When it comes to running multiple tasks simultaneously in Python, the concurrent.futures module is a powerful and straightforward tool. In this article, we'll explore how to use ThreadPoolExecutor to execute tasks in parallel, along with practical examples.
Why Use ThreadPoolExecutor?
In Python, threads are perfect for tasks where I/O operations dominate, such as network calls or file read/write operations. With ThreadPoolExecutor, you can:
- Run multiple tasks concurrently without manually managing threads.
- Limit the number of active threads to avoid overwhelming your system.
- Easily collect results using its intuitive API.
Example: Running Tasks in Parallel
Let's look at a simple example to understand the concept.
The Code
from concurrent.futures import ThreadPoolExecutor import time # Function simulating a task def task(n): print(f"Task {n} started") time.sleep(2) # Simulates a long-running task print(f"Task {n} finished") return f"Result of task {n}" # Using ThreadPoolExecutor def execute_tasks(): tasks = [1, 2, 3, 4, 5] # List of tasks results = [] # Create a thread pool with 3 simultaneous threads with ThreadPoolExecutor(max_workers=3) as executor: # Execute tasks in parallel results = executor.map(task, tasks) return list(results) if __name__ == "__main__": results = execute_tasks() print("All results:", results)
Expected Output
When you run this code, you'll see something like this (in a somewhat parallel order):
Task 1 started Task 2 started Task 3 started Task 1 finished Task 4 started Task 2 finished Task 5 started Task 3 finished Task 4 finished Task 5 finished All results: ['Result of task 1', 'Result of task 2', 'Result of task 3', 'Result of task 4', 'Result of task 5']
Tasks 1, 2, and 3 start simultaneously because max_workers=3. Other tasks (4 and 5) wait until threads are available.
When to Use It?
Typical Use Cases:
- Fetching data from APIs: Load multiple URLs concurrently.
- File processing: Read, write, or transform multiple files simultaneously.
- Task automation: Launch multiple scripts or commands in parallel.
Best Practices
-
Limit the number of threads:
- Too many threads can overload your CPU or create bottlenecks.
-
Handle exceptions:
- If one task fails, it can affect the entire pool. Catch exceptions in your functions.
-
Use ProcessPoolExecutor for CPU-bound tasks:
- Threads are not optimal for heavy computations due to Python's Global Interpreter Lock (GIL).
Advanced Example: Fetching URLs in Parallel
Here's a real-world example: fetching multiple URLs in parallel.
import requests from concurrent.futures import ThreadPoolExecutor # Function to fetch a URL def fetch_url(url): try: response = requests.get(url) return f"URL: {url}, Status: {response.status_code}" except Exception as e: return f"URL: {url}, Error: {e}" # List of URLs to fetch urls = [ "https://example.com", "https://httpbin.org/get", "https://jsonplaceholder.typicode.com/posts", "https://invalid-url.com" ] def fetch_all_urls(urls): with ThreadPoolExecutor(max_workers=4) as executor: results = executor.map(fetch_url, urls) return list(results) if __name__ == "__main__": results = fetch_all_urls(urls) for result in results: print(result)
Conclusion
ThreadPoolExecutor simplifies thread management in Python and is ideal for speeding up I/O-bound tasks. With just a few lines of code, you can parallelize operations and save valuable time.
The above is the detailed content of # Boost Your Python Tasks with `ThreadPoolExecutor`. For more information, please follow other related articles on the PHP Chinese website!
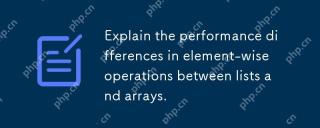
Arraysarebetterforelement-wiseoperationsduetofasteraccessandoptimizedimplementations.1)Arrayshavecontiguousmemoryfordirectaccess,enhancingperformance.2)Listsareflexiblebutslowerduetopotentialdynamicresizing.3)Forlargedatasets,arrays,especiallywithlib
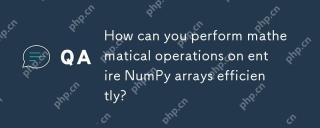
Mathematical operations of the entire array in NumPy can be efficiently implemented through vectorized operations. 1) Use simple operators such as addition (arr 2) to perform operations on arrays. 2) NumPy uses the underlying C language library, which improves the computing speed. 3) You can perform complex operations such as multiplication, division, and exponents. 4) Pay attention to broadcast operations to ensure that the array shape is compatible. 5) Using NumPy functions such as np.sum() can significantly improve performance.
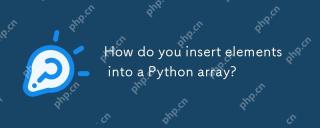
In Python, there are two main methods for inserting elements into a list: 1) Using the insert(index, value) method, you can insert elements at the specified index, but inserting at the beginning of a large list is inefficient; 2) Using the append(value) method, add elements at the end of the list, which is highly efficient. For large lists, it is recommended to use append() or consider using deque or NumPy arrays to optimize performance.
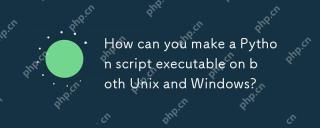
TomakeaPythonscriptexecutableonbothUnixandWindows:1)Addashebangline(#!/usr/bin/envpython3)andusechmod xtomakeitexecutableonUnix.2)OnWindows,ensurePythonisinstalledandassociatedwith.pyfiles,oruseabatchfile(run.bat)torunthescript.
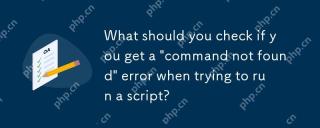
When encountering a "commandnotfound" error, the following points should be checked: 1. Confirm that the script exists and the path is correct; 2. Check file permissions and use chmod to add execution permissions if necessary; 3. Make sure the script interpreter is installed and in PATH; 4. Verify that the shebang line at the beginning of the script is correct. Doing so can effectively solve the script operation problem and ensure the coding process is smooth.
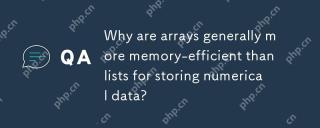
Arraysaregenerallymorememory-efficientthanlistsforstoringnumericaldataduetotheirfixed-sizenatureanddirectmemoryaccess.1)Arraysstoreelementsinacontiguousblock,reducingoverheadfrompointersormetadata.2)Lists,oftenimplementedasdynamicarraysorlinkedstruct
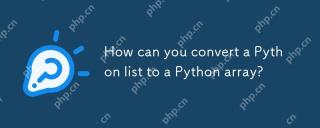
ToconvertaPythonlisttoanarray,usethearraymodule:1)Importthearraymodule,2)Createalist,3)Usearray(typecode,list)toconvertit,specifyingthetypecodelike'i'forintegers.Thisconversionoptimizesmemoryusageforhomogeneousdata,enhancingperformanceinnumericalcomp
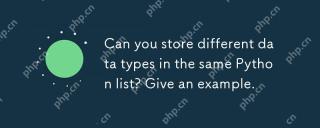
Python lists can store different types of data. The example list contains integers, strings, floating point numbers, booleans, nested lists, and dictionaries. List flexibility is valuable in data processing and prototyping, but it needs to be used with caution to ensure the readability and maintainability of the code.


Hot AI Tools

Undresser.AI Undress
AI-powered app for creating realistic nude photos

AI Clothes Remover
Online AI tool for removing clothes from photos.

Undress AI Tool
Undress images for free

Clothoff.io
AI clothes remover

Video Face Swap
Swap faces in any video effortlessly with our completely free AI face swap tool!

Hot Article

Hot Tools

Notepad++7.3.1
Easy-to-use and free code editor

Atom editor mac version download
The most popular open source editor
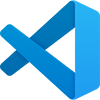
VSCode Windows 64-bit Download
A free and powerful IDE editor launched by Microsoft
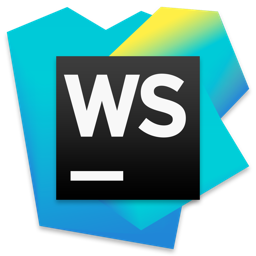
WebStorm Mac version
Useful JavaScript development tools
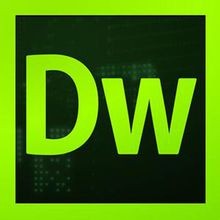
Dreamweaver CS6
Visual web development tools
