Edge detection is a crucial image analysis technique for object recognition based on outlines and is vital for image information recovery. It extracts key features like lines and curves, often used by advanced computer vision and image processing algorithms. A robust edge detection algorithm accurately identifies major edges while suppressing noise-induced false edges.
Edges represent significant local changes in image intensity (pixel values), typically occurring at region boundaries. This tutorial explains the Canny edge detection algorithm and its Python implementation.
The Canny Edge Detector
Named after its inventor, John F. Canny (1986), the Canny detector takes a grayscale image as input and outputs an image highlighting intensity discontinuities (edges).
The process involves:
- Noise Reduction: Gaussian convolution smooths the input image, reducing noise.
- Gradient Calculation: A first derivative operator highlights areas with high spatial derivatives. Gradient magnitude and direction are determined using x and y derivatives, crucial for edge direction identification.
- Non-Maximal Suppression: This step thins the edges. The algorithm traces along gradient ridges, setting non-ridge pixels to zero, resulting in a thin edge line. This involves comparing the gradient to its neighbors; only the maximal gradient is retained.
-
Hysteresis Thresholding: Two thresholds,
t1
(upper) andt2
(lower), witht1 > t2
, control edge tracking. Tracking starts at points abovet1
and continues until the gradient falls belowt2
. Points abovet1
are always edges; points belowt1
but abovet2
are edges only if connected to points abovet1
.
The Gaussian kernel width and the t1
/t2
thresholds are parameters influencing the Canny detector's output.
Python Implementation
Two methods are shown: using scikit-image
and OpenCV
.
Using scikit-image
Install scikit-image
(e.g., sudo apt-get install python-skimage
on Ubuntu). The canny()
function (in the feature
module) applies the Canny detector.
Using the sample image "boat.png" (shown below):
The code:
from skimage import io, feature im = io.imread('boat.png') edges = feature.canny(im) io.imshow(edges) io.show()
The output (edge-detected image):
Parameter adjustments yield varying edge detection results.
Using OpenCV
Install OpenCV (see relevant installation guides for your operating system). OpenCV's Canny()
function performs edge detection.
The code:
from skimage import io, feature im = io.imread('boat.png') edges = feature.canny(im) io.imshow(edges) io.show()
Arguments: im
(image), lower threshold (25), upper threshold (255), L2gradient=False
(uses L1-norm). matplotlib
displays the results.
The output (edge-detected image):
Conclusion
This tutorial covered the Canny edge detector and its straightforward implementation using scikit-image
and OpenCV
, demonstrating its effectiveness in edge detection.
The above is the detailed content of Canny Edge Detector Using Python. For more information, please follow other related articles on the PHP Chinese website!
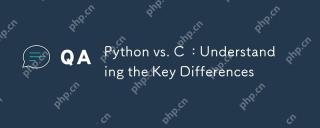
Python and C each have their own advantages, and the choice should be based on project requirements. 1) Python is suitable for rapid development and data processing due to its concise syntax and dynamic typing. 2)C is suitable for high performance and system programming due to its static typing and manual memory management.
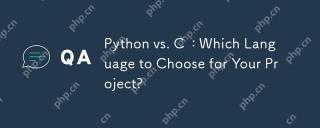
Choosing Python or C depends on project requirements: 1) If you need rapid development, data processing and prototype design, choose Python; 2) If you need high performance, low latency and close hardware control, choose C.
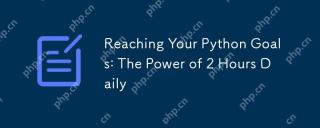
By investing 2 hours of Python learning every day, you can effectively improve your programming skills. 1. Learn new knowledge: read documents or watch tutorials. 2. Practice: Write code and complete exercises. 3. Review: Consolidate the content you have learned. 4. Project practice: Apply what you have learned in actual projects. Such a structured learning plan can help you systematically master Python and achieve career goals.
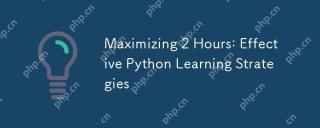
Methods to learn Python efficiently within two hours include: 1. Review the basic knowledge and ensure that you are familiar with Python installation and basic syntax; 2. Understand the core concepts of Python, such as variables, lists, functions, etc.; 3. Master basic and advanced usage by using examples; 4. Learn common errors and debugging techniques; 5. Apply performance optimization and best practices, such as using list comprehensions and following the PEP8 style guide.
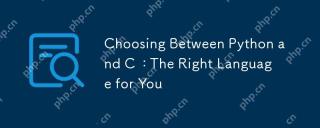
Python is suitable for beginners and data science, and C is suitable for system programming and game development. 1. Python is simple and easy to use, suitable for data science and web development. 2.C provides high performance and control, suitable for game development and system programming. The choice should be based on project needs and personal interests.
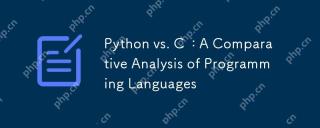
Python is more suitable for data science and rapid development, while C is more suitable for high performance and system programming. 1. Python syntax is concise and easy to learn, suitable for data processing and scientific computing. 2.C has complex syntax but excellent performance and is often used in game development and system programming.
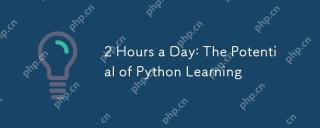
It is feasible to invest two hours a day to learn Python. 1. Learn new knowledge: Learn new concepts in one hour, such as lists and dictionaries. 2. Practice and exercises: Use one hour to perform programming exercises, such as writing small programs. Through reasonable planning and perseverance, you can master the core concepts of Python in a short time.
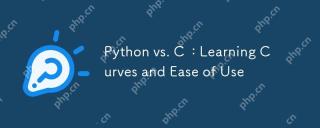
Python is easier to learn and use, while C is more powerful but complex. 1. Python syntax is concise and suitable for beginners. Dynamic typing and automatic memory management make it easy to use, but may cause runtime errors. 2.C provides low-level control and advanced features, suitable for high-performance applications, but has a high learning threshold and requires manual memory and type safety management.


Hot AI Tools

Undresser.AI Undress
AI-powered app for creating realistic nude photos

AI Clothes Remover
Online AI tool for removing clothes from photos.

Undress AI Tool
Undress images for free

Clothoff.io
AI clothes remover

Video Face Swap
Swap faces in any video effortlessly with our completely free AI face swap tool!

Hot Article

Hot Tools

Atom editor mac version download
The most popular open source editor
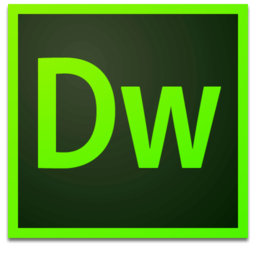
Dreamweaver Mac version
Visual web development tools

PhpStorm Mac version
The latest (2018.2.1) professional PHP integrated development tool

mPDF
mPDF is a PHP library that can generate PDF files from UTF-8 encoded HTML. The original author, Ian Back, wrote mPDF to output PDF files "on the fly" from his website and handle different languages. It is slower than original scripts like HTML2FPDF and produces larger files when using Unicode fonts, but supports CSS styles etc. and has a lot of enhancements. Supports almost all languages, including RTL (Arabic and Hebrew) and CJK (Chinese, Japanese and Korean). Supports nested block-level elements (such as P, DIV),
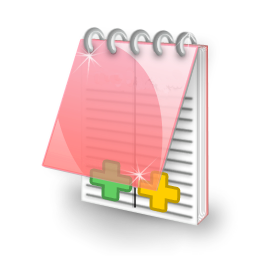
EditPlus Chinese cracked version
Small size, syntax highlighting, does not support code prompt function