


Overlapping Annotations in Matplotlib
Matplotlib visualizations often encounter the issue of overlapping annotations, leading to cluttered and difficult-to-interpret graphs. This article provides a comprehensive solution to address this challenge.
The Herausforderung
Overlapping annotations arise when multiple annotations share the same screen space, creating visual clutter. In the provided code, the annotation text for data points tends to overlap, especially in denser regions of the graph.
The Lösung
To avoid overlapping annotations, the adjustText library, written by Phlya, offers a simple and effective solution. This library automatically adjusts the position of annotations to minimize overlaps while maintaining readability.
Using adjustText
The following code snippet demonstrates how to use adjustText to optimize annotation placement in the provided example:
<code class="python">import matplotlib.pyplot as plt from adjustText import adjust_text # ... (code to generate the data and plot remain the same as before) ... plt.xlabel("Proportional Euclidean Distance") plt.ylabel("Percentage Timewindows Attended") plt.title("Test plot") texts = [x for (x,y,z) in together] eucs = [y for (x,y,z) in together] covers = [z for (x,y,z) in together] p1 = plt.plot(eucs,covers,color="black", alpha=0.5) texts = [] for x, y, s in zip(eucs, covers, text): texts.append(plt.text(x, y, s)) adjust_text(texts, only_move={'points':'y', 'texts':'y'}, arrowprops=dict(arrowstyle="->", color='r', lw=0.5)) plt.show()</code>
Customizing Text Placement
adjustText offers various customization options to fine-tune the placement of annotations. For instance, it allows you to control which elements are movable (only_move parameter), the alignment of annotations, and the strength of repulsion between text objects.
By experimenting with these parameters, you can achieve optimal text placement that maximizes clarity and visual appeal in your Matplotlib graphs without the worry of overlapping annotations.
The above is the detailed content of How can I prevent overlapping annotations in Matplotlib visualizations?. For more information, please follow other related articles on the PHP Chinese website!
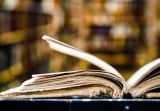
This tutorial demonstrates how to use Python to process the statistical concept of Zipf's law and demonstrates the efficiency of Python's reading and sorting large text files when processing the law. You may be wondering what the term Zipf distribution means. To understand this term, we first need to define Zipf's law. Don't worry, I'll try to simplify the instructions. Zipf's Law Zipf's law simply means: in a large natural language corpus, the most frequently occurring words appear about twice as frequently as the second frequent words, three times as the third frequent words, four times as the fourth frequent words, and so on. Let's look at an example. If you look at the Brown corpus in American English, you will notice that the most frequent word is "th
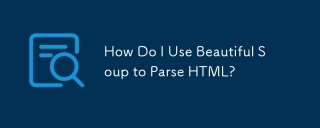
This article explains how to use Beautiful Soup, a Python library, to parse HTML. It details common methods like find(), find_all(), select(), and get_text() for data extraction, handling of diverse HTML structures and errors, and alternatives (Sel
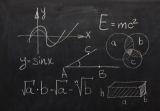
Python's statistics module provides powerful data statistical analysis capabilities to help us quickly understand the overall characteristics of data, such as biostatistics and business analysis. Instead of looking at data points one by one, just look at statistics such as mean or variance to discover trends and features in the original data that may be ignored, and compare large datasets more easily and effectively. This tutorial will explain how to calculate the mean and measure the degree of dispersion of the dataset. Unless otherwise stated, all functions in this module support the calculation of the mean() function instead of simply summing the average. Floating point numbers can also be used. import random import statistics from fracti
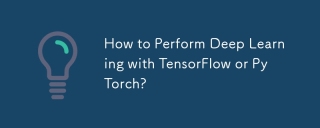
This article compares TensorFlow and PyTorch for deep learning. It details the steps involved: data preparation, model building, training, evaluation, and deployment. Key differences between the frameworks, particularly regarding computational grap
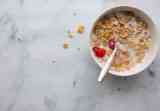
Serialization and deserialization of Python objects are key aspects of any non-trivial program. If you save something to a Python file, you do object serialization and deserialization if you read the configuration file, or if you respond to an HTTP request. In a sense, serialization and deserialization are the most boring things in the world. Who cares about all these formats and protocols? You want to persist or stream some Python objects and retrieve them in full at a later time. This is a great way to see the world on a conceptual level. However, on a practical level, the serialization scheme, format or protocol you choose may determine the speed, security, freedom of maintenance status, and other aspects of the program
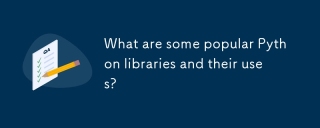
The article discusses popular Python libraries like NumPy, Pandas, Matplotlib, Scikit-learn, TensorFlow, Django, Flask, and Requests, detailing their uses in scientific computing, data analysis, visualization, machine learning, web development, and H
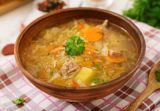
This tutorial builds upon the previous introduction to Beautiful Soup, focusing on DOM manipulation beyond simple tree navigation. We'll explore efficient search methods and techniques for modifying HTML structure. One common DOM search method is ex
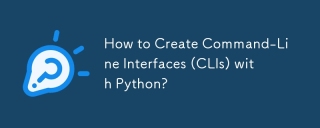
This article guides Python developers on building command-line interfaces (CLIs). It details using libraries like typer, click, and argparse, emphasizing input/output handling, and promoting user-friendly design patterns for improved CLI usability.


Hot AI Tools

Undresser.AI Undress
AI-powered app for creating realistic nude photos

AI Clothes Remover
Online AI tool for removing clothes from photos.

Undress AI Tool
Undress images for free

Clothoff.io
AI clothes remover

AI Hentai Generator
Generate AI Hentai for free.

Hot Article

Hot Tools
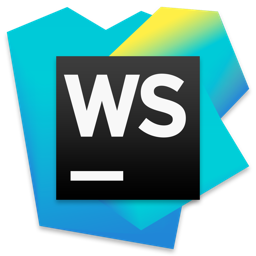
WebStorm Mac version
Useful JavaScript development tools

DVWA
Damn Vulnerable Web App (DVWA) is a PHP/MySQL web application that is very vulnerable. Its main goals are to be an aid for security professionals to test their skills and tools in a legal environment, to help web developers better understand the process of securing web applications, and to help teachers/students teach/learn in a classroom environment Web application security. The goal of DVWA is to practice some of the most common web vulnerabilities through a simple and straightforward interface, with varying degrees of difficulty. Please note that this software

Zend Studio 13.0.1
Powerful PHP integrated development environment
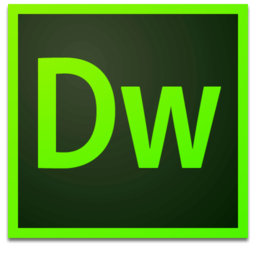
Dreamweaver Mac version
Visual web development tools

Notepad++7.3.1
Easy-to-use and free code editor
