


Apply Multiple Functions to Multiple GroupBy Columns
Introduction
When working with grouped data, it is often necessary to apply multiple functions to multiple columns. The Pandas library provides several methods for achieving this, including the agg and apply methods. However, these methods have certain limitations and may not always meet specific use cases.
Using agg with a Dict
As mentioned in the question, it is possible to apply multiple functions to a groupby Series object using a dictionary:
grouped['D'].agg({'result1' : np.sum, 'result2' : np.mean})
This approach allows specifying the column names as keys and the corresponding functions as values. However, this only works for Series groupby objects. When applied to a groupby DataFrame, the dictionary keys are expected to be column names, not output column names.
Using agg with Lambda Functions
The question also explores using lambda functions within agg to perform operations based on other columns within the groupby object. This approach is suitable when your functions involve dependencies on other columns. While not explicitly supported by the agg method, it is possible to work around this limitation by manually specifying the column names as strings:
grouped.agg({'C_sum' : lambda x: x['C'].sum(), 'C_std': lambda x: x['C'].std(), 'D_sum' : lambda x: x['D'].sum()}, 'D_sumifC3': lambda x: x['D'][x['C'] == 3].sum(), ...)
This approach allows applying multiple functions to different columns, including those dependent on others. However, it can be verbose and requires careful handling of column names.
Using apply with a Custom Function
A more flexible approach is to use the apply method, which passes the entire group DataFrame to the provided function. This allows performing more complex operations and interactions between columns within the group:
def f(x): d = {} d['a_sum'] = x['a'].sum() d['a_max'] = x['a'].max() d['b_mean'] = x['b'].mean() d['c_d_prodsum'] = (x['c'] * x['d']).sum() return pd.Series(d, index=['a_sum', 'a_max', 'b_mean', 'c_d_prodsum']) df.groupby('group').apply(f)
By returning a Series with appropriately labeled columns, you can easily perform multiple calculations on the groupby DataFrame. This approach is more versatile and allows complex operations based on multiple columns.
Conclusion
Applying multiple functions to multiple grouped columns requires careful consideration of the data structure and the desired operations. The agg method is suitable for simple operations on Series objects, while the apply method offers greater flexibility when working with groupby DataFrames or performing complex calculations.
The above is the detailed content of How Can I Efficiently Apply Multiple Functions to Multiple GroupBy Columns in Pandas?. For more information, please follow other related articles on the PHP Chinese website!
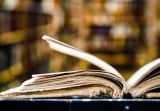
This tutorial demonstrates how to use Python to process the statistical concept of Zipf's law and demonstrates the efficiency of Python's reading and sorting large text files when processing the law. You may be wondering what the term Zipf distribution means. To understand this term, we first need to define Zipf's law. Don't worry, I'll try to simplify the instructions. Zipf's Law Zipf's law simply means: in a large natural language corpus, the most frequently occurring words appear about twice as frequently as the second frequent words, three times as the third frequent words, four times as the fourth frequent words, and so on. Let's look at an example. If you look at the Brown corpus in American English, you will notice that the most frequent word is "th
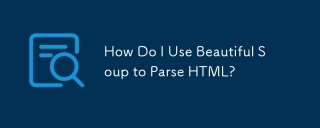
This article explains how to use Beautiful Soup, a Python library, to parse HTML. It details common methods like find(), find_all(), select(), and get_text() for data extraction, handling of diverse HTML structures and errors, and alternatives (Sel
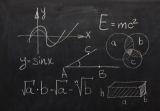
Python's statistics module provides powerful data statistical analysis capabilities to help us quickly understand the overall characteristics of data, such as biostatistics and business analysis. Instead of looking at data points one by one, just look at statistics such as mean or variance to discover trends and features in the original data that may be ignored, and compare large datasets more easily and effectively. This tutorial will explain how to calculate the mean and measure the degree of dispersion of the dataset. Unless otherwise stated, all functions in this module support the calculation of the mean() function instead of simply summing the average. Floating point numbers can also be used. import random import statistics from fracti
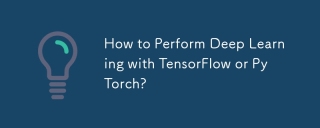
This article compares TensorFlow and PyTorch for deep learning. It details the steps involved: data preparation, model building, training, evaluation, and deployment. Key differences between the frameworks, particularly regarding computational grap
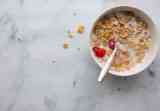
Serialization and deserialization of Python objects are key aspects of any non-trivial program. If you save something to a Python file, you do object serialization and deserialization if you read the configuration file, or if you respond to an HTTP request. In a sense, serialization and deserialization are the most boring things in the world. Who cares about all these formats and protocols? You want to persist or stream some Python objects and retrieve them in full at a later time. This is a great way to see the world on a conceptual level. However, on a practical level, the serialization scheme, format or protocol you choose may determine the speed, security, freedom of maintenance status, and other aspects of the program
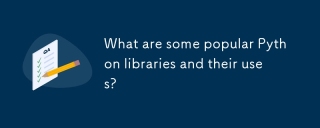
The article discusses popular Python libraries like NumPy, Pandas, Matplotlib, Scikit-learn, TensorFlow, Django, Flask, and Requests, detailing their uses in scientific computing, data analysis, visualization, machine learning, web development, and H
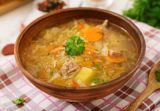
This tutorial builds upon the previous introduction to Beautiful Soup, focusing on DOM manipulation beyond simple tree navigation. We'll explore efficient search methods and techniques for modifying HTML structure. One common DOM search method is ex
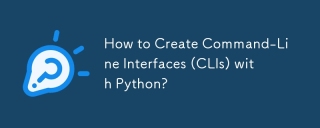
This article guides Python developers on building command-line interfaces (CLIs). It details using libraries like typer, click, and argparse, emphasizing input/output handling, and promoting user-friendly design patterns for improved CLI usability.


Hot AI Tools

Undresser.AI Undress
AI-powered app for creating realistic nude photos

AI Clothes Remover
Online AI tool for removing clothes from photos.

Undress AI Tool
Undress images for free

Clothoff.io
AI clothes remover

AI Hentai Generator
Generate AI Hentai for free.

Hot Article

Hot Tools

Atom editor mac version download
The most popular open source editor
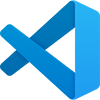
VSCode Windows 64-bit Download
A free and powerful IDE editor launched by Microsoft

MinGW - Minimalist GNU for Windows
This project is in the process of being migrated to osdn.net/projects/mingw, you can continue to follow us there. MinGW: A native Windows port of the GNU Compiler Collection (GCC), freely distributable import libraries and header files for building native Windows applications; includes extensions to the MSVC runtime to support C99 functionality. All MinGW software can run on 64-bit Windows platforms.

SublimeText3 Linux new version
SublimeText3 Linux latest version

mPDF
mPDF is a PHP library that can generate PDF files from UTF-8 encoded HTML. The original author, Ian Back, wrote mPDF to output PDF files "on the fly" from his website and handle different languages. It is slower than original scripts like HTML2FPDF and produces larger files when using Unicode fonts, but supports CSS styles etc. and has a lot of enhancements. Supports almost all languages, including RTL (Arabic and Hebrew) and CJK (Chinese, Japanese and Korean). Supports nested block-level elements (such as P, DIV),
