When the hype has created "tremendous traffic", no one cares about whether the product is great or not.
Recently, OpenAI’s secret project “Q*” has been receiving widespread attention from insiders. Last month, a project based on it and codenamed "Strawberry" was exposed again. Presumably, the project is capable of providing advanced reasoning capabilities. In recent days, regarding this project, there have been several waves of rumors on the Internet that "the dead pigeon will not pay for the life of the human being". Especially the account of "Brother Strawberry", which promotes non-stop, gives people expectations but also disappoints them. Unexpectedly, wherever this Sam Altman appears, the "marketing account" where he posts is actually an intelligent body under his skin? Today, the founder of an AI agent startup company "MultiOn" came out directly to claim: Although we did not wait for OpenAI to release "Q*", we released a new agent Agent that controls the "Strawberry Brother" account Q, come and play with us online!联 Multion co -founder and CEO DIV GARG, who took a break from a PhD in computer science in Stanford. It seems that OpenAI’s marketing operation of making wedding dresses for itself has left everyone confused. After all, many people have been staying up all night waiting for OpenAI’s “big news” recently. This goes back to the interaction between Sam Altman and "Brother Strawberry". Under the photo of strawberries posted by Sam Altman, he replied to "Brother Strawberry": The surprise will come soon.
However, Div Garg, the founder of “MultiOn”, has quietly deleted the post claiming that Agent Q is “Brother Strawberry”.
This time, "MultiOn" announced that the Agent Q they released is a breakthrough AI agent
. Its training method combines Monte Carlo Tree Search (MCTS) and self-criticism, and it learns from human feedback through an algorithm called Direct Preference Optimization (DPO). At the same time, as a next-generation AI agent with planning and AI self-healing capabilities, Agent Q’s performance is 3.4 times higher than the LLama 3 baseline zero-sample performance. At the same time, in the evaluation of real-scenario tasks, Agent Q's success rate reached 95.4%.
What can Agent Q do? Let’s take a look at the official demo first.
It can reserve a seat for you at a certain restaurant at a certain time.
Then perform web operations for you, such as checking the availability. Finally booked successfully.
In addition, you can book flights (such as flying from New York to San Francisco this Saturday, one-way, window seat and economy class).
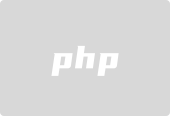
However, netizens don’t seem to buy Agent Q. What everyone is more concerned about is whether they are really using the "Strawberry Brother" account to promote things. Some people even call them shameless liars.
目前,Agent Q 的相關論文已經放出,由 MultiOn 和史丹佛大學的研究者共同撰寫。這項研究的成果將在今年稍後向開發人員和使用 MultiOn 的一般用戶開放。
- 論文地址:https://multion-research.s3.us-east-2.amazonaws.com/AgentQ.pdf
網頁上實施規劃並自我糾錯,從成功和失敗的經驗中學習,提高它在複雜任務中的表現。最終,該智能體可以更好地規劃如何在網路上衝浪,以適應現實世界的複雜情況。 在技術細節上, Agent Q 的主要組件包括如下:使用MCTS(Monte Carlo Tree 探索,蒙特卡洛樹搜尋)進行引導式搜尋:該技術透過不同的操作和網頁來自主生成數據,以平衡探索和利用。 MCTS 使用高採樣溫度和多樣化提示來擴展操作空間,確保多樣化和最佳的軌跡集合。 AI 自我批評:在每個步驟中,基於 AI 的自我批評都會提供有價值的回饋,從而完善智能體的決策過程。這一步驟級回饋對於長期任務至關重要,因為稀疏訊號通常會導致學習困難。 直接偏好最佳化(DPO):此演算法透過從 MCTS 產生的資料建立偏好對以微調模型。這種離策略訓練方法允許模型從聚合資料集(包括搜尋過程中探索的次優分支)中有效地學習,從而提高複雜環境中的成功率。 下面重點講一下網頁(Web-Page)端的 MCTS 演算法。研究者探索如何透過 MCTS 賦予智能體額外的搜尋能力。 在以往的工作中,MCTS 演算法通常由四個階段組成:選擇、擴展、模擬和反向傳播,每個階段在平衡探索與利用、迭代細化策略方面都發揮關鍵作用。 研究者將網頁智能體執行公式化為網頁樹搜索,其中狀態由智能體歷史和當前網頁的 DOM 樹組成。與國際象棋或圍棋等棋盤遊戲不同,研究者使用的複雜網路智能體操作空間是開放格式且可變的。 研究者將基礎模型用作操作建議(action-proposal)分佈,並在每個節點(網頁)上採樣固定數量的可能操作。一旦在瀏覽器中選擇並執行一個操作,則會遍歷下個網頁,並且該網頁與更新的歷史記錄共同成為新節點。 研究者對回饋模型進行多次迭代查詢,每次從清單中刪除從上一次迭代中選擇的最佳操作,直到對所有操作進行完整排序。下圖 4 為完整的 AI 回饋過程。 擴展和回溯。研究者在瀏覽器環境中選擇並執行一個操作以到達一個新節點(頁面)。從選定的狀態節點軌跡開始,他們使用目前策略 ?_? 展開軌跡,直到到達終止狀態。環境在軌跡結束時返回獎勵 ?,其中如果智能體成功則 ? = 1,否則 ? = 0。接下來,透過從葉節點到根節點自下而上地更新每個節點的值來反向傳播此獎勵,如下所示:下圖 3 展示了所有結果和基線。當讓智能體在測試時能夠搜尋資訊時,即為基礎xLAM-v0.1-r 模型應用MCTS 時,成功率從28.6% 提升到了48.4%,接近平均人類表現的50.0%,並且顯著超過了僅透過結果監督訓練的零樣本DPO 模型的表現。 研究者進一步根據下圖中概述的演算法對基礎模型進行了微調,結果比基礎 DPO 模型提高了 0.9%。在精心訓練的 Agent Q 模型上再應用 MCTS,智能體的表現提升到了 50.5%,略微超過了人類的平均表現。他們認為,即使智能體經過了大量的強化學習訓練,在測試時具備搜尋能力仍然是一個重要的範式轉移。與沒有經過訓練的零樣本智能體相比,這是一個顯著的進步。 此外,儘管密集級監督比純粹的基於結果的監督有所改善,但在 WebShop 環境中,這種訓練方法的提升效果並不大。這是因為在這個環境裡,智能體只需要做很短的決策路徑,可以透過結果來學習信用分配。 研究者選擇了讓智能體在 OpenTable 官網上預訂餐廳的任務來測試 Agent Q 框架在真實世界中的表現如何。要完成這個訂餐任務,智能體必須在 OpenTable 網站上找到餐廳的頁面,選擇特定的日期和時間,並挑選符合使用者偏好的座位,最後提交使用者的聯絡方式,才能預定成功。 最初,他們對 xLAM-v0.1-r 模型進行了實驗,但該模型表現不佳,初始成功率僅為 0.0%。因此,他們轉而使用 LLaMa 70B Instruct 模型,取得了一些初步的成功。 不過由於 OpenTable 是一個即時環境,很難透過程式設計或自動化的方式進行測量和評估。因此,研究者使用GPT-4-V 根據以下指標為每個軌跡收集獎勵:(1) 日期和時間設定正確,(2) 聚會規模設定正確,(3) 使用者資訊輸入正確,以及(4) 點擊完成預訂。如果滿足上述所有條件,則視為智能體完成了任務。結果監督設定如下圖 5 所示。 而 Agent Q 將 LLaMa-3 模型的零樣本成功率從 18.6% 大幅提高到了 81.7%,這個結果僅在單日自主資料收集後便實現了,相當於成功率激增了 340%。在引入線上搜尋功能後,成功率更是攀升至 95.4%。 參考連結:https://www.multion.ai/blog/introducing-agent-q-research-breakthrough-for-the-next-generation-of-ai-agents-with-planning- and-self-healing-capabilitiesThe above is the detailed content of The account that has been breaking the news about OpenAI's 'Strawberry” is actually an intelligent agent? Stanford startup 'hype” AgentQ. For more information, please follow other related articles on the PHP Chinese website!
Statement:The content of this article is voluntarily contributed by netizens, and the copyright belongs to the original author. This site does not assume corresponding legal responsibility. If you find any content suspected of plagiarism or infringement, please contact admin@php.cn