


Unlocking Dinosaur Secrets with Machine Learning: A Model Comparison
Machine learning empowers us to unearth hidden patterns within data, leading to insightful predictions and solutions for real-world problems. Let's explore this power by applying it to the fascinating world of dinosaurs! This article compares three popular machine learning models—Naive Bayes, Decision Trees, and Random Forests—as they tackle a unique dinosaur dataset. We'll journey through data exploration, preparation, and model evaluation, highlighting each model's performance and the insights gained.
-
Dinosaur Dataset: A Prehistoric Treasure Trove
Our dataset is a rich collection of dinosaur information, including diet, geological period, location, and size. Each entry represents a unique dinosaur, providing a mix of categorical and numerical data ripe for analysis.
Key Attributes:
- name: Dinosaur species (categorical).
- diet: Dietary habits (e.g., herbivore, carnivore).
- period: Geological period of existence.
- lived_in: Geographic region inhabited.
- length: Approximate size (numerical).
- taxonomy: Taxonomic classification.
Dataset Source: Jurassic Park - The Exhaustive Dinosaur Dataset
-
Data Preparation and Exploration: Unveiling Prehistoric Trends
2.1 Dataset Overview:
Our initial analysis revealed a class imbalance, with herbivores significantly outnumbering other dietary types. This imbalance posed a challenge, particularly for the Naive Bayes model, which assumes equal class representation.
2.2 Data Cleaning:
To ensure data quality, we performed the following:
- Imputation of missing values using appropriate statistical methods.
- Identification and management of outliers in numerical attributes like 'length'.
2.3 Exploratory Data Analysis (EDA):
EDA revealed intriguing patterns and correlations:
- Herbivorous dinosaurs were more common during the Jurassic period.
- Significant size variations existed across different species, as reflected in the 'length' attribute.
-
Feature Engineering: Refining the Data for Optimal Performance
To enhance model accuracy, we employed feature engineering techniques:
- Scaling and Normalization: Standardized numerical features (like 'length') for consistent model input.
- Feature Selection: Prioritized influential attributes such as 'diet', 'taxonomy', and 'period' to focus on the most relevant data.
-
Model Training and Performance Comparison: A Prehistoric Showdown
Our primary objective was to compare the performance of three models on the dinosaur dataset.
4.1 Naive Bayes:
This probabilistic model assumes feature independence. Its simplicity makes it computationally efficient, but its performance suffered due to the dataset's class imbalance, resulting in less accurate predictions for underrepresented classes.
4.2 Decision Tree:
Decision Trees excel at capturing non-linear relationships through hierarchical branching. It performed better than Naive Bayes, effectively identifying complex patterns. However, it showed susceptibility to overfitting if the tree depth wasn't carefully controlled.
4.3 Random Forest:
This ensemble method, combining multiple Decision Trees, proved the most robust. By aggregating predictions, it minimized overfitting and handled the dataset's complexity effectively, achieving the highest accuracy.
-
Results and Analysis: Interpreting the Findings
Key Findings:
- Random Forest achieved superior accuracy and balanced performance across all metrics, demonstrating its strength in handling complex data interactions.
- Decision Tree showed reasonable performance but lagged slightly behind Random Forest in predictive accuracy.
- Naive Bayes struggled with the imbalanced data, leading to lower accuracy and recall.
Challenges and Future Improvements:
- Addressing class imbalance using techniques like SMOTE or resampling could enhance model performance for underrepresented dinosaur types.
- Hyperparameter tuning for Decision Trees and Random Forests could further refine accuracy.
- Exploring alternative ensemble methods, such as boosting, might provide additional insights.
Conclusion: A Journey Through Time and Data Science
This comparative analysis demonstrated the varying performance of machine learning models on a unique dinosaur dataset. The process, from data preparation to model evaluation, revealed the strengths and limitations of each:
- Naive Bayes: Simple and fast, but sensitive to class imbalance.
- Decision Tree: Interpretable and intuitive, but prone to overfitting.
- Random Forest: The most accurate and robust, highlighting the power of ensemble learning.
Random Forest emerged as the most reliable model for this dataset. Future research will explore advanced techniques like boosting and refined feature engineering to further improve prediction accuracy.
Happy coding! ?
For more details, visit my GitHub repository.
The above is the detailed content of Comparative Analysis of Classification Techniques: Naive Bayes, Decision Trees, and Random Forests. For more information, please follow other related articles on the PHP Chinese website!
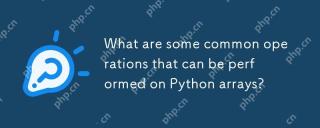
Pythonarrayssupportvariousoperations:1)Slicingextractssubsets,2)Appending/Extendingaddselements,3)Insertingplaceselementsatspecificpositions,4)Removingdeleteselements,5)Sorting/Reversingchangesorder,and6)Listcomprehensionscreatenewlistsbasedonexistin
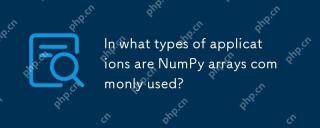
NumPyarraysareessentialforapplicationsrequiringefficientnumericalcomputationsanddatamanipulation.Theyarecrucialindatascience,machinelearning,physics,engineering,andfinanceduetotheirabilitytohandlelarge-scaledataefficiently.Forexample,infinancialanaly
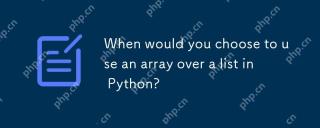
Useanarray.arrayoveralistinPythonwhendealingwithhomogeneousdata,performance-criticalcode,orinterfacingwithCcode.1)HomogeneousData:Arrayssavememorywithtypedelements.2)Performance-CriticalCode:Arraysofferbetterperformancefornumericaloperations.3)Interf
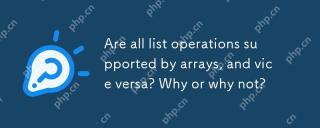
No,notalllistoperationsaresupportedbyarrays,andviceversa.1)Arraysdonotsupportdynamicoperationslikeappendorinsertwithoutresizing,whichimpactsperformance.2)Listsdonotguaranteeconstanttimecomplexityfordirectaccesslikearraysdo.
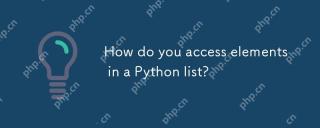
ToaccesselementsinaPythonlist,useindexing,negativeindexing,slicing,oriteration.1)Indexingstartsat0.2)Negativeindexingaccessesfromtheend.3)Slicingextractsportions.4)Iterationusesforloopsorenumerate.AlwayschecklistlengthtoavoidIndexError.
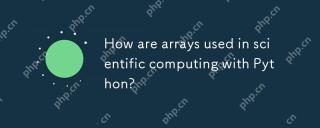
ArraysinPython,especiallyviaNumPy,arecrucialinscientificcomputingfortheirefficiencyandversatility.1)Theyareusedfornumericaloperations,dataanalysis,andmachinelearning.2)NumPy'simplementationinCensuresfasteroperationsthanPythonlists.3)Arraysenablequick
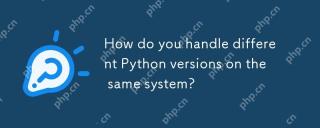
You can manage different Python versions by using pyenv, venv and Anaconda. 1) Use pyenv to manage multiple Python versions: install pyenv, set global and local versions. 2) Use venv to create a virtual environment to isolate project dependencies. 3) Use Anaconda to manage Python versions in your data science project. 4) Keep the system Python for system-level tasks. Through these tools and strategies, you can effectively manage different versions of Python to ensure the smooth running of the project.
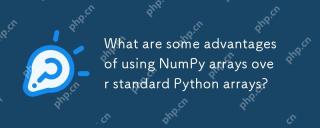
NumPyarrayshaveseveraladvantagesoverstandardPythonarrays:1)TheyaremuchfasterduetoC-basedimplementation,2)Theyaremorememory-efficient,especiallywithlargedatasets,and3)Theyofferoptimized,vectorizedfunctionsformathematicalandstatisticaloperations,making


Hot AI Tools

Undresser.AI Undress
AI-powered app for creating realistic nude photos

AI Clothes Remover
Online AI tool for removing clothes from photos.

Undress AI Tool
Undress images for free

Clothoff.io
AI clothes remover

Video Face Swap
Swap faces in any video effortlessly with our completely free AI face swap tool!

Hot Article

Hot Tools

Safe Exam Browser
Safe Exam Browser is a secure browser environment for taking online exams securely. This software turns any computer into a secure workstation. It controls access to any utility and prevents students from using unauthorized resources.

Notepad++7.3.1
Easy-to-use and free code editor

SAP NetWeaver Server Adapter for Eclipse
Integrate Eclipse with SAP NetWeaver application server.

SublimeText3 Chinese version
Chinese version, very easy to use
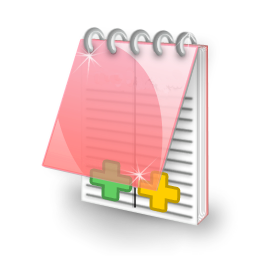
EditPlus Chinese cracked version
Small size, syntax highlighting, does not support code prompt function
