


Weighted Random Selection: Replacement vs. Non-Replacement
Weighted random selection is a fundamental technique used in various applications. It involves sampling elements from a given list with probability distributions determined by specified weights. When selecting elements with replacement, each item can be chosen multiple times, leading to a higher likelihood of picking items with higher weights. In contrast, selection without replacement restricts the selection of an item once it has been chosen.
Finding efficient algorithms for weighted random selection, especially with replacement, can be challenging. Existing methods, including modified reservoir algorithms, prove unsuitable for significant fraction selections from small list sizes.
An Efficient Approach: Alias Method
One approach that excels in this scenario is the alias method. This technique creates a structured set of bins, each representing a portion of the weighted list. By utilizing bit operations, the bins can be efficiently indexed, avoiding binary searches. Each bin contains two elements from the original list, enabling efficient representation of the distribution.
For instance, consider a list of five equally weighted choices: (a:1, b:1, c:1, d:1, e:1). The alias method creates a set of eight bins, each with a probability mass of 0.125.
- Normalization: Adjust the weights to sum to 1.0. In this case, (a:0.2 b:0.2 c:0.2 d:0.2 e:0.2).
- Partition: Allocate bins with weights lower than the partition probability (0.125), starting with the lowest weight. Here, (p1{a|null,1.0},p2,p3,p4,p5,p6,p7,p8).
- Filling: Fill remaining space in the partition with the highest weight variable. For example, (p1{a|null,1.0},p2{a|b,0.6},p3,p4,p5,p6,p7,p8).
Runtime Selection:
At runtime, we generate a random number and use bit operations to efficiently determine the bin corresponding to the probability distribution. If the bin is split, we use the decimal portion of the random number to select between the two elements in the bin.
In summary, the alias method provides an efficient technique for weighted random selection with replacement. It utilizes bit operations for fast bin indexing and achieves accurate probability distributions by carefully dividing the weights into manageable bins.
The above is the detailed content of When to Use Replacement vs. Non-Replacement in Weighted Random Selection?. For more information, please follow other related articles on the PHP Chinese website!
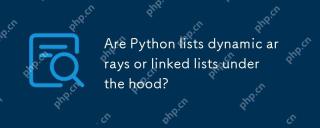
Pythonlistsareimplementedasdynamicarrays,notlinkedlists.1)Theyarestoredincontiguousmemoryblocks,whichmayrequirereallocationwhenappendingitems,impactingperformance.2)Linkedlistswouldofferefficientinsertions/deletionsbutslowerindexedaccess,leadingPytho
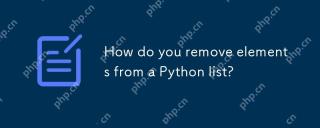
Pythonoffersfourmainmethodstoremoveelementsfromalist:1)remove(value)removesthefirstoccurrenceofavalue,2)pop(index)removesandreturnsanelementataspecifiedindex,3)delstatementremoveselementsbyindexorslice,and4)clear()removesallitemsfromthelist.Eachmetho
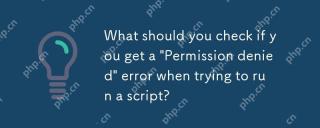
Toresolvea"Permissiondenied"errorwhenrunningascript,followthesesteps:1)Checkandadjustthescript'spermissionsusingchmod xmyscript.shtomakeitexecutable.2)Ensurethescriptislocatedinadirectorywhereyouhavewritepermissions,suchasyourhomedirectory.
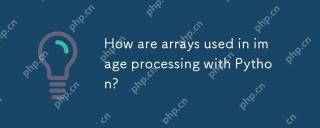
ArraysarecrucialinPythonimageprocessingastheyenableefficientmanipulationandanalysisofimagedata.1)ImagesareconvertedtoNumPyarrays,withgrayscaleimagesas2Darraysandcolorimagesas3Darrays.2)Arraysallowforvectorizedoperations,enablingfastadjustmentslikebri
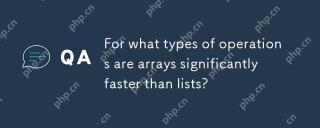
Arraysaresignificantlyfasterthanlistsforoperationsbenefitingfromdirectmemoryaccessandfixed-sizestructures.1)Accessingelements:Arraysprovideconstant-timeaccessduetocontiguousmemorystorage.2)Iteration:Arraysleveragecachelocalityforfasteriteration.3)Mem
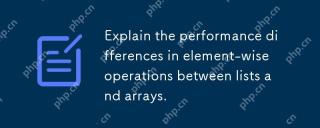
Arraysarebetterforelement-wiseoperationsduetofasteraccessandoptimizedimplementations.1)Arrayshavecontiguousmemoryfordirectaccess,enhancingperformance.2)Listsareflexiblebutslowerduetopotentialdynamicresizing.3)Forlargedatasets,arrays,especiallywithlib
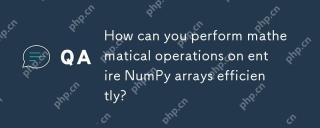
Mathematical operations of the entire array in NumPy can be efficiently implemented through vectorized operations. 1) Use simple operators such as addition (arr 2) to perform operations on arrays. 2) NumPy uses the underlying C language library, which improves the computing speed. 3) You can perform complex operations such as multiplication, division, and exponents. 4) Pay attention to broadcast operations to ensure that the array shape is compatible. 5) Using NumPy functions such as np.sum() can significantly improve performance.
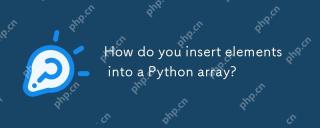
In Python, there are two main methods for inserting elements into a list: 1) Using the insert(index, value) method, you can insert elements at the specified index, but inserting at the beginning of a large list is inefficient; 2) Using the append(value) method, add elements at the end of the list, which is highly efficient. For large lists, it is recommended to use append() or consider using deque or NumPy arrays to optimize performance.


Hot AI Tools

Undresser.AI Undress
AI-powered app for creating realistic nude photos

AI Clothes Remover
Online AI tool for removing clothes from photos.

Undress AI Tool
Undress images for free

Clothoff.io
AI clothes remover

Video Face Swap
Swap faces in any video effortlessly with our completely free AI face swap tool!

Hot Article

Hot Tools
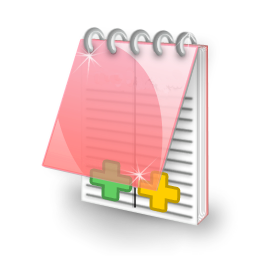
EditPlus Chinese cracked version
Small size, syntax highlighting, does not support code prompt function

SublimeText3 Linux new version
SublimeText3 Linux latest version
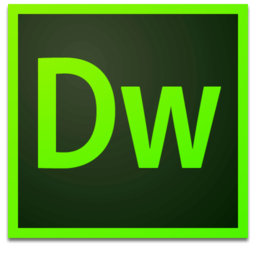
Dreamweaver Mac version
Visual web development tools

SublimeText3 English version
Recommended: Win version, supports code prompts!

MinGW - Minimalist GNU for Windows
This project is in the process of being migrated to osdn.net/projects/mingw, you can continue to follow us there. MinGW: A native Windows port of the GNU Compiler Collection (GCC), freely distributable import libraries and header files for building native Windows applications; includes extensions to the MSVC runtime to support C99 functionality. All MinGW software can run on 64-bit Windows platforms.
