


Why is BLAS so much faster for matrix-matrix multiplication than my custom implementation?
Unveiling the Performance Secrets of BLAS
Matrix-matrix multiplications are fundamental operations in linear algebra, and their efficiency directly impacts the speed of scientific computing tasks. Curious about the remarkable performance of BLAS (Basic Linear Algebra Subprograms), an implementation of these multiplications, a user compared it to their own custom implementation and encountered a significant disparity in execution time.
Understanding the Performance Gap
To delve into the reasons behind this performance gap, we must consider the different levels of BLAS:
- Level 1: Vector operations that benefit from vectorization through SIMD (Single Instruction Multiple Data).
- Level 2: Matrix-vector operations that can exploit parallelism in multiprocessor architectures with shared memory.
- Level 3: Matrix-matrix operations that perform an enormous number of operations on a limited amount of data.
Level 3 functions, like matrix-matrix multiplication, are particularly sensitive to cache hierarchy optimization. By reducing data movement between cache levels, cache-optimized implementations dramatically improve performance.
Factors Enhancing BLAS Performance
Besides cache optimization, other factors contribute to BLAS's superior performance:
- Optimized Compilers: While compilers play a role, they are not the primary reason for BLAS's efficiency.
- Efficient Algorithms: BLAS implementations typically employ established matrix multiplication algorithms, such as the standard triple-loop approach. Algorithms like the Strassen algorithm or the Coppersmith-Winograd algorithm are generally not used in BLAS due to their numerical instability or high computational overhead for large matrices.
State-of-the-Art BLAS Implementations
Modern BLAS implementations, such as BLIS, exemplify the latest advancements in performance optimization. BLIS provides a fully optimized matrix-matrix product that demonstrates exceptional speed and scalability.
By understanding the intricate architecture of BLAS, the user can appreciate the challenges and complexities faced in accelerating matrix-matrix multiplications. The combination of cache optimization, efficient algorithms, and ongoing research ensures that BLAS remains the cornerstone of high-performance scientific computing.
The above is the detailed content of Why is BLAS so much faster for matrix-matrix multiplication than my custom implementation?. For more information, please follow other related articles on the PHP Chinese website!
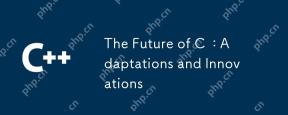
The future of C will focus on parallel computing, security, modularization and AI/machine learning: 1) Parallel computing will be enhanced through features such as coroutines; 2) Security will be improved through stricter type checking and memory management mechanisms; 3) Modulation will simplify code organization and compilation; 4) AI and machine learning will prompt C to adapt to new needs, such as numerical computing and GPU programming support.
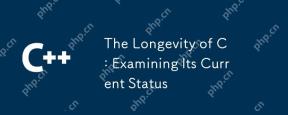
C is still important in modern programming because of its efficient, flexible and powerful nature. 1)C supports object-oriented programming, suitable for system programming, game development and embedded systems. 2) Polymorphism is the highlight of C, allowing the call to derived class methods through base class pointers or references to enhance the flexibility and scalability of the code.
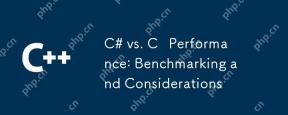
The performance differences between C# and C are mainly reflected in execution speed and resource management: 1) C usually performs better in numerical calculations and string operations because it is closer to hardware and has no additional overhead such as garbage collection; 2) C# is more concise in multi-threaded programming, but its performance is slightly inferior to C; 3) Which language to choose should be determined based on project requirements and team technology stack.
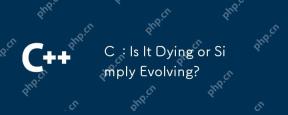
C isnotdying;it'sevolving.1)C remainsrelevantduetoitsversatilityandefficiencyinperformance-criticalapplications.2)Thelanguageiscontinuouslyupdated,withC 20introducingfeatureslikemodulesandcoroutinestoimproveusabilityandperformance.3)Despitechallen
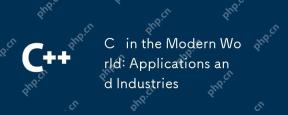
C is widely used and important in the modern world. 1) In game development, C is widely used for its high performance and polymorphism, such as UnrealEngine and Unity. 2) In financial trading systems, C's low latency and high throughput make it the first choice, suitable for high-frequency trading and real-time data analysis.
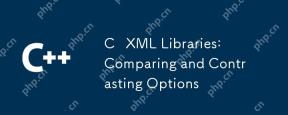
There are four commonly used XML libraries in C: TinyXML-2, PugiXML, Xerces-C, and RapidXML. 1.TinyXML-2 is suitable for environments with limited resources, lightweight but limited functions. 2. PugiXML is fast and supports XPath query, suitable for complex XML structures. 3.Xerces-C is powerful, supports DOM and SAX resolution, and is suitable for complex processing. 4. RapidXML focuses on performance and parses extremely fast, but does not support XPath queries.
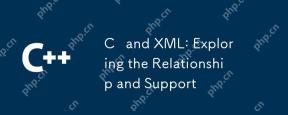
C interacts with XML through third-party libraries (such as TinyXML, Pugixml, Xerces-C). 1) Use the library to parse XML files and convert them into C-processable data structures. 2) When generating XML, convert the C data structure to XML format. 3) In practical applications, XML is often used for configuration files and data exchange to improve development efficiency.
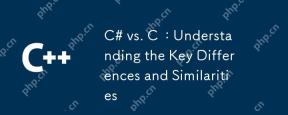
The main differences between C# and C are syntax, performance and application scenarios. 1) The C# syntax is more concise, supports garbage collection, and is suitable for .NET framework development. 2) C has higher performance and requires manual memory management, which is often used in system programming and game development.


Hot AI Tools

Undresser.AI Undress
AI-powered app for creating realistic nude photos

AI Clothes Remover
Online AI tool for removing clothes from photos.

Undress AI Tool
Undress images for free

Clothoff.io
AI clothes remover

Video Face Swap
Swap faces in any video effortlessly with our completely free AI face swap tool!

Hot Article

Hot Tools
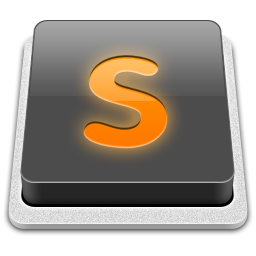
SublimeText3 Mac version
God-level code editing software (SublimeText3)

DVWA
Damn Vulnerable Web App (DVWA) is a PHP/MySQL web application that is very vulnerable. Its main goals are to be an aid for security professionals to test their skills and tools in a legal environment, to help web developers better understand the process of securing web applications, and to help teachers/students teach/learn in a classroom environment Web application security. The goal of DVWA is to practice some of the most common web vulnerabilities through a simple and straightforward interface, with varying degrees of difficulty. Please note that this software
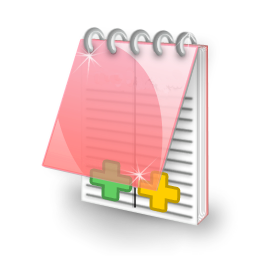
EditPlus Chinese cracked version
Small size, syntax highlighting, does not support code prompt function

SecLists
SecLists is the ultimate security tester's companion. It is a collection of various types of lists that are frequently used during security assessments, all in one place. SecLists helps make security testing more efficient and productive by conveniently providing all the lists a security tester might need. List types include usernames, passwords, URLs, fuzzing payloads, sensitive data patterns, web shells, and more. The tester can simply pull this repository onto a new test machine and he will have access to every type of list he needs.
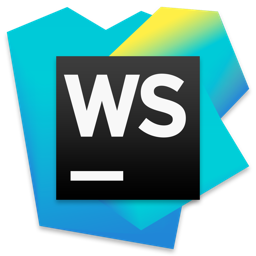
WebStorm Mac version
Useful JavaScript development tools
