


One Hot Encoding in Python: Handling Categorical Features in Machine Learning
One hot encoding is a technique used in machine learning to transform categorical variables into binary vectors. It is often used when dealing with categorical variables that have a high number of unique values.
Is One Hot Encoding Necessary for Classification?
Yes, one hot encoding is typically required when using classifiers that expect numerical input. Categorical variables are not inherently numerical, and classifiers cannot directly interpret them. One hot encoding converts categorical variables into binary vectors that represent the presence or absence of each unique value.
Step-by-Step One Hot Encoding in Python
Approach 1: Using Pandas pd.get_dummies
This method is suitable for small datasets with a limited number of unique values.
import pandas as pd # Create a pandas Series with categorical data s = pd.Series(['a', 'b', 'c', 'a']) # One hot encode the Series one_hot = pd.get_dummies(s) print(one_hot)
Approach 2: Using Scikit-Learn
Scikit-learn's OneHotEncoder offers more flexibility and control over the encoding process.
from sklearn.preprocessing import OneHotEncoder # Create a numpy array with categorical data data = np.array([['a', 'b', 'c'], ['a', 'c', 'b']]) # Create an encoder enc = OneHotEncoder() # Fit the encoder to the data enc.fit(data) # Transform the data one_hot = enc.transform(data).toarray() print(one_hot)
Resolving the Stuck Encoding Issue
The third part of your code where one hot encoding gets stuck may be due to the following reasons:
- Memory constraints: One hot encoding can result in a significant increase in the number of features, especially for high-cardinality categorical variables. This may lead to memory issues.
- Computational complexity: The time complexity of one hot encoding is O(N * C), where N is the number of rows and C is the number of unique values. This can be computationally intensive for large datasets.
To address these issues, you can:
- Reduce the number of unique values: Consider merging or aggregating categorical variables with similar values.
- Use sparse encoding: Sparse encoding represents binary vectors as lists of indices rather than full vectors. This can save memory and speed up computation.
- Use incremental/partial encoding: Encode data in batches to avoid memory exhaustion.
- Consider using libraries that optimize encoding: Libraries like Category Encoders provide efficient and scalable encoding algorithms.
The above is the detailed content of Is One Hot Encoding Essential for Machine Learning Classification?. For more information, please follow other related articles on the PHP Chinese website!
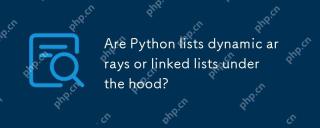
Pythonlistsareimplementedasdynamicarrays,notlinkedlists.1)Theyarestoredincontiguousmemoryblocks,whichmayrequirereallocationwhenappendingitems,impactingperformance.2)Linkedlistswouldofferefficientinsertions/deletionsbutslowerindexedaccess,leadingPytho
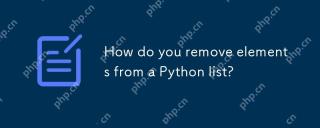
Pythonoffersfourmainmethodstoremoveelementsfromalist:1)remove(value)removesthefirstoccurrenceofavalue,2)pop(index)removesandreturnsanelementataspecifiedindex,3)delstatementremoveselementsbyindexorslice,and4)clear()removesallitemsfromthelist.Eachmetho
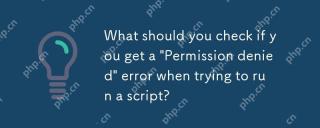
Toresolvea"Permissiondenied"errorwhenrunningascript,followthesesteps:1)Checkandadjustthescript'spermissionsusingchmod xmyscript.shtomakeitexecutable.2)Ensurethescriptislocatedinadirectorywhereyouhavewritepermissions,suchasyourhomedirectory.
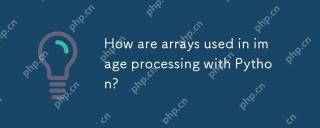
ArraysarecrucialinPythonimageprocessingastheyenableefficientmanipulationandanalysisofimagedata.1)ImagesareconvertedtoNumPyarrays,withgrayscaleimagesas2Darraysandcolorimagesas3Darrays.2)Arraysallowforvectorizedoperations,enablingfastadjustmentslikebri
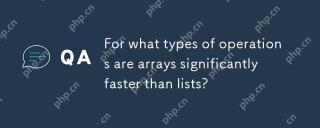
Arraysaresignificantlyfasterthanlistsforoperationsbenefitingfromdirectmemoryaccessandfixed-sizestructures.1)Accessingelements:Arraysprovideconstant-timeaccessduetocontiguousmemorystorage.2)Iteration:Arraysleveragecachelocalityforfasteriteration.3)Mem
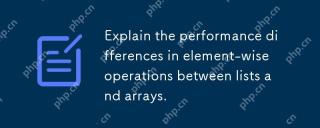
Arraysarebetterforelement-wiseoperationsduetofasteraccessandoptimizedimplementations.1)Arrayshavecontiguousmemoryfordirectaccess,enhancingperformance.2)Listsareflexiblebutslowerduetopotentialdynamicresizing.3)Forlargedatasets,arrays,especiallywithlib
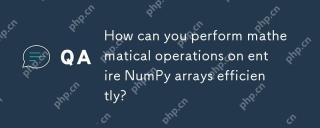
Mathematical operations of the entire array in NumPy can be efficiently implemented through vectorized operations. 1) Use simple operators such as addition (arr 2) to perform operations on arrays. 2) NumPy uses the underlying C language library, which improves the computing speed. 3) You can perform complex operations such as multiplication, division, and exponents. 4) Pay attention to broadcast operations to ensure that the array shape is compatible. 5) Using NumPy functions such as np.sum() can significantly improve performance.
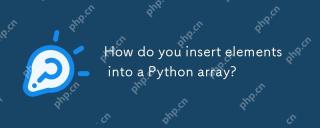
In Python, there are two main methods for inserting elements into a list: 1) Using the insert(index, value) method, you can insert elements at the specified index, but inserting at the beginning of a large list is inefficient; 2) Using the append(value) method, add elements at the end of the list, which is highly efficient. For large lists, it is recommended to use append() or consider using deque or NumPy arrays to optimize performance.


Hot AI Tools

Undresser.AI Undress
AI-powered app for creating realistic nude photos

AI Clothes Remover
Online AI tool for removing clothes from photos.

Undress AI Tool
Undress images for free

Clothoff.io
AI clothes remover

Video Face Swap
Swap faces in any video effortlessly with our completely free AI face swap tool!

Hot Article

Hot Tools
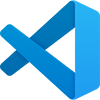
VSCode Windows 64-bit Download
A free and powerful IDE editor launched by Microsoft

Safe Exam Browser
Safe Exam Browser is a secure browser environment for taking online exams securely. This software turns any computer into a secure workstation. It controls access to any utility and prevents students from using unauthorized resources.

MantisBT
Mantis is an easy-to-deploy web-based defect tracking tool designed to aid in product defect tracking. It requires PHP, MySQL and a web server. Check out our demo and hosting services.

SAP NetWeaver Server Adapter for Eclipse
Integrate Eclipse with SAP NetWeaver application server.

DVWA
Damn Vulnerable Web App (DVWA) is a PHP/MySQL web application that is very vulnerable. Its main goals are to be an aid for security professionals to test their skills and tools in a legal environment, to help web developers better understand the process of securing web applications, and to help teachers/students teach/learn in a classroom environment Web application security. The goal of DVWA is to practice some of the most common web vulnerabilities through a simple and straightforward interface, with varying degrees of difficulty. Please note that this software
