


Why Do Functions Defined in Loops Often Return the Same Value, and How Can This Be Fixed?
Creating Functions Within Loops: Addressing Late Binding Issues
When attempting to define individual functions within a loop, it's common to encounter the issue where all functions return the same value despite being intended to represent unique outcomes. This phenomenon, known as late binding, occurs because functions do not receive their arguments until they are called.
Consider the following example using a for loop:
functions = [] for i in range(3): def f(): return i functions.append(f)
As written, each function looks up its corresponding value of i at the time it is called. However, after the loop has executed, all functions will reference the final value of i (2), resulting in the following output:
print([f() for f in functions]) # Expected: [0, 1, 2] # Actual: [2, 2, 2]
Solution: Enforcing Early Binding
To address this issue, it's necessary to force early binding by assigning arguments to functions at definition time rather than call time. This can be achieved by adding a default argument to the function definition:
functions = [] for i in range(3): def f(i=i): return i functions.append(f)
The default argument (in this case, i=i) is evaluated when the function is defined, not when it is called. This ensures that each function retains its unique argument value, producing the desired output:
print([f() for f in functions]) # Output: [0, 1, 2]
Alternative Approach Using Closure
If concern arises over the potential for additional arguments to be passed to the function, a more elaborate approach can be implemented using closures:
def make_f(i): def f(): return i return f
In this scenario, a function factory (make_f) is created. Within the loop, the returned function from make_f is assigned to the f variable instead of calling def f(): directly. This approach guarantees that each function retains its exclusive argument value, like in the early binding solution.
The above is the detailed content of Why Do Functions Defined in Loops Often Return the Same Value, and How Can This Be Fixed?. For more information, please follow other related articles on the PHP Chinese website!
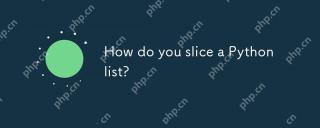
SlicingaPythonlistisdoneusingthesyntaxlist[start:stop:step].Here'showitworks:1)Startistheindexofthefirstelementtoinclude.2)Stopistheindexofthefirstelementtoexclude.3)Stepistheincrementbetweenelements.It'susefulforextractingportionsoflistsandcanuseneg
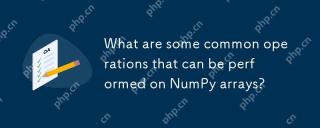
NumPyallowsforvariousoperationsonarrays:1)Basicarithmeticlikeaddition,subtraction,multiplication,anddivision;2)Advancedoperationssuchasmatrixmultiplication;3)Element-wiseoperationswithoutexplicitloops;4)Arrayindexingandslicingfordatamanipulation;5)Ag
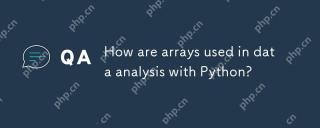
ArraysinPython,particularlythroughNumPyandPandas,areessentialfordataanalysis,offeringspeedandefficiency.1)NumPyarraysenableefficienthandlingoflargedatasetsandcomplexoperationslikemovingaverages.2)PandasextendsNumPy'scapabilitieswithDataFramesforstruc
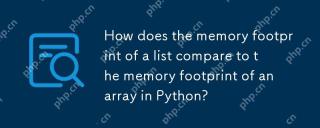
ListsandNumPyarraysinPythonhavedifferentmemoryfootprints:listsaremoreflexiblebutlessmemory-efficient,whileNumPyarraysareoptimizedfornumericaldata.1)Listsstorereferencestoobjects,withoverheadaround64byteson64-bitsystems.2)NumPyarraysstoredatacontiguou
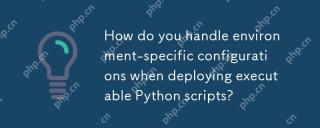
ToensurePythonscriptsbehavecorrectlyacrossdevelopment,staging,andproduction,usethesestrategies:1)Environmentvariablesforsimplesettings,2)Configurationfilesforcomplexsetups,and3)Dynamicloadingforadaptability.Eachmethodoffersuniquebenefitsandrequiresca
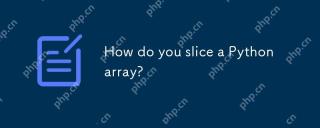
The basic syntax for Python list slicing is list[start:stop:step]. 1.start is the first element index included, 2.stop is the first element index excluded, and 3.step determines the step size between elements. Slices are not only used to extract data, but also to modify and invert lists.
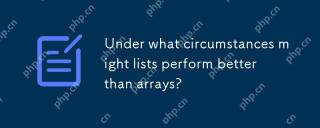
Listsoutperformarraysin:1)dynamicsizingandfrequentinsertions/deletions,2)storingheterogeneousdata,and3)memoryefficiencyforsparsedata,butmayhaveslightperformancecostsincertainoperations.
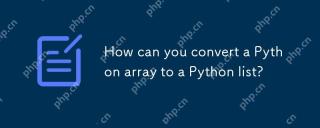
ToconvertaPythonarraytoalist,usethelist()constructororageneratorexpression.1)Importthearraymoduleandcreateanarray.2)Uselist(arr)or[xforxinarr]toconvertittoalist,consideringperformanceandmemoryefficiencyforlargedatasets.


Hot AI Tools

Undresser.AI Undress
AI-powered app for creating realistic nude photos

AI Clothes Remover
Online AI tool for removing clothes from photos.

Undress AI Tool
Undress images for free

Clothoff.io
AI clothes remover

Video Face Swap
Swap faces in any video effortlessly with our completely free AI face swap tool!

Hot Article

Hot Tools
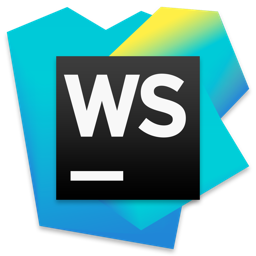
WebStorm Mac version
Useful JavaScript development tools

SublimeText3 English version
Recommended: Win version, supports code prompts!
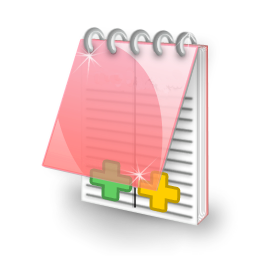
EditPlus Chinese cracked version
Small size, syntax highlighting, does not support code prompt function
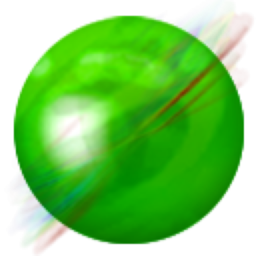
ZendStudio 13.5.1 Mac
Powerful PHP integrated development environment

Atom editor mac version download
The most popular open source editor
