


Case Study on Python for AI: Unlocking Business Success through Advanced Analytics
The Power of Python in AI
Python has become the cornerstone of Artificial Intelligence (AI) development, primarily due to its simplicity, readability, and extensive library ecosystem. From machine learning to natural language processing (NLP) and deep learning, Python enables businesses to unlock new possibilities by applying AI to solve real-world challenges. This case study delves into how Python is transforming industries through AI applications, offering practical insights, tools, and approaches for businesses across sectors such as finance, healthcare, logistics, and retail.
Why Python is Ideal for AI Development
Python's dominance in AI is no accident. Several factors make it the preferred choice:
- Ease of Use: Python's syntax is intuitive and allows developers to focus on problem-solving without being bogged down by complex code.
- Extensive Libraries: Python's rich set of libraries accelerates AI development. Libraries such as Scikit-learn, TensorFlow, and PyTorch enable rapid prototyping, while Pandas and NumPy handle data manipulation.
- Active Community: Python has a large, active community that continuously supports new developments, offering solutions and improvements that fuel innovation in AI.
Automated Machine Learning (AutoML) in Python
AutoML automates the end-to-end process of applying machine learning to real-world problems. With Python’s AutoML libraries, even non-experts can build and deploy high-performing models.
Use Case:
Fraud Detection in the Financial Sector
- Business Challenge: A financial institution needed to detect fraudulent transactions faster and more accurately.
- Solution: Using Python's H2O.ai AutoML framework, the institution automated the model-building process, reducing development time and improving accuracy by 30%.
- Result: The automated solution enabled the business to detect fraud in real-time, saving millions of dollars in potential losses.
Key Libraries Used:
- H2O.ai, TPOT, Auto-sklearn
Why It Matters:
AutoML eliminates the need for manual hyperparameter tuning and model selection, allowing businesses to focus on deploying AI solutions that deliver immediate impact.
Time Series Forecasting with Python
Accurate time series forecasting is crucial for sectors like retail, finance, and manufacturing, where predicting future trends can drive better decision-making.
Use Case: Sales Forecasting for Retail
- Business Challenge: A global retail company struggled to predict sales trends accurately, leading to inventory mismanagement.
- Solution: The company implemented Python’s Prophet library for time series forecasting, improving sales forecast accuracy by 20%.
- Result: With more precise demand forecasting, the company reduced overstock and stockouts, saving significant operational costs.
Key Libraries Used:
- Prophet, statsmodels
Why It Matters:
Python’s time series libraries provide robust tools for businesses to forecast trends, optimize supply chains, and make better financial decisions.
Natural Language Processing (NLP) for Sentiment Analysis
NLP helps businesses analyze vast amounts of unstructured data, such as customer feedback, reviews, and social media posts, to extract actionable insights.
Use Case: Customer Sentiment Analysis in E-commerce
- Business Challenge: An e-commerce company wanted to better understand customer sentiment to enhance product recommendations and customer support.
- Solution: Using Python's spaCy and NLTK libraries, the company performed sentiment analysis on customer reviews and social media posts.
- Result: The insights from sentiment analysis helped improve customer satisfaction and retention by 15%, as the company addressed recurring issues proactively.
Key Libraries Used:
- spaCy, NLTK, transformers
Why It Matters:
Python’s NLP tools allow companies to gain real-time insights from customer interactions, enabling personalized experiences and better business strategies.
Predictive Modeling for Business Optimization
Predictive modeling is vital for industries looking to forecast future trends, improve decision-making, and optimize business operations.
Use Case: Demand Forecasting for Inventory Management
- Business Challenge: A major retailer needed a more accurate method to predict product demand and optimize inventory levels.
- Solution: By leveraging scikit-learn and XGBoost, the retailer built a predictive model that improved demand forecasting accuracy by 25%.
- Result: With more accurate forecasts, the retailer reduced inventory costs by 15%, leading to a more efficient supply chain.
Key Libraries Used:
- scikit-learn, XGBoost, Pandas
Why It Matters:
Python’s powerful libraries for predictive modeling help businesses reduce waste, optimize resources, and improve profitability.
Reinforcement Learning (RL) with Python
Reinforcement learning (RL) is an emerging field of AI that enables systems to learn by interacting with their environment. RL is used in gaming, robotics, and optimization problems.
Use Case: Optimizing Delivery Routes in Logistics
- Business Challenge: A logistics company needed to reduce delivery times while minimizing fuel consumption.
- Solution: Using Python’s OpenAI Gym and Stable-baselines3, the company implemented reinforcement learning to optimize delivery routes.
- Result: The company saw a 12% reduction in delivery times and a 10% decrease in fuel costs.
Key Libraries Used:
- OpenAI Gym, Stable-baselines3
Why It Matters:
Reinforcement learning enables businesses to optimize complex operations in real-time, driving efficiency and reducing costs.
Ethics and Bias in AI: Ensuring Fairness with Python
AI systems, if not carefully managed, can perpetuate biases present in the training data. Python offers tools to detect and mitigate bias, ensuring that AI models are fair and transparent.
Use Case: Bias Mitigation in Hiring Algorithms
- Business Challenge: A recruitment firm noticed that its AI-based hiring system favored certain demographics, leading to a lack of diversity.
- Solution: By using Python’s Fairlearn library, the firm detected and reduced bias in its hiring algorithms, ensuring that candidates were evaluated more fairly.
- Result: The firm achieved greater diversity in its hires, while maintaining high standards of candidate evaluation.
Key Libraries Used:
- Fairlearn, AIF360
Why It Matters:
Addressing bias in AI is critical to ensuring that models are not only accurate but also equitable, fostering trust in AI systems.
Challenges in AI Implementation with Python
While Python is widely used in AI, it comes with some challenges, particularly in scaling AI models for large datasets and real-time applications.
- Scalability: Python can be slower than other languages, particularly when handling large-scale AI applications.
- Solution: Python users can leverage Numba for just-in-time compilation or use cloud-based solutions like AWS Lambda to deploy scalable AI systems.
The Future of Python in AI
Python’s versatility and ease of use make it an essential tool for AI development. From automating machine learning with AutoML to advanced techniques like reinforcement learning, Python empowers businesses to innovate and stay competitive. As the demand for ethical, scalable AI solutions grows, Python’s ecosystem will continue to expand, making it an indispensable asset for AI-driven enterprises.
The above is the detailed content of Case Study on Python for AI: Unlocking Business Success through Advanced Analytics. For more information, please follow other related articles on the PHP Chinese website!
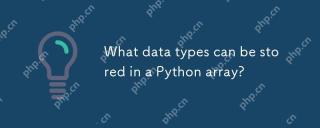
Pythonlistscanstoreanydatatype,arraymodulearraysstoreonetype,andNumPyarraysarefornumericalcomputations.1)Listsareversatilebutlessmemory-efficient.2)Arraymodulearraysarememory-efficientforhomogeneousdata.3)NumPyarraysareoptimizedforperformanceinscient
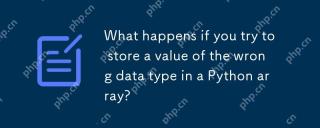
WhenyouattempttostoreavalueofthewrongdatatypeinaPythonarray,you'llencounteraTypeError.Thisisduetothearraymodule'sstricttypeenforcement,whichrequiresallelementstobeofthesametypeasspecifiedbythetypecode.Forperformancereasons,arraysaremoreefficientthanl
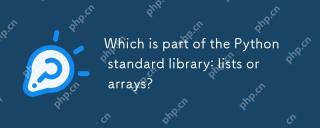
Pythonlistsarepartofthestandardlibrary,whilearraysarenot.Listsarebuilt-in,versatile,andusedforstoringcollections,whereasarraysareprovidedbythearraymoduleandlesscommonlyusedduetolimitedfunctionality.
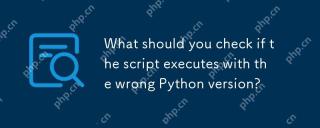
ThescriptisrunningwiththewrongPythonversionduetoincorrectdefaultinterpretersettings.Tofixthis:1)CheckthedefaultPythonversionusingpython--versionorpython3--version.2)Usevirtualenvironmentsbycreatingonewithpython3.9-mvenvmyenv,activatingit,andverifying
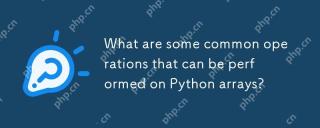
Pythonarrayssupportvariousoperations:1)Slicingextractssubsets,2)Appending/Extendingaddselements,3)Insertingplaceselementsatspecificpositions,4)Removingdeleteselements,5)Sorting/Reversingchangesorder,and6)Listcomprehensionscreatenewlistsbasedonexistin
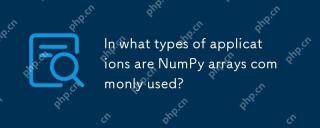
NumPyarraysareessentialforapplicationsrequiringefficientnumericalcomputationsanddatamanipulation.Theyarecrucialindatascience,machinelearning,physics,engineering,andfinanceduetotheirabilitytohandlelarge-scaledataefficiently.Forexample,infinancialanaly
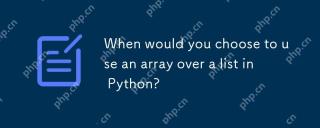
Useanarray.arrayoveralistinPythonwhendealingwithhomogeneousdata,performance-criticalcode,orinterfacingwithCcode.1)HomogeneousData:Arrayssavememorywithtypedelements.2)Performance-CriticalCode:Arraysofferbetterperformancefornumericaloperations.3)Interf
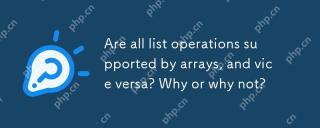
No,notalllistoperationsaresupportedbyarrays,andviceversa.1)Arraysdonotsupportdynamicoperationslikeappendorinsertwithoutresizing,whichimpactsperformance.2)Listsdonotguaranteeconstanttimecomplexityfordirectaccesslikearraysdo.


Hot AI Tools

Undresser.AI Undress
AI-powered app for creating realistic nude photos

AI Clothes Remover
Online AI tool for removing clothes from photos.

Undress AI Tool
Undress images for free

Clothoff.io
AI clothes remover

Video Face Swap
Swap faces in any video effortlessly with our completely free AI face swap tool!

Hot Article

Hot Tools

PhpStorm Mac version
The latest (2018.2.1) professional PHP integrated development tool

mPDF
mPDF is a PHP library that can generate PDF files from UTF-8 encoded HTML. The original author, Ian Back, wrote mPDF to output PDF files "on the fly" from his website and handle different languages. It is slower than original scripts like HTML2FPDF and produces larger files when using Unicode fonts, but supports CSS styles etc. and has a lot of enhancements. Supports almost all languages, including RTL (Arabic and Hebrew) and CJK (Chinese, Japanese and Korean). Supports nested block-level elements (such as P, DIV),

MinGW - Minimalist GNU for Windows
This project is in the process of being migrated to osdn.net/projects/mingw, you can continue to follow us there. MinGW: A native Windows port of the GNU Compiler Collection (GCC), freely distributable import libraries and header files for building native Windows applications; includes extensions to the MSVC runtime to support C99 functionality. All MinGW software can run on 64-bit Windows platforms.

MantisBT
Mantis is an easy-to-deploy web-based defect tracking tool designed to aid in product defect tracking. It requires PHP, MySQL and a web server. Check out our demo and hosting services.
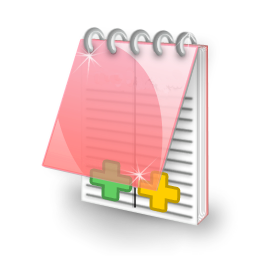
EditPlus Chinese cracked version
Small size, syntax highlighting, does not support code prompt function
