


Mastering Python Memory Optimization: Techniques for Data Science and Machine Learning
As a prolific author, I invite you to explore my Amazon book collection. Remember to follow me on Medium for updates and show your support! Your encouragement is greatly appreciated!
Python's growing prominence in data science and machine learning necessitates efficient memory management for large-scale projects. The expanding size of datasets and increasing computational demands make optimized memory usage critical. My experience with memory-intensive Python applications has yielded several effective optimization strategies, which I'll share here.
We'll begin with NumPy, a cornerstone library for numerical computation. NumPy arrays offer substantial memory advantages over Python lists, particularly for extensive datasets. Their contiguous memory allocation and static typing minimize overhead.
Consider this comparison:
import numpy as np import sys # Creating a list and a NumPy array with 1 million integers py_list = list(range(1000000)) np_array = np.arange(1000000) # Comparing memory usage print(f"Python list size: {sys.getsizeof(py_list) / 1e6:.2f} MB") print(f"NumPy array size: {np_array.nbytes / 1e6:.2f} MB")
The NumPy array's smaller memory footprint will be evident. This disparity becomes more pronounced with larger datasets.
NumPy also provides memory-efficient operations. Instead of generating new arrays for each operation, it often modifies arrays in-place:
# In-place operations np_array += 1 # Modifies the original array directly
Turning to Pandas, categorical data types are key to memory optimization. For string columns with limited unique values, converting to categorical type drastically reduces memory consumption:
import pandas as pd # DataFrame with repeated string values df = pd.DataFrame({'category': ['A', 'B', 'C'] * 1000000}) # Memory usage check print(f"Original memory usage: {df.memory_usage(deep=True).sum() / 1e6:.2f} MB") # Conversion to categorical df['category'] = pd.Categorical(df['category']) # Post-conversion memory usage print(f"Memory usage after conversion: {df.memory_usage(deep=True).sum() / 1e6:.2f} MB")
The memory savings can be substantial, especially with large datasets containing repetitive strings.
For sparse datasets, Pandas offers sparse data structures, storing only non-null values, resulting in significant memory savings for datasets with numerous null or zero values:
# Creating a sparse series sparse_series = pd.Series([0, 0, 1, 0, 2, 0, 0, 3], dtype="Sparse[int]") print(f"Memory usage: {sparse_series.memory_usage(deep=True) / 1e3:.2f} KB")
When datasets exceed available RAM, memory-mapped files are transformative. They allow working with large files as if they were in memory, without loading the entire file:
import mmap import os # Creating a large file with open('large_file.bin', 'wb') as f: f.write(b'0' * 1000000000) # 1 GB file # Memory-mapping the file with open('large_file.bin', 'r+b') as f: mmapped_file = mmap.mmap(f.fileno(), 0) # Reading from the memory-mapped file print(mmapped_file[1000000:1000010]) # Cleaning up mmapped_file.close() os.remove('large_file.bin')
This is particularly useful for random access on large files without loading them completely into memory.
Generator expressions and itertools
are powerful for memory-efficient data processing. They allow processing large datasets without loading everything into memory simultaneously:
import itertools # Generator expression sum_squares = sum(x*x for x in range(1000000)) # Using itertools for memory-efficient operations evens = itertools.islice(itertools.count(0, 2), 1000000) sum_evens = sum(evens) print(f"Sum of squares: {sum_squares}") print(f"Sum of even numbers: {sum_evens}")
These techniques minimize memory overhead while processing large datasets.
For performance-critical code sections, Cython offers significant optimization potential. Compiling Python code to C results in substantial speed improvements and potential memory reduction:
def sum_squares_cython(int n): cdef int i cdef long long result = 0 for i in range(n): result += i * i return result # Usage result = sum_squares_cython(1000000) print(f"Sum of squares: {result}")
This Cython function will outperform its pure Python counterpart, especially for large n
values.
PyPy, a Just-In-Time compiler, offers automatic memory optimizations. It's especially beneficial for long-running programs, often significantly reducing memory usage:
import numpy as np import sys # Creating a list and a NumPy array with 1 million integers py_list = list(range(1000000)) np_array = np.arange(1000000) # Comparing memory usage print(f"Python list size: {sys.getsizeof(py_list) / 1e6:.2f} MB") print(f"NumPy array size: {np_array.nbytes / 1e6:.2f} MB")
PyPy can lead to improved memory efficiency and speed compared to standard CPython.
Memory profiling is essential for identifying optimization opportunities. The memory_profiler
library is a valuable tool:
# In-place operations np_array += 1 # Modifies the original array directly
Use mprof run script.py
and mprof plot
to visualize memory usage.
Addressing memory leaks is crucial. The tracemalloc
module (Python 3.4 ) helps identify memory allocation sources:
import pandas as pd # DataFrame with repeated string values df = pd.DataFrame({'category': ['A', 'B', 'C'] * 1000000}) # Memory usage check print(f"Original memory usage: {df.memory_usage(deep=True).sum() / 1e6:.2f} MB") # Conversion to categorical df['category'] = pd.Categorical(df['category']) # Post-conversion memory usage print(f"Memory usage after conversion: {df.memory_usage(deep=True).sum() / 1e6:.2f} MB")
This pinpoints memory-intensive code sections.
For extremely memory-intensive applications, custom memory management might be necessary. This could involve object pools for object reuse or custom caching:
# Creating a sparse series sparse_series = pd.Series([0, 0, 1, 0, 2, 0, 0, 3], dtype="Sparse[int]") print(f"Memory usage: {sparse_series.memory_usage(deep=True) / 1e3:.2f} KB")
This minimizes object creation/destruction overhead.
For exceptionally large datasets, consider out-of-core computation libraries like Dask:
import mmap import os # Creating a large file with open('large_file.bin', 'wb') as f: f.write(b'0' * 1000000000) # 1 GB file # Memory-mapping the file with open('large_file.bin', 'r+b') as f: mmapped_file = mmap.mmap(f.fileno(), 0) # Reading from the memory-mapped file print(mmapped_file[1000000:1000010]) # Cleaning up mmapped_file.close() os.remove('large_file.bin')
Dask handles datasets larger than available RAM by dividing computations into smaller chunks.
Algorithm optimization is also vital. Choosing efficient algorithms can significantly reduce memory usage:
import itertools # Generator expression sum_squares = sum(x*x for x in range(1000000)) # Using itertools for memory-efficient operations evens = itertools.islice(itertools.count(0, 2), 1000000) sum_evens = sum(evens) print(f"Sum of squares: {sum_squares}") print(f"Sum of even numbers: {sum_evens}")
This optimized Fibonacci function uses constant memory, unlike a naive recursive implementation.
In summary, effective Python memory optimization combines efficient data structures, specialized libraries, memory-efficient coding, and appropriate algorithms. These techniques reduce memory footprint, enabling handling of larger datasets and more complex computations. Remember to profile your code to identify bottlenecks and focus optimization efforts where they'll have the greatest impact.
101 Books
101 Books, an AI-powered publishing house co-founded by author Aarav Joshi, leverages AI to minimize publishing costs, making quality knowledge accessible (some books are as low as $4!).
Find our Golang Clean Code book on Amazon.
For updates and more titles, search for Aarav Joshi on Amazon. Special discounts are available via [link].
Our Creations
Explore our creations:
Investor Central | Investor Central Spanish | Investor Central German | Smart Living | Epochs & Echoes | Puzzling Mysteries | Hindutva | Elite Dev | JS Schools
We are on Medium
Tech Koala Insights | Epochs & Echoes World | Investor Central Medium | Puzzling Mysteries Medium | Science & Epochs Medium | Modern Hindutva
The above is the detailed content of Mastering Python Memory Optimization: Techniques for Data Science and Machine Learning. For more information, please follow other related articles on the PHP Chinese website!
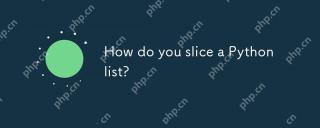
SlicingaPythonlistisdoneusingthesyntaxlist[start:stop:step].Here'showitworks:1)Startistheindexofthefirstelementtoinclude.2)Stopistheindexofthefirstelementtoexclude.3)Stepistheincrementbetweenelements.It'susefulforextractingportionsoflistsandcanuseneg
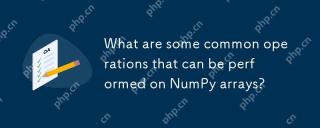
NumPyallowsforvariousoperationsonarrays:1)Basicarithmeticlikeaddition,subtraction,multiplication,anddivision;2)Advancedoperationssuchasmatrixmultiplication;3)Element-wiseoperationswithoutexplicitloops;4)Arrayindexingandslicingfordatamanipulation;5)Ag
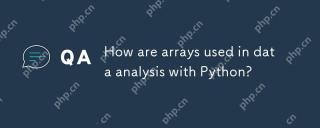
ArraysinPython,particularlythroughNumPyandPandas,areessentialfordataanalysis,offeringspeedandefficiency.1)NumPyarraysenableefficienthandlingoflargedatasetsandcomplexoperationslikemovingaverages.2)PandasextendsNumPy'scapabilitieswithDataFramesforstruc
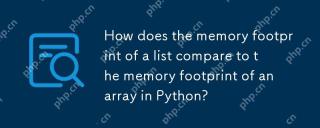
ListsandNumPyarraysinPythonhavedifferentmemoryfootprints:listsaremoreflexiblebutlessmemory-efficient,whileNumPyarraysareoptimizedfornumericaldata.1)Listsstorereferencestoobjects,withoverheadaround64byteson64-bitsystems.2)NumPyarraysstoredatacontiguou
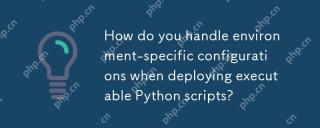
ToensurePythonscriptsbehavecorrectlyacrossdevelopment,staging,andproduction,usethesestrategies:1)Environmentvariablesforsimplesettings,2)Configurationfilesforcomplexsetups,and3)Dynamicloadingforadaptability.Eachmethodoffersuniquebenefitsandrequiresca
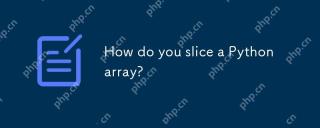
The basic syntax for Python list slicing is list[start:stop:step]. 1.start is the first element index included, 2.stop is the first element index excluded, and 3.step determines the step size between elements. Slices are not only used to extract data, but also to modify and invert lists.
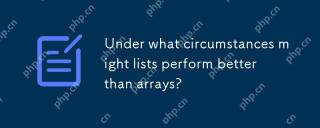
Listsoutperformarraysin:1)dynamicsizingandfrequentinsertions/deletions,2)storingheterogeneousdata,and3)memoryefficiencyforsparsedata,butmayhaveslightperformancecostsincertainoperations.
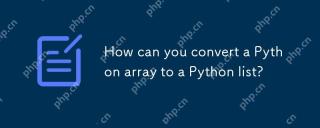
ToconvertaPythonarraytoalist,usethelist()constructororageneratorexpression.1)Importthearraymoduleandcreateanarray.2)Uselist(arr)or[xforxinarr]toconvertittoalist,consideringperformanceandmemoryefficiencyforlargedatasets.


Hot AI Tools

Undresser.AI Undress
AI-powered app for creating realistic nude photos

AI Clothes Remover
Online AI tool for removing clothes from photos.

Undress AI Tool
Undress images for free

Clothoff.io
AI clothes remover

Video Face Swap
Swap faces in any video effortlessly with our completely free AI face swap tool!

Hot Article

Hot Tools

SublimeText3 Chinese version
Chinese version, very easy to use

SAP NetWeaver Server Adapter for Eclipse
Integrate Eclipse with SAP NetWeaver application server.

SublimeText3 English version
Recommended: Win version, supports code prompts!

mPDF
mPDF is a PHP library that can generate PDF files from UTF-8 encoded HTML. The original author, Ian Back, wrote mPDF to output PDF files "on the fly" from his website and handle different languages. It is slower than original scripts like HTML2FPDF and produces larger files when using Unicode fonts, but supports CSS styles etc. and has a lot of enhancements. Supports almost all languages, including RTL (Arabic and Hebrew) and CJK (Chinese, Japanese and Korean). Supports nested block-level elements (such as P, DIV),

SecLists
SecLists is the ultimate security tester's companion. It is a collection of various types of lists that are frequently used during security assessments, all in one place. SecLists helps make security testing more efficient and productive by conveniently providing all the lists a security tester might need. List types include usernames, passwords, URLs, fuzzing payloads, sensitive data patterns, web shells, and more. The tester can simply pull this repository onto a new test machine and he will have access to every type of list he needs.
