Timesheets: the bane of every software engineer's existence. Wouldn't you rather wrestle a complex bug at 3 AM than meticulously document your workday? Unfortunately, freelancing or full-time employment often necessitates this tedious task.
This year, I reached my limit. After a hectic year of projects – some cancelled, some redesigned, others indefinitely postponed – I faced a looming year-end timesheet deadline. The prospect of manually recreating my entire year's work was daunting. My solution? Automate it.
This is my journey from timesheet dread to a coding adventure. Get ready for a streamlined, efficient approach.
The Problem: Timesheets Are a Nightmare
Let's set the stage:
- The Challenge: Record every hour spent on every task for the entire year.
- The Hurdle: My memory is less reliable than a poorly written unit test.
- The Deadline: One day. Just one.
Manual entry was impossible. My plan: extract data from my daily tools – JIRA, Git, Slack, and Outlook – and combine it into a comprehensive timesheet.
The Tools
My arsenal:
- JIRA: Task and ticket tracking.
- Git: Commit history (because every good engineer links commits to tickets, right?).
- Slack: Team communication (meetings and messages included).
- Outlook: Calendar events (because, yes, meetings are work).
Step 1: Extracting JIRA Tickets
First, I tackled JIRA. I needed all tickets assigned to me within a specific timeframe. JIRA's robust API and a bit of Python magic made this achievable.
The Script
This Python script retrieves JIRA tickets:
import os from jira import JIRA import pandas as pd from datetime import datetime import logging import sys from typing import List, Dict, Any import argparse # ... (rest of the script remains the same) ...
Functionality
- Authentication: Uses your JIRA email and API token for authentication.
- JQL Query: Constructs a JQL query to fetch tickets assigned to you within a date range.
- Data Export: Exports results to a CSV for analysis.
Step 2: Retrieving Git Commits
Next, I processed Git. Since our team includes JIRA ticket IDs in commit messages, I created a script to extract commit data and link it to tickets.
The Script
import os from jira import JIRA import pandas as pd from datetime import datetime import logging import sys from typing import List, Dict, Any import argparse # ... (rest of the script remains the same) ...
Functionality
-
Git Log: Uses
git log
to fetch commit history. - JIRA ID Extraction: Uses regular expressions to extract JIRA ticket IDs from commit messages.
- CSV Export: Saves results to a CSV.
Step 3: Handling Slack Messages
Slack proved more challenging. Messages are context-rich, making direct task mapping difficult. I bypassed AI (due to cost and complexity) and created a generic ticket for communication time, then wrote a script to fetch Slack messages.
The Script
import subprocess import csv import re def get_git_commits(since_date=None, author=None): # ... (rest of the script remains the same) ...
Functionality
- Conversation List: Retrieves all channels and DMs accessible to the bot.
- Message Retrieval: Retrieves messages within a specified date range.
- CSV Export: Saves messages to a CSV.
Step 4: Capturing Outlook Meetings
Finally, I incorporated meetings. Using the exchangelib
Python library, I created a script to extract calendar events and export them to a CSV.
The Script
import os from datetime import datetime from slack_sdk import WebClient from slack_sdk.errors import SlackApiError import pandas as pd # ... (rest of the script remains the same) ...
Functionality
- Authentication: Uses your Outlook email and password for authentication.
- Calendar Query: Fetches calendar events within a specified date range.
- CSV Export: Saves events to a CSV.
What's Next?
Now I had four CSV files:
- JIRA Tickets: All tasks worked on.
- Git Commits: All code written.
- Slack Messages: All communication.
- Outlook Meetings: All meetings attended.
In Part 2, I'll demonstrate how I combined these datasets to create a complete timesheet. Hint: more Python, data manipulation, and a touch of magic.
Stay tuned! Remember: Efficiency is key.
What's your least favorite task as a software engineer? Have you automated it yet? Share your experiences in the comments!
The above is the detailed content of The Lazy Engineer's Guide to Automating Timesheets: Part 1. For more information, please follow other related articles on the PHP Chinese website!
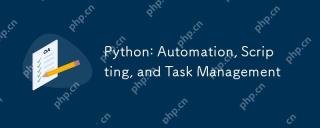
Python excels in automation, scripting, and task management. 1) Automation: File backup is realized through standard libraries such as os and shutil. 2) Script writing: Use the psutil library to monitor system resources. 3) Task management: Use the schedule library to schedule tasks. Python's ease of use and rich library support makes it the preferred tool in these areas.
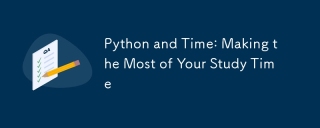
To maximize the efficiency of learning Python in a limited time, you can use Python's datetime, time, and schedule modules. 1. The datetime module is used to record and plan learning time. 2. The time module helps to set study and rest time. 3. The schedule module automatically arranges weekly learning tasks.
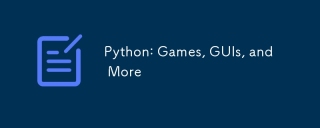
Python excels in gaming and GUI development. 1) Game development uses Pygame, providing drawing, audio and other functions, which are suitable for creating 2D games. 2) GUI development can choose Tkinter or PyQt. Tkinter is simple and easy to use, PyQt has rich functions and is suitable for professional development.
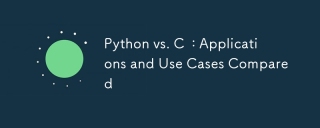
Python is suitable for data science, web development and automation tasks, while C is suitable for system programming, game development and embedded systems. Python is known for its simplicity and powerful ecosystem, while C is known for its high performance and underlying control capabilities.
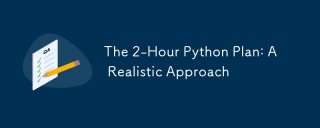
You can learn basic programming concepts and skills of Python within 2 hours. 1. Learn variables and data types, 2. Master control flow (conditional statements and loops), 3. Understand the definition and use of functions, 4. Quickly get started with Python programming through simple examples and code snippets.
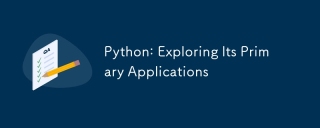
Python is widely used in the fields of web development, data science, machine learning, automation and scripting. 1) In web development, Django and Flask frameworks simplify the development process. 2) In the fields of data science and machine learning, NumPy, Pandas, Scikit-learn and TensorFlow libraries provide strong support. 3) In terms of automation and scripting, Python is suitable for tasks such as automated testing and system management.
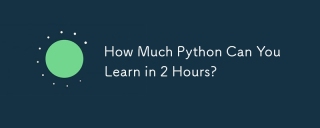
You can learn the basics of Python within two hours. 1. Learn variables and data types, 2. Master control structures such as if statements and loops, 3. Understand the definition and use of functions. These will help you start writing simple Python programs.
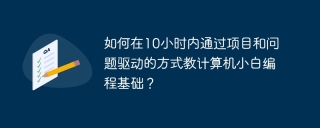
How to teach computer novice programming basics within 10 hours? If you only have 10 hours to teach computer novice some programming knowledge, what would you choose to teach...


Hot AI Tools

Undresser.AI Undress
AI-powered app for creating realistic nude photos

AI Clothes Remover
Online AI tool for removing clothes from photos.

Undress AI Tool
Undress images for free

Clothoff.io
AI clothes remover

AI Hentai Generator
Generate AI Hentai for free.

Hot Article

Hot Tools

Atom editor mac version download
The most popular open source editor

MinGW - Minimalist GNU for Windows
This project is in the process of being migrated to osdn.net/projects/mingw, you can continue to follow us there. MinGW: A native Windows port of the GNU Compiler Collection (GCC), freely distributable import libraries and header files for building native Windows applications; includes extensions to the MSVC runtime to support C99 functionality. All MinGW software can run on 64-bit Windows platforms.
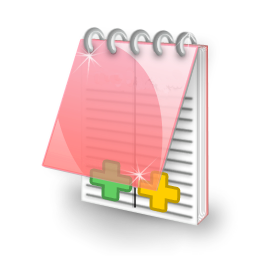
EditPlus Chinese cracked version
Small size, syntax highlighting, does not support code prompt function
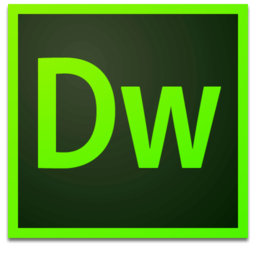
Dreamweaver Mac version
Visual web development tools

Notepad++7.3.1
Easy-to-use and free code editor