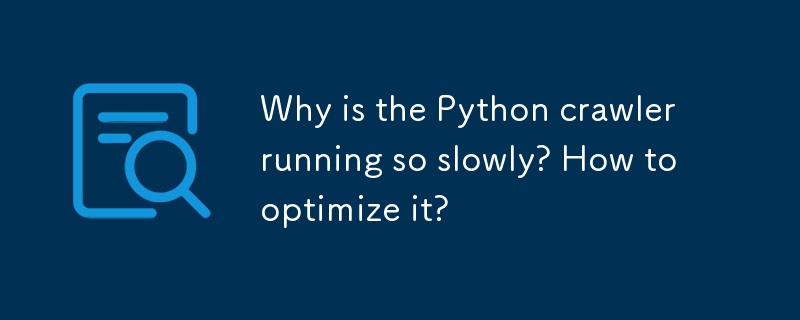
During the development process of Python crawlers, low operating efficiency is a common and thorny problem. This article will deeply explore the reasons why Python crawlers run slowly, and provide a series of practical optimization strategies to help developers significantly improve the crawler running speed. At the same time, we will also mention 98IP proxy as one of the optimization methods to further improve crawler performance.
1. Analysis of the reasons why Python crawler runs slowly
1.1 Low network request efficiency
Network requests are a key part of crawler operation, but they are also the most likely to become bottlenecks. Reasons may include:
-
Frequent HTTP requests: Frequent HTTP requests sent by the crawler without reasonable merging or scheduling will lead to frequent network IO operations, thereby reducing the overall speed.
-
Improper request interval: Too short a request interval may trigger the anti-crawler mechanism of the target website, causing request blocking or IP being blocked, thereby increasing the number of retries and reducing efficiency.
1.2 Data processing bottleneck
Data processing is another major overhead of crawlers, especially when processing massive amounts of data. Reasons may include:
-
Complex parsing methods: Using inefficient data parsing methods, such as using regular expressions (regex) to process complex HTML structures, will significantly affect the processing speed.
-
Improper memory management: Loading a large amount of data into the memory at one time not only takes up a lot of resources, but may also cause memory leaks and affect system performance.
1.3 Unreasonable concurrency control
Concurrency control is an important means to improve crawler efficiency, but if the control is unreasonable, it may reduce efficiency. Reasons may include:
-
Improper thread/process management: Failure to fully utilize multi-core CPU resources, or the communication overhead between threads/processes is too large, resulting in the inability to take advantage of concurrency.
-
Improper asynchronous programming: When using asynchronous programming, if the event loop design is unreasonable or task scheduling is improper, it will lead to performance bottlenecks.
2. Python crawler optimization strategy
2.1 Optimize network requests
-
Use efficient HTTP libraries: For example, the requests library, which is more efficient than urllib and supports connection pooling, can reduce the overhead of TCP connections.
-
Merge requests: For requests that can be merged, try to merge them to reduce the number of network IOs.
-
Set a reasonable request interval: Avoid request intervals that are too short to prevent triggering the anti-crawler mechanism. The request interval can be set using the
time.sleep()
function.
2.2 Optimize data processing
-
Use efficient parsing methods: For example, use BeautifulSoup or the lxml library to parse HTML, which are more efficient than regular expressions.
-
Batch processing of data: Do not load all data into memory at once, but process it in batches to reduce memory usage.
-
Use generators: Generators can generate data on demand, avoiding loading all data into memory at once and improving memory utilization.
2.3 Optimize concurrency control
-
Use multi-threads/multi-processes: Reasonably allocate the number of threads/processes according to the number of CPU cores and make full use of multi-core CPU resources.
-
Use asynchronous programming: For example, the asyncio library, which allows concurrent execution of tasks in a single thread, reducing communication overhead between threads/processes.
-
Use task queues: such as
concurrent.futures.ThreadPoolExecutor
or ProcessPoolExecutor
, which can manage task queues and automatically schedule tasks.
2.4 Use proxy IP (take 98IP proxy as an example)
-
Avoid IP bans: Using proxy IP can hide the real IP address and prevent the crawler from being banned by the target website. Especially when visiting the same website frequently, using a proxy IP can significantly reduce the risk of being banned.
-
Improve the request success rate: By changing the proxy IP, you can bypass the geographical restrictions or access restrictions of some websites and improve the request success rate. This is especially useful for accessing foreign websites or websites that require IP access from a specific region.
-
98IP proxy service: 98IP proxy provides high-quality proxy IP resources and supports multiple protocols and region selections. Using 98IP proxy can improve crawler performance while reducing the risk of being banned. When using it, just configure the proxy IP into the proxy settings for HTTP requests.
3. Sample code
The following is a sample code that uses requests library and BeautifulSoup library to crawl web pages, uses concurrent.futures.ThreadPoolExecutor
for concurrency control, and configures 98IP proxy:
<code class="language-python">import requests
from bs4 import BeautifulSoup
from concurrent.futures import ThreadPoolExecutor
# 目标URL列表
urls = [
'http://example.com/page1',
'http://example.com/page2',
# ....更多URL
]
# 98IP代理配置(示例,实际使用需替换为有效的98IP代理)
proxy = 'http://your_98ip_proxy:port' # 请替换为您的98IP代理地址和端口
# 爬取函数
def fetch_page(url):
try:
headers = {'User-Agent': 'Mozilla/5.0'}
proxies = {'http': proxy, 'https': proxy}
response = requests.get(url, headers=headers, proxies=proxies)
response.raise_for_status() # 检查请求是否成功
soup = BeautifulSoup(response.text, 'html.parser')
# 在此处处理解析后的数据
print(soup.title.string) # 以打印页面标题为例
except Exception as e:
print(f"抓取{url}出错:{e}")
# 使用ThreadPoolExecutor进行并发控制
with ThreadPoolExecutor(max_workers=5) as executor:
executor.map(fetch_page, urls)</code>
In the above code, we use ThreadPoolExecutor
to manage the thread pool and set the maximum number of worker threads to 5. Each thread calls the fetch_page
function to crawl the specified URL. In the fetch_page
function, we use the requests library to send HTTP requests and configure the 98IP proxy to hide the real IP address. At the same time, we also use the BeautifulSoup library to parse HTML content and take printing the page title as an example.
4. Summary
The reason why the Python crawler runs slowly may involve network requests, data processing, and concurrency control. By optimizing these aspects, we can significantly improve the crawler's running speed. In addition, using proxy IP is also one of the important means to improve crawler performance. As a high-quality proxy IP service provider, 98IP proxy can significantly improve crawler performance and reduce the risk of being banned. I hope the content of this article can help developers better understand and optimize the performance of Python crawlers.
The above is the detailed content of Why is the Python crawler running so slowly? How to optimize it?. For more information, please follow other related articles on the PHP Chinese website!