


The Nuances of String Prefixes: "u", "r", and "ur"
In the realm of Python strings, confusion often arises regarding the purpose and functionality of the string prefixes "u", "r", and "ur". This article aims to shed light on their distinct roles and the intricacies of raw string literals.
What Raw String Literals Entail
Contrary to common misconceptions, there is no distinct "raw string" type. Instead, "raw string literals" refer to strings prefixed with the letter "r", such as r'...' or r"""...""". These literals differ only in their handling of backslashes ().
In normal string literals, a backslash followed by another character typically triggers an escape sequence, representing special characters like newlines or tabs. Raw string literals, however, interpret the backslash as itself, except when it precedes a closing single or double quote that would otherwise terminate the string.
Differentiating "u", "r", and "ur" Prefixes
The "u" prefix denotes a Unicode string, which is a Unicode object of type unicode. In Python 2.*, u'...' represents a Unicode string, while '...' is a byte string.
The "r" prefix, as discussed earlier, denotes a raw string literal. It preserves backslashes literally, making it useful for regular expressions or when dealing with native Windows file paths. In Python 2.*, both r'...' and r'''...''' produce byte strings.
The "ur" prefix combines the functionality of "u" and "r", resulting in a raw Unicode string literal. Raw Unicode strings are particularly useful when working with file paths that contain Unicode characters.
Converting Between String Types
In Python 2.*, there is a distinction between byte strings and Unicode strings. To convert from a Unicode string to a byte string, one can use the .encode() method. To convert from a byte string to a Unicode string, one can use the .decode() method.
Encodings and String Prefixes
In Python 2.*, the encoding of a string is determined by the codec used to decode the raw byte data (when creating the string) or to encode the Unicode data (when creating the string). The "u" prefix does not affect the encoding of the resulting Unicode string.
In Python 3.*, strings are Unicode-by-default, and the "u" prefix is no longer necessary. Additionally, raw string literals are not needed for regular expressions as backslashes are not treated as escape sequences in raw strings.
The above is the detailed content of What's the Difference Between Python String Prefixes 'u', 'r', and 'ur'?. For more information, please follow other related articles on the PHP Chinese website!
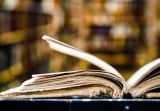
This tutorial demonstrates how to use Python to process the statistical concept of Zipf's law and demonstrates the efficiency of Python's reading and sorting large text files when processing the law. You may be wondering what the term Zipf distribution means. To understand this term, we first need to define Zipf's law. Don't worry, I'll try to simplify the instructions. Zipf's Law Zipf's law simply means: in a large natural language corpus, the most frequently occurring words appear about twice as frequently as the second frequent words, three times as the third frequent words, four times as the fourth frequent words, and so on. Let's look at an example. If you look at the Brown corpus in American English, you will notice that the most frequent word is "th
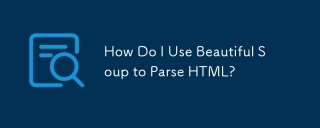
This article explains how to use Beautiful Soup, a Python library, to parse HTML. It details common methods like find(), find_all(), select(), and get_text() for data extraction, handling of diverse HTML structures and errors, and alternatives (Sel
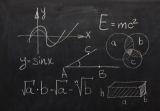
Python's statistics module provides powerful data statistical analysis capabilities to help us quickly understand the overall characteristics of data, such as biostatistics and business analysis. Instead of looking at data points one by one, just look at statistics such as mean or variance to discover trends and features in the original data that may be ignored, and compare large datasets more easily and effectively. This tutorial will explain how to calculate the mean and measure the degree of dispersion of the dataset. Unless otherwise stated, all functions in this module support the calculation of the mean() function instead of simply summing the average. Floating point numbers can also be used. import random import statistics from fracti
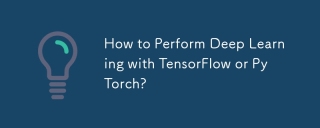
This article compares TensorFlow and PyTorch for deep learning. It details the steps involved: data preparation, model building, training, evaluation, and deployment. Key differences between the frameworks, particularly regarding computational grap
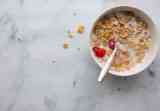
Serialization and deserialization of Python objects are key aspects of any non-trivial program. If you save something to a Python file, you do object serialization and deserialization if you read the configuration file, or if you respond to an HTTP request. In a sense, serialization and deserialization are the most boring things in the world. Who cares about all these formats and protocols? You want to persist or stream some Python objects and retrieve them in full at a later time. This is a great way to see the world on a conceptual level. However, on a practical level, the serialization scheme, format or protocol you choose may determine the speed, security, freedom of maintenance status, and other aspects of the program
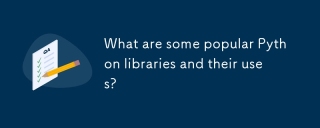
The article discusses popular Python libraries like NumPy, Pandas, Matplotlib, Scikit-learn, TensorFlow, Django, Flask, and Requests, detailing their uses in scientific computing, data analysis, visualization, machine learning, web development, and H
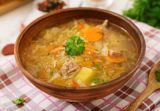
This tutorial builds upon the previous introduction to Beautiful Soup, focusing on DOM manipulation beyond simple tree navigation. We'll explore efficient search methods and techniques for modifying HTML structure. One common DOM search method is ex
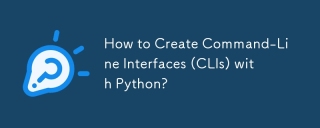
This article guides Python developers on building command-line interfaces (CLIs). It details using libraries like typer, click, and argparse, emphasizing input/output handling, and promoting user-friendly design patterns for improved CLI usability.


Hot AI Tools

Undresser.AI Undress
AI-powered app for creating realistic nude photos

AI Clothes Remover
Online AI tool for removing clothes from photos.

Undress AI Tool
Undress images for free

Clothoff.io
AI clothes remover

AI Hentai Generator
Generate AI Hentai for free.

Hot Article

Hot Tools

Atom editor mac version download
The most popular open source editor
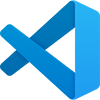
VSCode Windows 64-bit Download
A free and powerful IDE editor launched by Microsoft

MinGW - Minimalist GNU for Windows
This project is in the process of being migrated to osdn.net/projects/mingw, you can continue to follow us there. MinGW: A native Windows port of the GNU Compiler Collection (GCC), freely distributable import libraries and header files for building native Windows applications; includes extensions to the MSVC runtime to support C99 functionality. All MinGW software can run on 64-bit Windows platforms.

SublimeText3 Linux new version
SublimeText3 Linux latest version

mPDF
mPDF is a PHP library that can generate PDF files from UTF-8 encoded HTML. The original author, Ian Back, wrote mPDF to output PDF files "on the fly" from his website and handle different languages. It is slower than original scripts like HTML2FPDF and produces larger files when using Unicode fonts, but supports CSS styles etc. and has a lot of enhancements. Supports almost all languages, including RTL (Arabic and Hebrew) and CJK (Chinese, Japanese and Korean). Supports nested block-level elements (such as P, DIV),
