Yes, I had stopped publishing here, but from a marketing point of view it is better to continue publishing... We continue.
Text originally published here.
The objective of this text is to give a direct summary of the basic concepts necessary to understand concurrency and parallelism in the Python language. I recommend having a minimum background on the subject or combining this text with study from other sources. All references are at the end of the text.
I will cover the following topics:
- What is a process?
- What are threads?
- What do I/O bound and CPU bound mean?
- What is Python GIL?
- What is competition?
- What is parallelism?
- The asyncio library
- The threading library
- The multiprocessing library
What is a process?
In computing, a process is an instance of an application running. If you open an application on your computer, such as a browser, that application will be associated with some process. A process is composed of:
- Hardware context: stores contents of general and CPU-specific registers
- Software context: specifies the resources that can be allocated by the process
- Address space: specifies the area of memory that the process belongs to
The following image was taken from the book by Francis Machado and Luis Maia:
This information is necessary to execute a program.
What are threads?
A thread is a subroutine of a program, being the smallest unit of execution that an operating system manages and a component of a process.
The various threads of a hypothetical process can be executed concurrently (which we will understand shortly), sharing resources such as memory. Different processes do not share these resources.
The image below was taken from Wikipedia:
Interpreting the image above, we can extract that a program is saved on disk (secondary, non-volatile memory) and includes several instructions, and can be instantiated (started) in one or more processes, and these in turn can have several associated threads.
What do I/O bound and CPU bound mean?
These two expressions appear a lot in the discussion about competition and can appear in Portuguese with I/O (input/output) and CPU (central processing unit).
When we talk about I/O bound and CPU bound we are talking about the limiting factors that prevent an operation from running faster on our computer, and we can find these two types of operations in the same codebase.
A CPU bound operation is CPU intensive, and will run faster if the CPU is more powerful. In other words, if we go from 2GHz to 4GHz clock speed, this operation will probably run faster. We are talking here about operations that perform many computations, calculations; for example, how to calculate Pi.
An I/O bound operation depends on the network speed and the speed of the input and output devices. Making a request to a web server or reading a file from disk are I/O bound operations.
Both types of operations can benefit from the use of concurrency.
What is Python's GIL?
GIL stands for global interpreter lock, which aims to prevent a Python process from executing more than one Python instruction bytecode at the same time. To run a thread it is necessary to "acquire" the GIL and while one thread holds the GIL another thread cannot acquire it at the same time. This does not mean that we cannot have more than one thread in this context.
Here we are considering the Python reference implementation. CPython is the standard implementation of Python, used as a reference for how the language behaves. There are other implementations, such as Jython or IronPython. GIL is present in CPython and only recently we had a PEP (Python Enhancement Proposal) proposing to make GIL optional.
The idea of GIL is to prevent race conditions, which can arise when more than one thread needs to reference a Python object at the same time. If more than one thread modifies a shared variable, that variable may be in an unexpected state. Image taken from Matthew Fowler's book:
In the image above, two threads are trying to increase a reference count simultaneously, and instead of the count giving 2, since both are increasing 1, the final result gives 1 (each thread is a column).
What is competition?
Competition in computing happens when dealing with more than one task, without necessarily executing these two tasks at exactly the same time. A well-known phrase from Rob Pyke on the subject:
Competition means dealing with many things at the same time. Parallelism is doing many things at the same time.
Think about this hypothetical situation: if you are going to make two cakes, you can start by preheating the oven and, in the meantime, prepare the dough for the first cake. Once the oven is at the correct temperature, you can place the dough for the first cake in the oven and, while waiting for the cake to rise in the oven, you can prepare the dough for the second cake. The idea of competition is basically this, you don't need to be idle, stuck, stopped, while waiting for a task to complete, you can do a switch and change tasks.
In this context we have two types of multitasking:
- Cooperative multitasking: in this model we explain in the code the points where tasks can be switch. In Python this is achieved using an event loop, a common design pattern, using only one thread and one CPU core, using, for example, asyncio with async and await
- Preemptive multitasking: in this model we let the operating system handle the switch. In Python this is achieved with more than one thread and one CPU core using, for example, the threading lib
The image below helps to summarize concurrency in Python:
What is parallelism?
Parallelism means that more than one task is being executed at the same time. In other words, parallelism implies concurrency (dealing with more than one task), but concurrency does not imply parallelism (tasks are not necessarily being executed in parallel at the same time). For parallelism to be possible we need more than one CPU core.
In Python parallelism is achieved, for example, with the multiprocessing lib, where we will have more than one Python process, each with its own GIL. The image helps illustrate parallelism in Python:
The asyncio library
There are different ways to achieve concurrency and parallelism in Python and we can use some libraries to optimize our code, depending on the type of operation we are dealing with, I/O bound or CPU bound. asyncio is a lib to achieve concurrency using async and await. From the documentation:
Asyncio is used as a foundation for several asynchronous Python frameworks that provide high-performance networking and web servers, database connection libraries, distributed job queues, etc.
As you can imagine, this lib is suitable for optimizing I/O bound tasks, where we have network waiting time, writing to disk, etc. In a CPU bound operation there is no waiting, we only depend on the CPU calculation speed.
The threading library
Python's threading lib allows us to operate more than one thread, however, we still deal with one CPU core and one Python process, and remember that this is a case of preemptive multitasking where the operating system does the task switching for us. The lib is also more useful for optimizing I/O bound operations.
About threading, the Real Python website provides some important points:
Because the operating system is in control of when one task will stop and another task will start, any data that is shared between threads needs to be protected, or thread-safe. Unfortunately requests.Session() is not thread-safe. There are several strategies for making data access thread-safe depending on what the data is and how you are using it. One of them is to use thread-safe data structures as the Queue of the Python queue module.
We found the queue documentation here.
The multiprocessing library
About the multiprocessing lib in the Python documentation:
multiprocessing is a package that supports generating processes using an API similar to the threading module. The multiprocessing package provides both local and remote concurrency, effectively bypassing the GIL by using sub-processes instead of threads. That's why the multiprocessing module allows the programmer to take advantage of multiple processors on one machine.
It is worth pointing out that running more than one process on different CPU cores does not mean disabling the GIL, but rather that each process will have its own GIL. By taking advantage of more than one CPU core, sharing heavy CPU workloads between multiple available cores, the lib is more suitable for CPU bound.
Sources:
FOWLER, Matthew. Python Concurrency with asyncio. Manning Publications, 2022.
MACHADO, Francis Berenger; MAIA, Luiz Paulo. Operating Systems Architecture: Including Exercises with the SOSIM Simulator and ENADE Questions. Rio de Janeiro: LTC, 2013.
Thread (computing) by Wikipedia
Speed Up Your Python Program With Concurrency by Real Python
The above is the detailed content of Concurrency and Parallelism in Python. For more information, please follow other related articles on the PHP Chinese website!
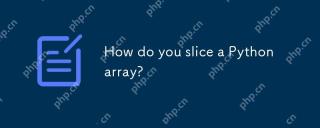
The basic syntax for Python list slicing is list[start:stop:step]. 1.start is the first element index included, 2.stop is the first element index excluded, and 3.step determines the step size between elements. Slices are not only used to extract data, but also to modify and invert lists.
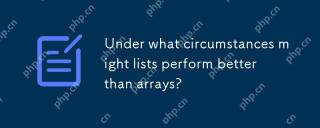
Listsoutperformarraysin:1)dynamicsizingandfrequentinsertions/deletions,2)storingheterogeneousdata,and3)memoryefficiencyforsparsedata,butmayhaveslightperformancecostsincertainoperations.
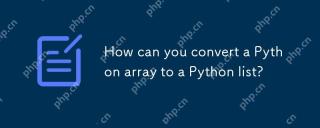
ToconvertaPythonarraytoalist,usethelist()constructororageneratorexpression.1)Importthearraymoduleandcreateanarray.2)Uselist(arr)or[xforxinarr]toconvertittoalist,consideringperformanceandmemoryefficiencyforlargedatasets.
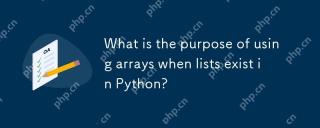
ChoosearraysoverlistsinPythonforbetterperformanceandmemoryefficiencyinspecificscenarios.1)Largenumericaldatasets:Arraysreducememoryusage.2)Performance-criticaloperations:Arraysofferspeedboostsfortaskslikeappendingorsearching.3)Typesafety:Arraysenforc
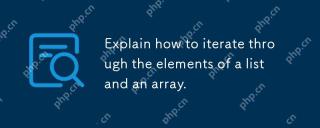
In Python, you can use for loops, enumerate and list comprehensions to traverse lists; in Java, you can use traditional for loops and enhanced for loops to traverse arrays. 1. Python list traversal methods include: for loop, enumerate and list comprehension. 2. Java array traversal methods include: traditional for loop and enhanced for loop.
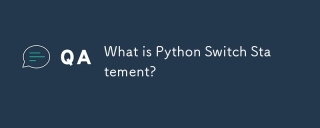
The article discusses Python's new "match" statement introduced in version 3.10, which serves as an equivalent to switch statements in other languages. It enhances code readability and offers performance benefits over traditional if-elif-el
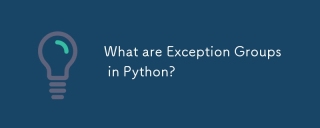
Exception Groups in Python 3.11 allow handling multiple exceptions simultaneously, improving error management in concurrent scenarios and complex operations.
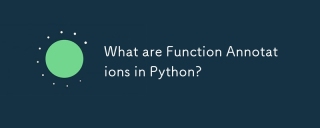
Function annotations in Python add metadata to functions for type checking, documentation, and IDE support. They enhance code readability, maintenance, and are crucial in API development, data science, and library creation.


Hot AI Tools

Undresser.AI Undress
AI-powered app for creating realistic nude photos

AI Clothes Remover
Online AI tool for removing clothes from photos.

Undress AI Tool
Undress images for free

Clothoff.io
AI clothes remover

Video Face Swap
Swap faces in any video effortlessly with our completely free AI face swap tool!

Hot Article

Hot Tools
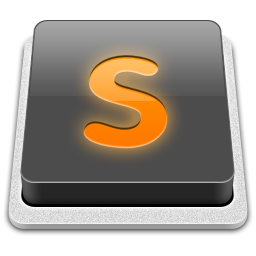
SublimeText3 Mac version
God-level code editing software (SublimeText3)
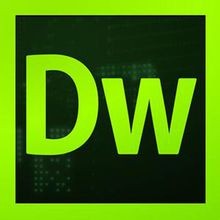
Dreamweaver CS6
Visual web development tools
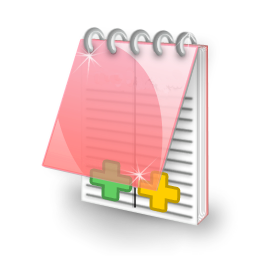
EditPlus Chinese cracked version
Small size, syntax highlighting, does not support code prompt function
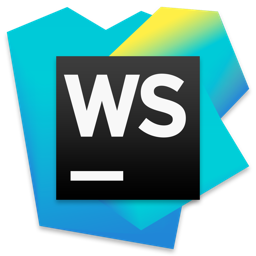
WebStorm Mac version
Useful JavaScript development tools
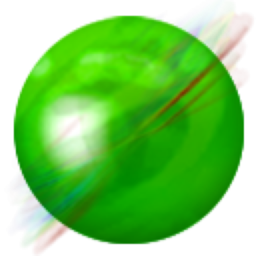
ZendStudio 13.5.1 Mac
Powerful PHP integrated development environment
