Introduction
Recently, I started diving into the world of Kotlin, a modern and versatile programming language that has captured my attention. However, as someone used to Jupyter's interactive environment, which allows for quick iterations and fluid code exploration, I was wondering if something similar existed for Kotlin.
To my pleasant surprise, I discovered that there is a Jupyter kernel for Kotlin. This tool combines the power and elegance of Kotlin with the interactivity and ease of use of Jupyter, creating an ideal development environment to learn and experiment with the language.
In this post, I will share my experience setting up a Jupyter environment with Kotlin support, and I will even go a step further, creating a notebook that allows you to work with multiple languages simultaneously.
Creating a container with Kotlin
Installing the Kotlin kernel for Jupyter is relatively simple, especially if we use Docker to create a controlled and reproducible environment. Let's look at the Dockerfile I created for this purpose – check the comments to understand each step:
Dockerfile
We start with an official Jupyter image downloaded from quay.io. We use a specific version to ensure reproducibility and label the image as kotlin-kernel for easy identification.
FROM quay.io/jupyter/base-notebook:2024-12-31 AS kotlin-kernel
We install OpenJDK 21, necessary to run Kotlin, the installation is done as root to avoid permissions problems and then we switch to the non-root user to ensure the security of the image.
USER root RUN apt-get update && apt-get -y install openjdk-21-jdk USER jovyan
We install the Kotlin kernel for Jupyter, this will allow us to run Kotlin code in our notebook.
RUN pip install --user \ kotlin-jupyter-kernel==0.12.0.322
We create a directory to store the notebooks.
RUN mkdir -p /home/jovyan/notebooks
Finally, we establish the NOTEBOOK_ARGS environment variable that allows us to configure the notebook with the options we need, in this case, we do not want a browser to open automatically and we want the notebook directory to be /home/jovyan/notebooks.
ENV NOTEBOOK_ARGS="--no-browser --notebook-dir=/home/jovyan/notebooks"
To build the Docker image, we run:
docker build --target kotlin-kernel -t kotlin-kernel .
This command builds the Docker image and tags it as kotlin-kernel.
To run the container:
docker run \ -it \ -p 8888:8888 \ -v $(pwd)/notebooks:/home/jovyan/notebooks \ kotlin-kernel
This command:
- Run the container in interactive mode (-it).
- Maps port 8888 of the container to port 8888 of the host (-p 8888:8888).
- Mounts the local notebooks directory into the :/home/jovyan/notebooks directory of the container (-v $(pwd)/notebooks::/home/jovyan/notebooks).
Once executed, you will be able to access JupyterLab in your browser and you will see that the Launcher already has two kernels available: Python and Kotlin.
And in fact, we can now create notebooks with Kotlin!
The next step in interactivity
During deeper into Kotlin, I noticed some interesting similarities with Python. This led me to want to visualize these similarities in more detail, creating direct comparisons between the two languages. I wondered if it would be possible to run Python and Kotlin code on the same notebook, and it turns out it is possible.
I discovered an extension (and Jupyter kernel) called SoS (Script of Scripts) that allows this functionality. I decided to add it to my container with the Kotlin kernel. Here are the additions to the Dockerfile:
Dockerfile update
We install SoS, which will allow us to run Python and Kotlin code in the same notebook.
FROM quay.io/jupyter/base-notebook:2024-12-31 AS kotlin-kernel
With these additions, we can now build and run our improved container:
USER root RUN apt-get update && apt-get -y install openjdk-21-jdk USER jovyan
When you access JupyterLab now, you will see three kernels available: Python, Kotlin and SoS.
And now we can run Python and Kotlin code in the same notebook:
Extra customization
To improve the visual experience and easily distinguish between cells of different languages, I decided to customize the appearance of the cells.
Jupyter Notebook allows you to add custom CSS, which allows us to add gradients to the left of each cell, depending on the language.
Here is the CSS I used:
RUN pip install --user \ kotlin-jupyter-kernel==0.12.0.322
To implement this customization, I saved the CSS in a file called custom.css and added it to the Dockerfile:
RUN mkdir -p /home/jovyan/notebooks
In addition, it is necessary to specify to the jupyter lab command that we want to use this custom CSS, adding the --custom-css flag to the execution command.
ENV NOTEBOOK_ARGS="--no-browser --notebook-dir=/home/jovyan/notebooks"
Errors and how to hide them
During using the multi-language kernel, an error occasionally appears when running a Kotlin cell. This error is displayed randomly and, although I have not yet been able to identify its origin or how to definitively resolve it, I have found a temporary solution to improve the user experience.
To hide this annoying error, I decided to use CSS. I added the following line to the custom.css file mentioned above:
FROM quay.io/jupyter/base-notebook:2024-12-31 AS kotlin-kernel
This line of CSS hides Kotlin-specific error messages in the notebook. Although it is not an ideal solution as it could hide important errors, it significantly improves the visual experience when working with Kotlin notebooks, especially when dealing with this recurring and seemingly harmless error.
Conclusion
In this post, we have explored how to create an interactive development environment for Kotlin using Jupyter Notebooks.
We start with the basic setup of a Docker container with Kotlin support, then move towards a more sophisticated environment that allows code execution in multiple languages within the same notebook.
In addition, we have seen how to customize the appearance of our notebooks to improve the visual experience and readability, and how to "hide" some common errors that may arise while using these notebooks.
This not only makes learning Kotlin easier, but also allows direct comparisons to other languages like Python, which can be extremely useful for developers who are transitioning to Kotlin or who regularly work with multiple programming languages.
Additional resources
For those interested in further exploring or replicating this environment, I have made all the code used in this project available in my GitHub repository.
I hope this guide is useful to you in your learning journey with Kotlin and Jupyter.
The above is the detailed content of Creating a notebook with Jupyter and Kotlin. For more information, please follow other related articles on the PHP Chinese website!
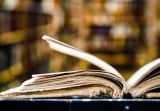
This tutorial demonstrates how to use Python to process the statistical concept of Zipf's law and demonstrates the efficiency of Python's reading and sorting large text files when processing the law. You may be wondering what the term Zipf distribution means. To understand this term, we first need to define Zipf's law. Don't worry, I'll try to simplify the instructions. Zipf's Law Zipf's law simply means: in a large natural language corpus, the most frequently occurring words appear about twice as frequently as the second frequent words, three times as the third frequent words, four times as the fourth frequent words, and so on. Let's look at an example. If you look at the Brown corpus in American English, you will notice that the most frequent word is "th
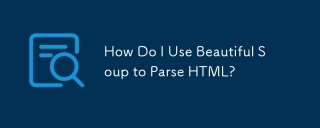
This article explains how to use Beautiful Soup, a Python library, to parse HTML. It details common methods like find(), find_all(), select(), and get_text() for data extraction, handling of diverse HTML structures and errors, and alternatives (Sel
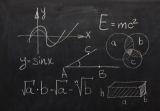
Python's statistics module provides powerful data statistical analysis capabilities to help us quickly understand the overall characteristics of data, such as biostatistics and business analysis. Instead of looking at data points one by one, just look at statistics such as mean or variance to discover trends and features in the original data that may be ignored, and compare large datasets more easily and effectively. This tutorial will explain how to calculate the mean and measure the degree of dispersion of the dataset. Unless otherwise stated, all functions in this module support the calculation of the mean() function instead of simply summing the average. Floating point numbers can also be used. import random import statistics from fracti
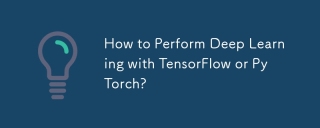
This article compares TensorFlow and PyTorch for deep learning. It details the steps involved: data preparation, model building, training, evaluation, and deployment. Key differences between the frameworks, particularly regarding computational grap
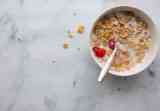
Serialization and deserialization of Python objects are key aspects of any non-trivial program. If you save something to a Python file, you do object serialization and deserialization if you read the configuration file, or if you respond to an HTTP request. In a sense, serialization and deserialization are the most boring things in the world. Who cares about all these formats and protocols? You want to persist or stream some Python objects and retrieve them in full at a later time. This is a great way to see the world on a conceptual level. However, on a practical level, the serialization scheme, format or protocol you choose may determine the speed, security, freedom of maintenance status, and other aspects of the program
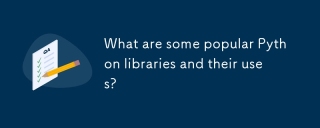
The article discusses popular Python libraries like NumPy, Pandas, Matplotlib, Scikit-learn, TensorFlow, Django, Flask, and Requests, detailing their uses in scientific computing, data analysis, visualization, machine learning, web development, and H
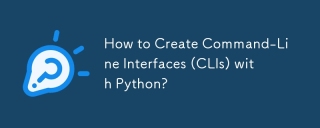
This article guides Python developers on building command-line interfaces (CLIs). It details using libraries like typer, click, and argparse, emphasizing input/output handling, and promoting user-friendly design patterns for improved CLI usability.
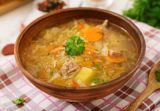
This tutorial builds upon the previous introduction to Beautiful Soup, focusing on DOM manipulation beyond simple tree navigation. We'll explore efficient search methods and techniques for modifying HTML structure. One common DOM search method is ex


Hot AI Tools

Undresser.AI Undress
AI-powered app for creating realistic nude photos

AI Clothes Remover
Online AI tool for removing clothes from photos.

Undress AI Tool
Undress images for free

Clothoff.io
AI clothes remover

AI Hentai Generator
Generate AI Hentai for free.

Hot Article

Hot Tools
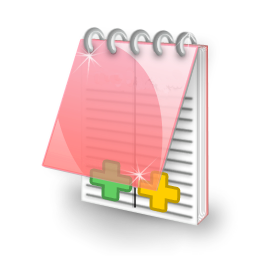
EditPlus Chinese cracked version
Small size, syntax highlighting, does not support code prompt function
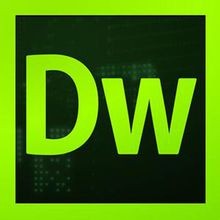
Dreamweaver CS6
Visual web development tools
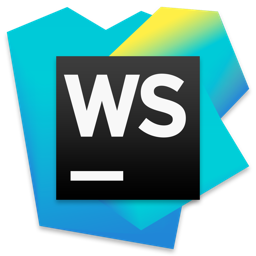
WebStorm Mac version
Useful JavaScript development tools
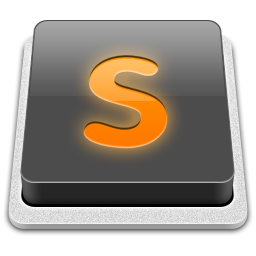
SublimeText3 Mac version
God-level code editing software (SublimeText3)

DVWA
Damn Vulnerable Web App (DVWA) is a PHP/MySQL web application that is very vulnerable. Its main goals are to be an aid for security professionals to test their skills and tools in a legal environment, to help web developers better understand the process of securing web applications, and to help teachers/students teach/learn in a classroom environment Web application security. The goal of DVWA is to practice some of the most common web vulnerabilities through a simple and straightforward interface, with varying degrees of difficulty. Please note that this software
