Machine learning (ML) is a powerful tool that enables computers to learn from data and make predictions or decisions. But not all machine learning is the same – there are different types of learning, each suitable for specific tasks. The two most common types are supervised learning and unsupervised learning. In this article, we'll explore the differences between them, provide real-world examples, and walk through code snippets to help you understand how they work.
What is supervised learning?
Supervised learning is a type of machine learning in which an algorithm learns from labeled data. In other words, the data you provide to the model includes input features and the correct outputs (labels). The goal is for the model to learn the relationship between inputs and outputs so that it can make accurate predictions on new, unseen data.
Real world examples of supervised learning
Email Spam Detection:
- Input: The text of the email.
- Output: Label indicating whether the email is "Spam" or "Not Spam".
- The model learns to classify emails based on labeled examples.
House Price Forecast:
- Input: Characteristics of the home (e.g. square footage, number of bedrooms, location).
- Output: Price of the house.
- The model learns to predict prices based on historical data.
Medical Diagnosis:
- Input: Patient data (e.g., symptoms, lab results).
- Output: Diagnosis (e.g. "Health" or "Diabetes").
- The model learns to diagnose based on labeled medical records.
What is unsupervised learning?
Unsupervised learning is a type of machine learning in which algorithms learn from unlabeled data. Unlike supervised learning, no correct output is provided. Instead, models try to find patterns, structures, or relationships in the data on their own.
Real world examples of unsupervised learning
Customer segmentation:
- Input: Customer data (e.g. age, purchase history, location).
- Output: Groups of similar customers (e.g., "high-frequency buyers", "budget shoppers").
- The model identifies clusters of customers with similar behavior.
Anomaly detection:
- Input: network traffic data.
- Output: Identify unusual patterns that may indicate a cyber attack.
- The model detects outliers or anomalies in the data.
Market Basket Analysis:
- Input: Grocery store transaction data.
- Output: Groups of products that are often purchased together (e.g., "bread and butter").
- The model identifies associations between products.
The main differences between supervised learning and unsupervised learning
**方面** | **监督学习** | **无监督学习** |
---|---|---|
**数据** | 标记的(提供输入和输出) | 未标记的(仅提供输入) |
**目标** | 预测结果或对数据进行分类 | 发现数据中的模式或结构 |
**示例** | 分类、回归 | 聚类、降维 |
**复杂性** | 更容易评估(已知输出) | 更难评估(没有基本事实) |
**用例** | 垃圾邮件检测、价格预测 | 客户细分、异常检测 |
Code Example
Let’s dig into some code and see how supervised and unsupervised learning work in practice. We will use Python and the popular Scikit-learn library.
Supervised Learning Example: Predicting House Prices
We will use a simple linear regression model to predict the price of a home based on characteristics such as square footage.
# 导入库 import numpy as np import pandas as pd from sklearn.model_selection import train_test_split from sklearn.linear_model import LinearRegression from sklearn.metrics import mean_squared_error # 创建样本数据集 data = { 'SquareFootage': [1400, 1600, 1700, 1875, 1100, 1550, 2350, 2450, 1425, 1700], 'Price': [245000, 312000, 279000, 308000, 199000, 219000, 405000, 324000, 319000, 255000] } df = pd.DataFrame(data) # 特征 (X) 和标签 (y) X = df[['SquareFootage']] y = df['Price'] # 将数据分成训练集和测试集 X_train, X_test, y_train, y_test = train_test_split(X, y, test_size=0.2, random_state=42) # 训练线性回归模型 model = LinearRegression() model.fit(X_train, y_train) # 做出预测 y_pred = model.predict(X_test) # 评估模型 mse = mean_squared_error(y_test, y_pred) print(f"均方误差:{mse:.2f}")
Unsupervised Learning Example: Customer Segmentation
We will use K-means clustering algorithm to group customers based on their age and spending habits.
# 导入库 import numpy as np import pandas as pd from sklearn.cluster import KMeans import matplotlib.pyplot as plt # 创建样本数据集 data = { 'Age': [25, 34, 22, 45, 32, 38, 41, 29, 35, 27], 'SpendingScore': [30, 85, 20, 90, 50, 75, 80, 40, 60, 55] } df = pd.DataFrame(data) # 特征 (X) X = df[['Age', 'SpendingScore']] # 训练 K 均值聚类模型 kmeans = KMeans(n_clusters=3, random_state=42) df['Cluster'] = kmeans.fit_predict(X) # 可视化集群 plt.scatter(df['Age'], df['SpendingScore'], c=df['Cluster'], cmap='viridis') plt.xlabel('年龄') plt.ylabel('消费评分') plt.title('客户细分') plt.show()
When to use supervised learning vs. unsupervised learning
When to use supervised learning:
- You have labeled data.
- You want to predict outcomes or classify data.
- Examples: Predicting sales, classifying images, detecting fraud.
When to use unsupervised learning:
- You have unlabeled data.
- You want to discover hidden patterns or structures.
- Examples: Group customers, reduce data dimensions, and find anomalies.
Conclusion
Supervised learning and unsupervised learning are two basic methods in machine learning, each with its own advantages and use cases. Supervised learning is great for making predictions when you have labeled data, while unsupervised learning is great when you want to explore and discover patterns in unlabeled data.
By understanding the differences and practicing with real-world examples, such as the ones in this article, you will master these basic machine learning techniques. If you have any questions or want to share your own experiences, please feel free to leave a comment below.
The above is the detailed content of Supervised vs. Unsupervised Learning. For more information, please follow other related articles on the PHP Chinese website!
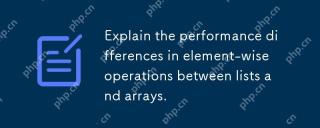
Arraysarebetterforelement-wiseoperationsduetofasteraccessandoptimizedimplementations.1)Arrayshavecontiguousmemoryfordirectaccess,enhancingperformance.2)Listsareflexiblebutslowerduetopotentialdynamicresizing.3)Forlargedatasets,arrays,especiallywithlib
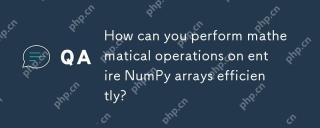
Mathematical operations of the entire array in NumPy can be efficiently implemented through vectorized operations. 1) Use simple operators such as addition (arr 2) to perform operations on arrays. 2) NumPy uses the underlying C language library, which improves the computing speed. 3) You can perform complex operations such as multiplication, division, and exponents. 4) Pay attention to broadcast operations to ensure that the array shape is compatible. 5) Using NumPy functions such as np.sum() can significantly improve performance.
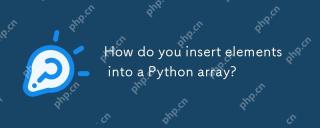
In Python, there are two main methods for inserting elements into a list: 1) Using the insert(index, value) method, you can insert elements at the specified index, but inserting at the beginning of a large list is inefficient; 2) Using the append(value) method, add elements at the end of the list, which is highly efficient. For large lists, it is recommended to use append() or consider using deque or NumPy arrays to optimize performance.
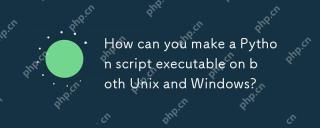
TomakeaPythonscriptexecutableonbothUnixandWindows:1)Addashebangline(#!/usr/bin/envpython3)andusechmod xtomakeitexecutableonUnix.2)OnWindows,ensurePythonisinstalledandassociatedwith.pyfiles,oruseabatchfile(run.bat)torunthescript.
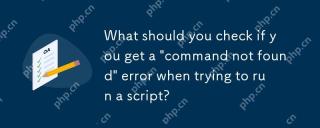
When encountering a "commandnotfound" error, the following points should be checked: 1. Confirm that the script exists and the path is correct; 2. Check file permissions and use chmod to add execution permissions if necessary; 3. Make sure the script interpreter is installed and in PATH; 4. Verify that the shebang line at the beginning of the script is correct. Doing so can effectively solve the script operation problem and ensure the coding process is smooth.
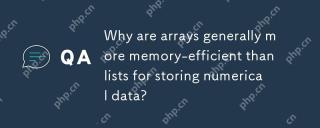
Arraysaregenerallymorememory-efficientthanlistsforstoringnumericaldataduetotheirfixed-sizenatureanddirectmemoryaccess.1)Arraysstoreelementsinacontiguousblock,reducingoverheadfrompointersormetadata.2)Lists,oftenimplementedasdynamicarraysorlinkedstruct
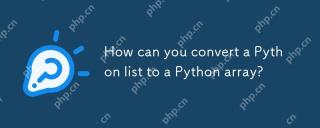
ToconvertaPythonlisttoanarray,usethearraymodule:1)Importthearraymodule,2)Createalist,3)Usearray(typecode,list)toconvertit,specifyingthetypecodelike'i'forintegers.Thisconversionoptimizesmemoryusageforhomogeneousdata,enhancingperformanceinnumericalcomp
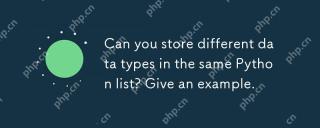
Python lists can store different types of data. The example list contains integers, strings, floating point numbers, booleans, nested lists, and dictionaries. List flexibility is valuable in data processing and prototyping, but it needs to be used with caution to ensure the readability and maintainability of the code.


Hot AI Tools

Undresser.AI Undress
AI-powered app for creating realistic nude photos

AI Clothes Remover
Online AI tool for removing clothes from photos.

Undress AI Tool
Undress images for free

Clothoff.io
AI clothes remover

Video Face Swap
Swap faces in any video effortlessly with our completely free AI face swap tool!

Hot Article

Hot Tools
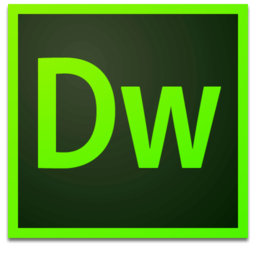
Dreamweaver Mac version
Visual web development tools
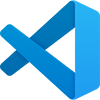
VSCode Windows 64-bit Download
A free and powerful IDE editor launched by Microsoft

SublimeText3 Chinese version
Chinese version, very easy to use

MinGW - Minimalist GNU for Windows
This project is in the process of being migrated to osdn.net/projects/mingw, you can continue to follow us there. MinGW: A native Windows port of the GNU Compiler Collection (GCC), freely distributable import libraries and header files for building native Windows applications; includes extensions to the MSVC runtime to support C99 functionality. All MinGW software can run on 64-bit Windows platforms.

SecLists
SecLists is the ultimate security tester's companion. It is a collection of various types of lists that are frequently used during security assessments, all in one place. SecLists helps make security testing more efficient and productive by conveniently providing all the lists a security tester might need. List types include usernames, passwords, URLs, fuzzing payloads, sensitive data patterns, web shells, and more. The tester can simply pull this repository onto a new test machine and he will have access to every type of list he needs.
