that executes automatically even when exceptions have been raised. Instead of try-finally blocks, you use the with
statement. Here is an example with a file:
def invoke_function(func, *args, **kwargs):<br> try:<br> return func(*args, **kwargs)<br> except Exception as e:<br> print(type(e))<br> raise<br>
Now, even if process()
raised an exception, the file will be closed properly immediately when the scope of the with
block is exited, regardless of whether the exception was handled or not.
Logging
Logging is pretty much a requirement in non-trivial, long-running systems. It is especially useful in web applications where you can treat all exceptions in a generic way: Just log the exception and return an error message to the caller.
When logging, it is useful to log the exception type, the error message, and the stacktrace. All this information is available via the sys.exc_info
object, but if you use the logger.exception()
method in your exception handler, the Python logging system will extract all the relevant information for you.
This is the best practice I recommend:
def f():<br> return 4 / 0<br>def g():<br> raise Exception("Don't call us. We'll call you")<br>def h():<br> try:<br> f()<br> except Exception as e:<br> print(e)<br> <br> try:<br> g()<br> except Exception as e:<br> print(e)<br>
If you follow this pattern then (assuming you set up logging correctly) no matter what happens you'll have a pretty good record in your logs of what went wrong, and you'll be able to fix the issue.
If you raise the exception again, make sure you don't log the same exception over and over again at different levels. It is a waste, and it might confuse you and make you think multiple instances of the same issue occurred, when in practice a single instance was logged multiple times.
The simplest way to do it is to let all exceptions propagate (unless they can be handled confidently and swallowed earlier) and then do the logging close to the top level of your application/system.
Sentry
Logging is a capability. The most common implementation is using log files. But, for large-scale distributed systems with hundreds, thousands or more servers, this is not always the best solution.
To keep track of exceptions across your whole infrastructure, a service like sentry is super helpful. It centralizes all exception reports, and in addition to the stacktrace it adds the state of each stack frame (the value of variables at the time the exception was raised). It also provides a really nice interface with dashboards, reports, and ways to break down the messages by multiple projects. It is open source, so you can run your own server or subscribe to the hosted version.
Below is a screenshot showing how sentry showcases the errors in your Python application.
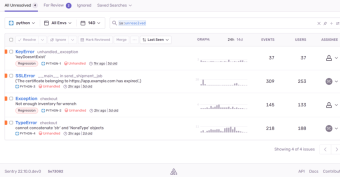
And here is a detailed stack trace of the file causing the error.
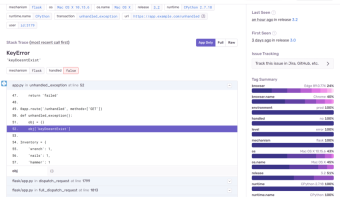
Some failures are temporary, in particular when dealing with distributed systems. A system that freaks out at the first sign of trouble is not very useful.
If your code is accessing some remote system that is not responding, the traditional solution is timeouts, but sometimes not every system is designed with timeouts. Timeouts are not always easy to calibrate as conditions change.
Another approach is to fail fast and then retry. The benefit is that if the target is responding fast then you don't have to spend a lot of time in sleep condition and can react immediately. But if it failed, you can retry multiple times until you decide it is really unreachable and raise an exception. In the next section, I'll introduce a decorator that can do it for you.
Helpful Decorators
Two decorators that can help with error handling are the @log_error
, which logs an exception and then raises it again, and the @retry
decorator, which will retry calling a function several times.
Error Logger
Here is a simple implementation. The decorator excepts a logger object. When it decorates a function and the function is invoked, it will wrap the call in a try-except clause, and if there was an exception, it will log it and finally raise the exception again.
def f():<br> return 4 / 0<br>def g():<br> raise Exception("Don't call us. We'll call you")<br>def h():<br> try:<br> f()<br> except Exception as e:<br> print(e)<br> <br> try:<br> g()<br> except Exception as e:<br> print(e)<br>
Here is how to use it:
Please enter a number: 10.3<br>Oops! That was no valid number. Try again...<br>Please enter a number: hello<br>Oops! That was no valid number. Try again...<br>Please enter a number: 10.0<br>Oops! That was no valid number. Try again...<br>Please enter a number: <br>
Retrier
Here is a very good implementation of the @retry decorator.
import json<br>import yaml<br><br>def parse_file(filename):<br> try:<br> return json.load(open(filename))<br> except json.JSONDecodeError<br> return yaml.load(open(filename))<br>
Conclusion
Error handling is crucial for both users and developers. Python provides great support in the language and standard library for exception-based error handling. By following best practices diligently, you can conquer this often neglected aspect.
This post has been updated with contributions from Esther Vaati. Esther is a software developer and writer for Envato Tuts .
The above is the detailed content of Professional Error Handling With Python. For more information, please follow other related articles on the PHP Chinese website!
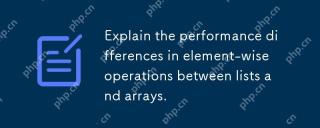
Arraysarebetterforelement-wiseoperationsduetofasteraccessandoptimizedimplementations.1)Arrayshavecontiguousmemoryfordirectaccess,enhancingperformance.2)Listsareflexiblebutslowerduetopotentialdynamicresizing.3)Forlargedatasets,arrays,especiallywithlib
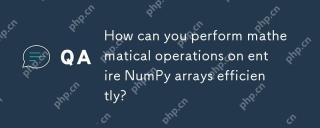
Mathematical operations of the entire array in NumPy can be efficiently implemented through vectorized operations. 1) Use simple operators such as addition (arr 2) to perform operations on arrays. 2) NumPy uses the underlying C language library, which improves the computing speed. 3) You can perform complex operations such as multiplication, division, and exponents. 4) Pay attention to broadcast operations to ensure that the array shape is compatible. 5) Using NumPy functions such as np.sum() can significantly improve performance.
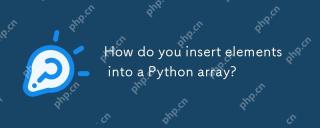
In Python, there are two main methods for inserting elements into a list: 1) Using the insert(index, value) method, you can insert elements at the specified index, but inserting at the beginning of a large list is inefficient; 2) Using the append(value) method, add elements at the end of the list, which is highly efficient. For large lists, it is recommended to use append() or consider using deque or NumPy arrays to optimize performance.
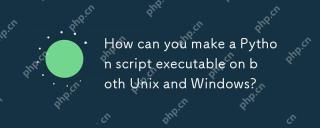
TomakeaPythonscriptexecutableonbothUnixandWindows:1)Addashebangline(#!/usr/bin/envpython3)andusechmod xtomakeitexecutableonUnix.2)OnWindows,ensurePythonisinstalledandassociatedwith.pyfiles,oruseabatchfile(run.bat)torunthescript.
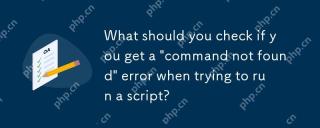
When encountering a "commandnotfound" error, the following points should be checked: 1. Confirm that the script exists and the path is correct; 2. Check file permissions and use chmod to add execution permissions if necessary; 3. Make sure the script interpreter is installed and in PATH; 4. Verify that the shebang line at the beginning of the script is correct. Doing so can effectively solve the script operation problem and ensure the coding process is smooth.
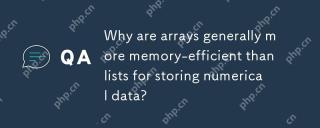
Arraysaregenerallymorememory-efficientthanlistsforstoringnumericaldataduetotheirfixed-sizenatureanddirectmemoryaccess.1)Arraysstoreelementsinacontiguousblock,reducingoverheadfrompointersormetadata.2)Lists,oftenimplementedasdynamicarraysorlinkedstruct
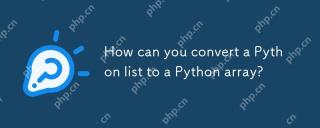
ToconvertaPythonlisttoanarray,usethearraymodule:1)Importthearraymodule,2)Createalist,3)Usearray(typecode,list)toconvertit,specifyingthetypecodelike'i'forintegers.Thisconversionoptimizesmemoryusageforhomogeneousdata,enhancingperformanceinnumericalcomp
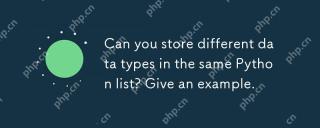
Python lists can store different types of data. The example list contains integers, strings, floating point numbers, booleans, nested lists, and dictionaries. List flexibility is valuable in data processing and prototyping, but it needs to be used with caution to ensure the readability and maintainability of the code.


Hot AI Tools

Undresser.AI Undress
AI-powered app for creating realistic nude photos

AI Clothes Remover
Online AI tool for removing clothes from photos.

Undress AI Tool
Undress images for free

Clothoff.io
AI clothes remover

Video Face Swap
Swap faces in any video effortlessly with our completely free AI face swap tool!

Hot Article

Hot Tools
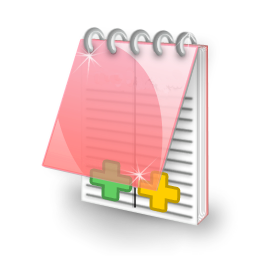
EditPlus Chinese cracked version
Small size, syntax highlighting, does not support code prompt function

Zend Studio 13.0.1
Powerful PHP integrated development environment

PhpStorm Mac version
The latest (2018.2.1) professional PHP integrated development tool
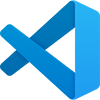
VSCode Windows 64-bit Download
A free and powerful IDE editor launched by Microsoft

mPDF
mPDF is a PHP library that can generate PDF files from UTF-8 encoded HTML. The original author, Ian Back, wrote mPDF to output PDF files "on the fly" from his website and handle different languages. It is slower than original scripts like HTML2FPDF and produces larger files when using Unicode fonts, but supports CSS styles etc. and has a lot of enhancements. Supports almost all languages, including RTL (Arabic and Hebrew) and CJK (Chinese, Japanese and Korean). Supports nested block-level elements (such as P, DIV),
