


Understanding Logits: A Guide to Softmax and Softmax Cross-Entropy with Logits
In the realm of machine learning, the term "logits" plays a crucial role in understanding neural network architectures and loss functions. This article delves into the concept of logits and explores the key differences between two fundamental TensorFlow functions: tf.nn.softmax and tf.nn.softmax_cross_entropy_with_logits.
What are Logits?
Logits refer to the unscaled, linear outputs of a neural network layer. Unlike probabilities, which range from 0 to 1, logits can take on any real value. The use of logits is prevalent in softmax functions, which normalize these values into probabilities.
Softmax: Converting Logits into Probabilities
The tf.nn.softmax function transforms logits into probabilities. It operates on unscaled outputs, squishing them into a range from 0 to 1 such that the sum of all probabilities equals 1. This function is extensively used in deep neural networks (DNNs) to compute the probabilities of different classes.
Softmax Cross-Entropy with Logits: A Combined Approach
tf.nn.softmax_cross_entropy_with_logits combines the softmax function with the calculation of cross-entropy loss. It performs the following steps in one mathematically efficient operation:
- Converts logits to probabilities using softmax.
- Computes the cross-entropy loss between the predicted probabilities and the ground truth labels.
Cross-Entropy Loss: Measuring Model Performance
Cross-entropy loss quantifies the divergence between the model's predicted probabilities and the true class labels. It provides a metric for evaluating model performance and is commonly used in classification tasks.
Choosing the Right Function
When optimizing a model with cross-entropy loss and softmax activation, it is generally recommended to use tf.nn.softmax_cross_entropy_with_logits. This function addresses numerical instability issues and is more mathematically efficient than performing softmax and cross-entropy calculation separately.
In situations where the labels belong to a single class (i.e., one-hot encoding), consider using tf.nn.sparse_softmax_cross_entropy_with_logits. This function optimizes memory usage and efficiency in such scenarios.
The above is the detailed content of **Softmax and Logits: When to Use `tf.nn.softmax_cross_entropy_with_logits` vs. Separate Softmax and Cross-Entropy Calculations?**. For more information, please follow other related articles on the PHP Chinese website!
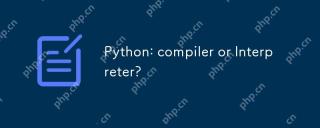
Python is an interpreted language, but it also includes the compilation process. 1) Python code is first compiled into bytecode. 2) Bytecode is interpreted and executed by Python virtual machine. 3) This hybrid mechanism makes Python both flexible and efficient, but not as fast as a fully compiled language.
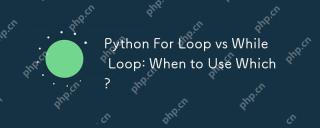
Useaforloopwheniteratingoverasequenceorforaspecificnumberoftimes;useawhileloopwhencontinuinguntilaconditionismet.Forloopsareidealforknownsequences,whilewhileloopssuitsituationswithundeterminediterations.
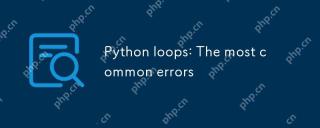
Pythonloopscanleadtoerrorslikeinfiniteloops,modifyinglistsduringiteration,off-by-oneerrors,zero-indexingissues,andnestedloopinefficiencies.Toavoidthese:1)Use'i
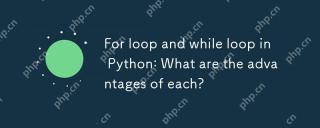
Forloopsareadvantageousforknowniterationsandsequences,offeringsimplicityandreadability;whileloopsareidealfordynamicconditionsandunknowniterations,providingcontrolovertermination.1)Forloopsareperfectforiteratingoverlists,tuples,orstrings,directlyacces
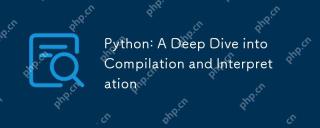
Pythonusesahybridmodelofcompilationandinterpretation:1)ThePythoninterpretercompilessourcecodeintoplatform-independentbytecode.2)ThePythonVirtualMachine(PVM)thenexecutesthisbytecode,balancingeaseofusewithperformance.
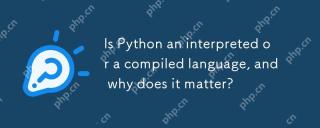
Pythonisbothinterpretedandcompiled.1)It'scompiledtobytecodeforportabilityacrossplatforms.2)Thebytecodeistheninterpreted,allowingfordynamictypingandrapiddevelopment,thoughitmaybeslowerthanfullycompiledlanguages.
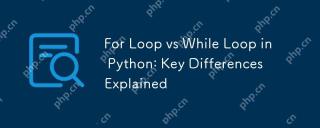
Forloopsareidealwhenyouknowthenumberofiterationsinadvance,whilewhileloopsarebetterforsituationswhereyouneedtoloopuntilaconditionismet.Forloopsaremoreefficientandreadable,suitableforiteratingoversequences,whereaswhileloopsoffermorecontrolandareusefulf
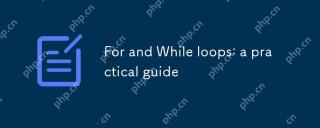
Forloopsareusedwhenthenumberofiterationsisknowninadvance,whilewhileloopsareusedwhentheiterationsdependonacondition.1)Forloopsareidealforiteratingoversequenceslikelistsorarrays.2)Whileloopsaresuitableforscenarioswheretheloopcontinuesuntilaspecificcond


Hot AI Tools

Undresser.AI Undress
AI-powered app for creating realistic nude photos

AI Clothes Remover
Online AI tool for removing clothes from photos.

Undress AI Tool
Undress images for free

Clothoff.io
AI clothes remover

Video Face Swap
Swap faces in any video effortlessly with our completely free AI face swap tool!

Hot Article

Hot Tools

MinGW - Minimalist GNU for Windows
This project is in the process of being migrated to osdn.net/projects/mingw, you can continue to follow us there. MinGW: A native Windows port of the GNU Compiler Collection (GCC), freely distributable import libraries and header files for building native Windows applications; includes extensions to the MSVC runtime to support C99 functionality. All MinGW software can run on 64-bit Windows platforms.
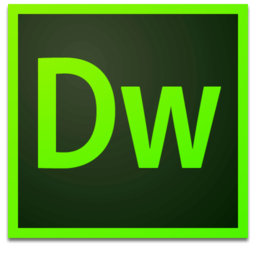
Dreamweaver Mac version
Visual web development tools

MantisBT
Mantis is an easy-to-deploy web-based defect tracking tool designed to aid in product defect tracking. It requires PHP, MySQL and a web server. Check out our demo and hosting services.
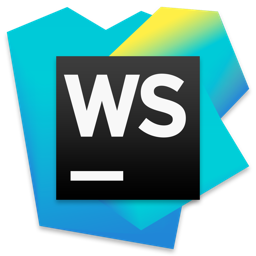
WebStorm Mac version
Useful JavaScript development tools

Zend Studio 13.0.1
Powerful PHP integrated development environment
