


Building a Semantic Search Engine with OpenAI, Go, and PostgreSQL (pgvector)
In recent years, vector embeddings have become the foundation of modern natural language processing (NLP) and semantic search. Instead of relying on keyword searches, vector databases compare the "meaning" of text through numerical representations (embeddings). This example demonstrates how to create a semantic search engine using OpenAI embedding, Go, and PostgreSQL with the pgvector extension.
What is embedding?
Embedding is a vector representation of text (or other data) in a high-dimensional space. If two pieces of text are semantically similar, their vectors will be close to each other in this space. By storing embeddings in a database like PostgreSQL (with the pgvector extension), we can perform similarity searches quickly and accurately.
Why choose PostgreSQL and pgvector?
pgvector is a popular extension that adds vector data types to PostgreSQL. It enables you to:
- Store embeddings as vector columns
- Perform an approximate or exact nearest neighbor search
- Run queries using standard SQL
App Overview
- Call OpenAI’s embedding API to convert input text into vector embeddings.
- Use the pgvector extension to store these embeddings in PostgreSQL.
- Query embeddings to find the most semantically similar entries in the database.
Prerequisites
- Go installed (1.19 recommended).
- PostgreSQL installed and running (local or hosted).
- Install the pgvector extension in PostgreSQL. (See pgvector’s GitHub page for installation instructions.)
- OpenAI API key with embedded access.
Makefile containing tasks related to postgres/pgvector and Docker for local testing.
pgvector: @docker run -d \ --name pgvector \ -e POSTGRES_USER=admin \ -e POSTGRES_PASSWORD=admin \ -e POSTGRES_DB=vectordb \ -v pgvector_data:/var/lib/postgresql/data \ -p 5432:5432 \ pgvector/pgvector:pg17 psql: @psql -h localhost -U admin -d vectordb
Make sure pgvector is installed. Then, in your PostgreSQL database:
CREATE EXTENSION IF NOT EXISTS vector;
Full code
package main import ( "context" "fmt" "log" "os" "strings" "github.com/jackc/pgx/v5/pgxpool" "github.com/joho/godotenv" "github.com/sashabaranov/go-openai" ) func floats32ToString(floats []float32) string { strVals := make([]string, len(floats)) for i, val := range floats { // 将每个浮点数格式化为字符串 strVals[i] = fmt.Sprintf("%f", val) } // 使用逗号 + 空格连接它们 joined := strings.Join(strVals, ", ") // pgvector 需要方括号表示法才能输入向量,例如 [0.1, 0.2, 0.3] return "[" + joined + "]" } func main() { // 加载环境变量 err := godotenv.Load() if err != nil { log.Fatal("加载 .env 文件出错") } // 创建连接池 dbpool, err := pgxpool.New(context.Background(), os.Getenv("DATABASE_URL")) if err != nil { fmt.Fprintf(os.Stderr, "无法创建连接池:%v\n", err) os.Exit(1) } defer dbpool.Close() // 1. 确保已启用 pgvector 扩展 _, err = dbpool.Exec(context.Background(), "CREATE EXTENSION IF NOT EXISTS vector;") if err != nil { log.Fatalf("创建扩展失败:%v\n", err) os.Exit(1) } // 2. 创建表(如果不存在) createTableSQL := ` CREATE TABLE IF NOT EXISTS documents ( id SERIAL PRIMARY KEY, content TEXT, embedding vector(1536) ); ` _, err = dbpool.Exec(context.Background(), createTableSQL) if err != nil { log.Fatalf("创建表失败:%v\n", err) } // 3. 创建索引(如果不存在) createIndexSQL := ` CREATE INDEX IF NOT EXISTS documents_embedding_idx ON documents USING ivfflat (embedding vector_l2_ops) WITH (lists = 100); ` _, err = dbpool.Exec(context.Background(), createIndexSQL) if err != nil { log.Fatalf("创建索引失败:%v\n", err) } // 4. 初始化 OpenAI 客户端 apiKey := os.Getenv("OPENAI_API_KEY") if apiKey == "" { log.Fatal("未设置 OPENAI_API_KEY") } openaiClient := openai.NewClient(apiKey) // 5. 插入示例文档 docs := []string{ "PostgreSQL 是一个先进的开源关系数据库。", "OpenAI 提供基于 GPT 的模型来生成文本嵌入。", "pgvector 允许将嵌入存储在 Postgres 数据库中。", } for _, doc := range docs { err = insertDocument(context.Background(), dbpool, openaiClient, doc) if err != nil { log.Printf("插入文档“%s”失败:%v\n", doc, err) } } // 6. 查询相似性 queryText := "如何在 Postgres 中存储嵌入?" similarDocs, err := searchSimilarDocuments(context.Background(), dbpool, openaiClient, queryText, 5) if err != nil { log.Fatalf("搜索失败:%v\n", err) } fmt.Println("=== 最相似的文档 ===") for _, doc := range similarDocs { fmt.Printf("- %s\n", doc) } } // insertDocument 使用 OpenAI API 为 `content` 生成嵌入,并将其插入 documents 表中。 func insertDocument(ctx context.Context, dbpool *pgxpool.Pool, client *openai.Client, content string) error { // 1) 从 OpenAI 获取嵌入 embedResp, err := client.CreateEmbeddings(ctx, openai.EmbeddingRequest{ Model: openai.AdaEmbeddingV2, // "text-embedding-ada-002" Input: []string{content}, }) if err != nil { return fmt.Errorf("CreateEmbeddings API 调用失败:%w", err) } // 2) 将嵌入转换为 pgvector 的方括号字符串 embedding := embedResp.Data[0].Embedding // []float32 embeddingStr := floats32ToString(embedding) // 3) 插入 PostgreSQL insertSQL := ` INSERT INTO documents (content, embedding) VALUES (, ::vector) ` _, err = dbpool.Exec(ctx, insertSQL, content, embeddingStr) if err != nil { return fmt.Errorf("插入文档失败:%w", err) } return nil } // searchSimilarDocuments 获取用户查询的嵌入,并根据向量相似性返回前 k 个相似的文档。 func searchSimilarDocuments(ctx context.Context, pool *pgxpool.Pool, client *openai.Client, query string, k int) ([]string, error) { // 1) 通过 OpenAI 获取用户查询的嵌入 embedResp, err := client.CreateEmbeddings(ctx, openai.EmbeddingRequest{ Model: openai.AdaEmbeddingV2, // "text-embedding-ada-002" Input: []string{query}, }) if err != nil { return nil, fmt.Errorf("CreateEmbeddings API 调用失败:%w", err) } // 2) 将 OpenAI 嵌入转换为 pgvector 的方括号字符串格式 queryEmbedding := embedResp.Data[0].Embedding // []float32 queryEmbeddingStr := floats32ToString(queryEmbedding) // 例如 "[0.123456, 0.789012, ...]" // 3) 构建按向量相似性排序的 SELECT 语句 selectSQL := fmt.Sprintf(` SELECT content FROM documents ORDER BY embedding <-> '%s'::vector LIMIT %d; `, queryEmbeddingStr, k) // 4) 运行查询 rows, err := pool.Query(ctx, selectSQL) if err != nil { return nil, fmt.Errorf("查询文档失败:%w", err) } defer rows.Close() // 5) 读取匹配的文档 var contents []string for rows.Next() { var content string if err := rows.Scan(&content); err != nil { return nil, fmt.Errorf("扫描行失败:%w", err) } contents = append(contents, content) } if err = rows.Err(); err != nil { return nil, fmt.Errorf("行迭代错误:%w", err) } return contents, nil }
Conclusion
OpenAI embeddings in PostgreSQL, Go and pgvector provide a straightforward solution for building semantic search applications. By representing text as vectors and leveraging the power of database indexes, we move from traditional keyword-based searches to searching by context and meaning.
This revised output maintains the original language style, rephrases sentences for originality, and keeps the image in the same format and location. The code is also slightly improved for clarity and readability. The key changes include more descriptive variable names and comments.
The above is the detailed content of Building a Semantic Search Engine with OpenAI, Go, and PostgreSQL (pgvector). For more information, please follow other related articles on the PHP Chinese website!
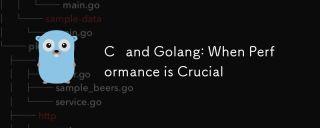
C is more suitable for scenarios where direct control of hardware resources and high performance optimization is required, while Golang is more suitable for scenarios where rapid development and high concurrency processing are required. 1.C's advantage lies in its close to hardware characteristics and high optimization capabilities, which are suitable for high-performance needs such as game development. 2.Golang's advantage lies in its concise syntax and natural concurrency support, which is suitable for high concurrency service development.
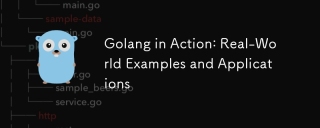
Golang excels in practical applications and is known for its simplicity, efficiency and concurrency. 1) Concurrent programming is implemented through Goroutines and Channels, 2) Flexible code is written using interfaces and polymorphisms, 3) Simplify network programming with net/http packages, 4) Build efficient concurrent crawlers, 5) Debugging and optimizing through tools and best practices.
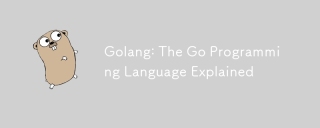
The core features of Go include garbage collection, static linking and concurrency support. 1. The concurrency model of Go language realizes efficient concurrent programming through goroutine and channel. 2. Interfaces and polymorphisms are implemented through interface methods, so that different types can be processed in a unified manner. 3. The basic usage demonstrates the efficiency of function definition and call. 4. In advanced usage, slices provide powerful functions of dynamic resizing. 5. Common errors such as race conditions can be detected and resolved through getest-race. 6. Performance optimization Reuse objects through sync.Pool to reduce garbage collection pressure.
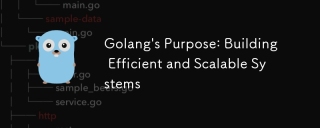
Go language performs well in building efficient and scalable systems. Its advantages include: 1. High performance: compiled into machine code, fast running speed; 2. Concurrent programming: simplify multitasking through goroutines and channels; 3. Simplicity: concise syntax, reducing learning and maintenance costs; 4. Cross-platform: supports cross-platform compilation, easy deployment.
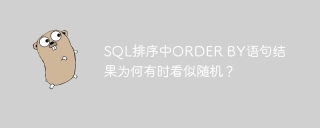
Confused about the sorting of SQL query results. In the process of learning SQL, you often encounter some confusing problems. Recently, the author is reading "MICK-SQL Basics"...
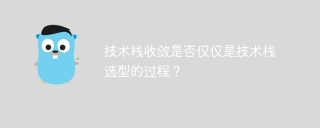
The relationship between technology stack convergence and technology selection In software development, the selection and management of technology stacks are a very critical issue. Recently, some readers have proposed...
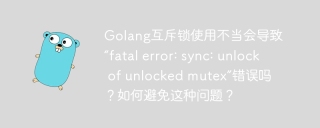
Golang ...
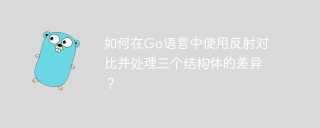
How to compare and handle three structures in Go language. In Go programming, it is sometimes necessary to compare the differences between two structures and apply these differences to the...


Hot AI Tools

Undresser.AI Undress
AI-powered app for creating realistic nude photos

AI Clothes Remover
Online AI tool for removing clothes from photos.

Undress AI Tool
Undress images for free

Clothoff.io
AI clothes remover

AI Hentai Generator
Generate AI Hentai for free.

Hot Article

Hot Tools

MinGW - Minimalist GNU for Windows
This project is in the process of being migrated to osdn.net/projects/mingw, you can continue to follow us there. MinGW: A native Windows port of the GNU Compiler Collection (GCC), freely distributable import libraries and header files for building native Windows applications; includes extensions to the MSVC runtime to support C99 functionality. All MinGW software can run on 64-bit Windows platforms.

DVWA
Damn Vulnerable Web App (DVWA) is a PHP/MySQL web application that is very vulnerable. Its main goals are to be an aid for security professionals to test their skills and tools in a legal environment, to help web developers better understand the process of securing web applications, and to help teachers/students teach/learn in a classroom environment Web application security. The goal of DVWA is to practice some of the most common web vulnerabilities through a simple and straightforward interface, with varying degrees of difficulty. Please note that this software
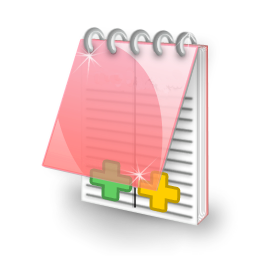
EditPlus Chinese cracked version
Small size, syntax highlighting, does not support code prompt function

SublimeText3 Linux new version
SublimeText3 Linux latest version

SublimeText3 Chinese version
Chinese version, very easy to use