


How to Implement a Bidirectional Hash Table in Python for Multiple Keys with the Same Value?
Bidirectional Hash Table Implementation in Python
Python's built-in dict is a highly useful data structure, providing efficient lookup based on keys. However, situations arise when reverse lookup from values is desired. This article explores the most efficient methods of implementing a bidirectional hash table, offering an example inspired by existing solutions and modified to address specific requirements.
Implementation
The presented solution employs a custom class bidict that extends the standard Python's dict. It maintains an additional inverse dictionary that automatically updates itself whenever the bidict is modified. This inverse dictionary maps values to a list of keys that have that value assigned to them.
Importantly, unlike existing libraries, this implementation allows multiple keys with the same value. This feature is crucial in certain scenarios.
Usage
The bidict class employs the following key methods:
- bidict(args, kwargs): Constructor, accepting arguments and keyword arguments that are stored in the standard dict and inverse dictionaries.
- bidict.__setitem__(key, value): Sets the key to the value. If key is already in the bidict, it removes key from the list of keys associated with its current value in the inverse dictionary. It then sets the key to the value in both dictionaries, updating the list of keys associated with the value in the inverse dictionary.
- bidict.__delitem__(key): Removes the key from the bidict. It finds the associated value of key and updates the inverse dictionary accordingly, removing key from the list of keys associated with value. If the list of keys for value becomes empty, value is removed from the inverse dictionary.
Example Usage
<code class="python">bd = bidict({'a': 1, 'b': 2}) print(bd.inverse) # {1: ['a'], 2: ['b']} bd['c'] = 1 print(bd.inverse) # {1: ['a', 'c'], 2: ['b']} del bd['c'] print(bd.inverse) # {1: ['a'], 2: ['b']}</code>
The above is the detailed content of How to Implement a Bidirectional Hash Table in Python for Multiple Keys with the Same Value?. For more information, please follow other related articles on the PHP Chinese website!
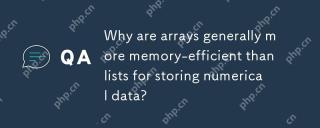
Arraysaregenerallymorememory-efficientthanlistsforstoringnumericaldataduetotheirfixed-sizenatureanddirectmemoryaccess.1)Arraysstoreelementsinacontiguousblock,reducingoverheadfrompointersormetadata.2)Lists,oftenimplementedasdynamicarraysorlinkedstruct
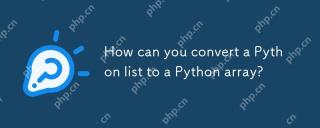
ToconvertaPythonlisttoanarray,usethearraymodule:1)Importthearraymodule,2)Createalist,3)Usearray(typecode,list)toconvertit,specifyingthetypecodelike'i'forintegers.Thisconversionoptimizesmemoryusageforhomogeneousdata,enhancingperformanceinnumericalcomp
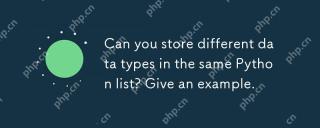
Python lists can store different types of data. The example list contains integers, strings, floating point numbers, booleans, nested lists, and dictionaries. List flexibility is valuable in data processing and prototyping, but it needs to be used with caution to ensure the readability and maintainability of the code.
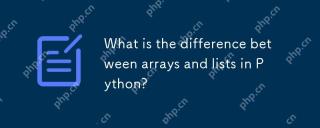
Pythondoesnothavebuilt-inarrays;usethearraymoduleformemory-efficienthomogeneousdatastorage,whilelistsareversatileformixeddatatypes.Arraysareefficientforlargedatasetsofthesametype,whereaslistsofferflexibilityandareeasiertouseformixedorsmallerdatasets.
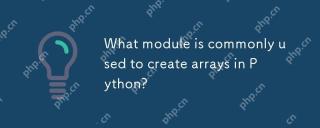
ThemostcommonlyusedmoduleforcreatingarraysinPythonisnumpy.1)Numpyprovidesefficienttoolsforarrayoperations,idealfornumericaldata.2)Arrayscanbecreatedusingnp.array()for1Dand2Dstructures.3)Numpyexcelsinelement-wiseoperationsandcomplexcalculationslikemea
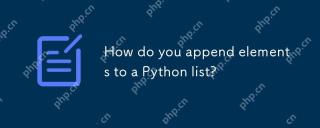
ToappendelementstoaPythonlist,usetheappend()methodforsingleelements,extend()formultipleelements,andinsert()forspecificpositions.1)Useappend()foraddingoneelementattheend.2)Useextend()toaddmultipleelementsefficiently.3)Useinsert()toaddanelementataspeci
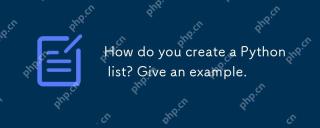
TocreateaPythonlist,usesquarebrackets[]andseparateitemswithcommas.1)Listsaredynamicandcanholdmixeddatatypes.2)Useappend(),remove(),andslicingformanipulation.3)Listcomprehensionsareefficientforcreatinglists.4)Becautiouswithlistreferences;usecopy()orsl
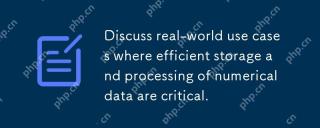
In the fields of finance, scientific research, medical care and AI, it is crucial to efficiently store and process numerical data. 1) In finance, using memory mapped files and NumPy libraries can significantly improve data processing speed. 2) In the field of scientific research, HDF5 files are optimized for data storage and retrieval. 3) In medical care, database optimization technologies such as indexing and partitioning improve data query performance. 4) In AI, data sharding and distributed training accelerate model training. System performance and scalability can be significantly improved by choosing the right tools and technologies and weighing trade-offs between storage and processing speeds.


Hot AI Tools

Undresser.AI Undress
AI-powered app for creating realistic nude photos

AI Clothes Remover
Online AI tool for removing clothes from photos.

Undress AI Tool
Undress images for free

Clothoff.io
AI clothes remover

Video Face Swap
Swap faces in any video effortlessly with our completely free AI face swap tool!

Hot Article

Hot Tools
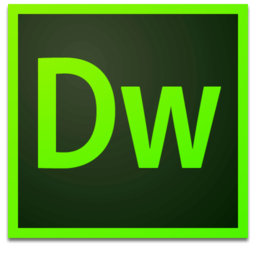
Dreamweaver Mac version
Visual web development tools

PhpStorm Mac version
The latest (2018.2.1) professional PHP integrated development tool
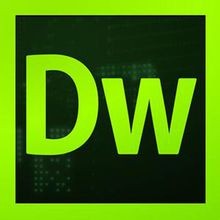
Dreamweaver CS6
Visual web development tools

Atom editor mac version download
The most popular open source editor

Safe Exam Browser
Safe Exam Browser is a secure browser environment for taking online exams securely. This software turns any computer into a secure workstation. It controls access to any utility and prevents students from using unauthorized resources.
