Ever feel overwhelmed while learning something new? Like you're drowning in information but not actually absorbing anything? We've all been there. Wouldn't it be awesome to have a personalized study companion that understands your level and explains things in a way that clicks? That's exactly what we're going to build together.
This tutorial will show you how to combine the BotHub API with PyQt5 to create an interactive and adaptable learning tool. It's not just another chatbot; it's more like a personal tutor, available 24/7.
Prepping Your Workspace
Before we start building, let's gather our tools. We'll need a few key Python libraries:
import os import datetime import json from dataclasses import dataclass from typing import List, Dict from openai import OpenAI from dotenv import load_dotenv from PyQt5.QtCore import Qt, QThread, pyqtSignal from PyQt5.QtGui import QMovie from PyQt5.QtWidgets import (QApplication, QWidget, QVBoxLayout, QHBoxLayout, QLabel, QLineEdit, QTextEdit, QRadioButton, QButtonGroup, QPushButton, QGroupBox, QListWidget, QListWidgetItem, QTabWidget, QFileDialog, QComboBox, QCheckBox, QMessageBox, QDialogButtonBox, QSpinBox, QFormLayout, QDialog, QDateEdit)
Think of these libraries as different parts of your toolkit. Some handle the basics, like file management (os), timekeeping (datetime), and data handling (json). Others, like dataclasses and typing, help us write clean, organized code. The real magic happens with openai, which lets us tap into the power of AI. dotenv keeps our sensitive information (like API keys) secure. And finally, PyQt5 helps us create a beautiful and intuitive user interface.
Crafting User Requests
To communicate with our AI, we'll create a UserRequest class. This helps organize the information the user provides:
@dataclass class UserRequest: query: str user_level: str preferences: Dict
Using the handy @dataclass decorator, we define three key pieces of information: the user's query (what they're asking), their user_level (beginner, intermediate, or advanced), and their preferences (like how long they want the response to be). This neatly packages everything into a single object.
Remembering User Sessions
To make the learning experience truly personalized, we need to remember what the user has done and how they like to learn. That's where the UserSession class comes in:
class UserSession: def __init__(self): self.history: List[Dict] = [] self.preferences: Dict = {} self.level: str = "beginner" def add_to_history(self, query, response): self.history.append({"query": query, "response": response, "timestamp": datetime.datetime.now().isoformat()}) def update_preferences(self, new_preferences): self.preferences.update(new_preferences)
A UserSession keeps track of the conversation history, the user's preferences, and their current level. It's like having a dedicated assistant who remembers everything and adapts to the user's needs.
The Brains of the Operation: EducationalAssistant
The EducationalAssistant class is the heart of our application. It's responsible for interacting with the BotHub API:
class EducationalAssistant: def __init__(self): load_dotenv() self.client = OpenAI(api_key=os.getenv('BOTHUB_API_KEY'), base_url='https://bothub.chat/api/v2/openai/v1') self.session = UserSession() def generate_prompt(self, request): prompt = f"""As an educational assistant, provide a response for a {request.user_level} level student. Query: {request.query}\n""" if request.preferences: prompt += "Consider these preferences:\n" for key, value in request.preferences.items(): if key == "response_length": prompt += f"Desired Length: Approximately {value} words\n" elif key == "include_examples" and value: prompt += "Include Examples: Yes\n" else: prompt += f"{key.capitalize()}: {value}\n" prompt += "Please provide a detailed explanation." return prompt def generate_text_response(self, request): try: response = self.client.chat.completions.create( model="claude-3.5-sonnet", // u can use any model in "Models available" on BotHub messages=[ {"role": "system", "content": "You are an educational assistant."}, {"role": "user", "content": self.generate_prompt(request)} ] ) return response.choices[0].message.content except Exception as e: return f"Error generating text response: {e}"
This class handles a few crucial tasks. First, it initializes the connection to BotHub using your API key (we talked about it previously). It also sets up a UserSession to keep track of the interaction. The generate_prompt method takes the user's request and transforms it into a prompt the API can understand. Finally, generate_text_response sends the prompt to the API and retrieves the AI-generated answer.
Smooth and Responsive: GenerateResponseThread
To avoid making the user wait while the AI is thinking, we'll use a separate thread for API calls:
import os import datetime import json from dataclasses import dataclass from typing import List, Dict from openai import OpenAI from dotenv import load_dotenv from PyQt5.QtCore import Qt, QThread, pyqtSignal from PyQt5.QtGui import QMovie from PyQt5.QtWidgets import (QApplication, QWidget, QVBoxLayout, QHBoxLayout, QLabel, QLineEdit, QTextEdit, QRadioButton, QButtonGroup, QPushButton, QGroupBox, QListWidget, QListWidgetItem, QTabWidget, QFileDialog, QComboBox, QCheckBox, QMessageBox, QDialogButtonBox, QSpinBox, QFormLayout, QDialog, QDateEdit)
This GenerateResponseThread, based on PyQt5's QThread, runs the API request in the background, ensuring that the user interface remains responsive.
Personalizing the Experience
Everyone learns differently. To cater to individual preferences, we'll create a PreferencesDialog:
@dataclass class UserRequest: query: str user_level: str preferences: Dict
This dialog allows users to customize settings like the AI's tone of voice, the desired response length, and whether to include examples. This level of customization ensures a more engaging and effective learning experience.
Building the Interface
Finally, let's create the user interface with the EducationalAssistantGUI class:
class UserSession: def __init__(self): self.history: List[Dict] = [] self.preferences: Dict = {} self.level: str = "beginner" def add_to_history(self, query, response): self.history.append({"query": query, "response": response, "timestamp": datetime.datetime.now().isoformat()}) def update_preferences(self, new_preferences): self.preferences.update(new_preferences)
This class builds the main window, which includes two tabs: "Chat" and "History." The "Chat" tab allows users to enter their queries, select their level, and see the AI's responses. The "History" tab displays past conversations, offering search and export functionalities.
Launching Your AI Study Buddy
Now, let's bring our creation to life:
class EducationalAssistant: def __init__(self): load_dotenv() self.client = OpenAI(api_key=os.getenv('BOTHUB_API_KEY'), base_url='https://bothub.chat/api/v2/openai/v1') self.session = UserSession() def generate_prompt(self, request): prompt = f"""As an educational assistant, provide a response for a {request.user_level} level student. Query: {request.query}\n""" if request.preferences: prompt += "Consider these preferences:\n" for key, value in request.preferences.items(): if key == "response_length": prompt += f"Desired Length: Approximately {value} words\n" elif key == "include_examples" and value: prompt += "Include Examples: Yes\n" else: prompt += f"{key.capitalize()}: {value}\n" prompt += "Please provide a detailed explanation." return prompt def generate_text_response(self, request): try: response = self.client.chat.completions.create( model="claude-3.5-sonnet", // u can use any model in "Models available" on BotHub messages=[ {"role": "system", "content": "You are an educational assistant."}, {"role": "user", "content": self.generate_prompt(request)} ] ) return response.choices[0].message.content except Exception as e: return f"Error generating text response: {e}"
Congratulations! You've built your own personalized AI learning assistant.
Now that you have a working app, think about how you could make it even better! The BotHub API offers a lot of flexibility. Instead of just text responses, you could integrate image generation or speech transcription. BotHub also gives you access to multiple AI models, allowing you to choose the best one for different tasks. Imagine your assistant being able to summarize complex topics, translate languages, or even generate practice quizzes! The possibilities are vast. You've built a solid foundation; now go forth and explore!
The above is the detailed content of Tutorial: Create Your Own AI Study Buddy. For more information, please follow other related articles on the PHP Chinese website!
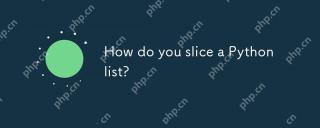
SlicingaPythonlistisdoneusingthesyntaxlist[start:stop:step].Here'showitworks:1)Startistheindexofthefirstelementtoinclude.2)Stopistheindexofthefirstelementtoexclude.3)Stepistheincrementbetweenelements.It'susefulforextractingportionsoflistsandcanuseneg
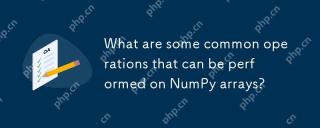
NumPyallowsforvariousoperationsonarrays:1)Basicarithmeticlikeaddition,subtraction,multiplication,anddivision;2)Advancedoperationssuchasmatrixmultiplication;3)Element-wiseoperationswithoutexplicitloops;4)Arrayindexingandslicingfordatamanipulation;5)Ag
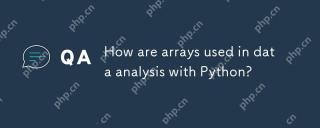
ArraysinPython,particularlythroughNumPyandPandas,areessentialfordataanalysis,offeringspeedandefficiency.1)NumPyarraysenableefficienthandlingoflargedatasetsandcomplexoperationslikemovingaverages.2)PandasextendsNumPy'scapabilitieswithDataFramesforstruc
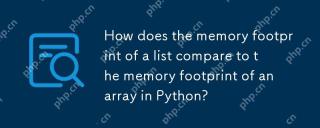
ListsandNumPyarraysinPythonhavedifferentmemoryfootprints:listsaremoreflexiblebutlessmemory-efficient,whileNumPyarraysareoptimizedfornumericaldata.1)Listsstorereferencestoobjects,withoverheadaround64byteson64-bitsystems.2)NumPyarraysstoredatacontiguou
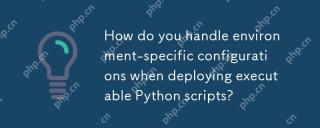
ToensurePythonscriptsbehavecorrectlyacrossdevelopment,staging,andproduction,usethesestrategies:1)Environmentvariablesforsimplesettings,2)Configurationfilesforcomplexsetups,and3)Dynamicloadingforadaptability.Eachmethodoffersuniquebenefitsandrequiresca
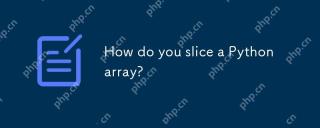
The basic syntax for Python list slicing is list[start:stop:step]. 1.start is the first element index included, 2.stop is the first element index excluded, and 3.step determines the step size between elements. Slices are not only used to extract data, but also to modify and invert lists.
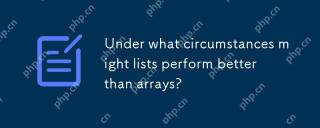
Listsoutperformarraysin:1)dynamicsizingandfrequentinsertions/deletions,2)storingheterogeneousdata,and3)memoryefficiencyforsparsedata,butmayhaveslightperformancecostsincertainoperations.
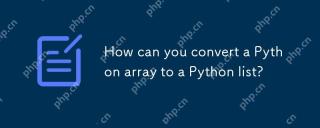
ToconvertaPythonarraytoalist,usethelist()constructororageneratorexpression.1)Importthearraymoduleandcreateanarray.2)Uselist(arr)or[xforxinarr]toconvertittoalist,consideringperformanceandmemoryefficiencyforlargedatasets.


Hot AI Tools

Undresser.AI Undress
AI-powered app for creating realistic nude photos

AI Clothes Remover
Online AI tool for removing clothes from photos.

Undress AI Tool
Undress images for free

Clothoff.io
AI clothes remover

Video Face Swap
Swap faces in any video effortlessly with our completely free AI face swap tool!

Hot Article

Hot Tools
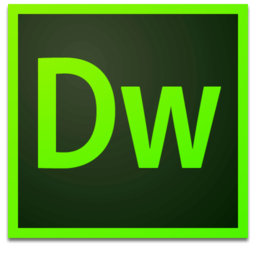
Dreamweaver Mac version
Visual web development tools
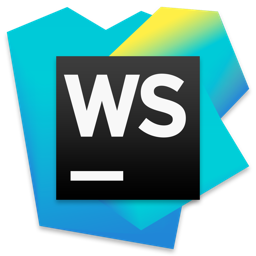
WebStorm Mac version
Useful JavaScript development tools

MinGW - Minimalist GNU for Windows
This project is in the process of being migrated to osdn.net/projects/mingw, you can continue to follow us there. MinGW: A native Windows port of the GNU Compiler Collection (GCC), freely distributable import libraries and header files for building native Windows applications; includes extensions to the MSVC runtime to support C99 functionality. All MinGW software can run on 64-bit Windows platforms.
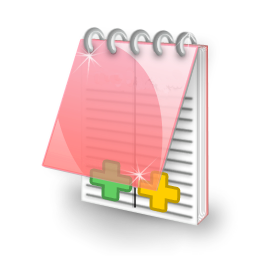
EditPlus Chinese cracked version
Small size, syntax highlighting, does not support code prompt function

SAP NetWeaver Server Adapter for Eclipse
Integrate Eclipse with SAP NetWeaver application server.
