金融、科研、医疗和AI等领域中,高效存储和处理数值数据至关重要。 1) 在金融中,使用内存映射文件和NumPy库可显着提升数据处理速度。 2) 科研领域,HDF5文件优化数据存储和检索。 3) 医疗中,数据库优化技术如索引和分区提高数据查询性能。 4) AI中,数据分片和分布式训练加速模型训练。通过选择适当的工具和技术,并权衡存储与处理速度之间的trade-off,可以显着提升系统性能和可扩展性。
When it comes to the efficient storage and processing of numerical data, real-world applications abound where these aspects are not just beneficial but absolutely critical. Let's dive into some of these scenarios, exploring why they matter and how they can be optimized.
In the world of finance, every millisecond counts. High-frequency trading platforms rely heavily on the ability to process vast amounts of numerical data in real-time. The difference between a profit and a loss can hinge on how quickly a system can analyze market data, execute trades, and adjust strategies. Here, efficient data structures like arrays or specialized libraries like NumPy in Python can be game-changers. I've worked on projects where we shaved off critical milliseconds by using memory-mapped files to store time-series data, allowing for lightning-fast access and manipulation.
import numpy as np import mmap # Example of using memory-mapped files for efficient data handling with open('data.bin', 'r b') as f: mm = mmap.mmap(f.fileno(), 0) data = np.frombuffer(mm, dtype=np.float64) # Process data here mm.close()
Scientific research, particularly in fields like climate modeling or particle physics, also demands robust numerical data handling. These applications often deal with terabytes of data, and the ability to store and process this efficiently can significantly impact the speed of discovery. For instance, in climate modeling, we need to store and analyze large datasets of temperature, humidity, and other variables over time. Using HDF5 files, which are designed for handling large datasets, can be a lifesaver. I once optimized a climate model's data pipeline by switching to HDF5, which not only reduced storage requirements but also sped up data retrieval by orders of magnitude.
import h5py # Example of using HDF5 for efficient storage and retrieval with h5py.File('climate_data.h5', 'w') as hdf: dataset = hdf.create_dataset('temperature', data=np.random.rand(1000, 1000)) # Store other datasets similarly # Later, to read the data with h5py.File('climate_data.h5', 'r') as hdf: temperature_data = hdf['temperature'][:] # Process the data
In healthcare, efficient data handling can literally save lives. Consider electronic health records (EHRs) systems, where patient data needs to be stored securely and accessed quickly. Here, database optimization techniques like indexing and partitioning become crucial. I've seen systems where we implemented columnar storage for numerical data like blood pressure readings, which drastically improved query performance for analytical purposes.
-- Example of optimizing EHR data storage CREATE TABLE patient_data ( patient_id INT, blood_pressure FLOAT ) PARTITION BY RANGE (patient_id) ( PARTITION p0 VALUES LESS THAN (10000), PARTITION p1 VALUES LESS THAN (20000), -- More partitions as needed ); CREATE INDEX idx_blood_pressure ON patient_data(blood_pressure);
Machine learning and AI applications are another arena where numerical data efficiency is paramount. Training models on large datasets requires not only computational power but also efficient data pipelines. Techniques like data sharding, where data is split across multiple nodes, can significantly speed up training times. I've implemented systems where we used TensorFlow's distributed training capabilities to process data more efficiently, allowing for faster model iterations.
import tensorflow as tf # Example of distributed training with TensorFlow strategy = tf.distribute.MirroredStrategy() with strategy.scope(): model = tf.keras.Sequential([...]) # Define your model model.compile(optimizer='adam', loss='sparse_categorical_crossentropy', metrics=['accuracy']) # Prepare the dataset dataset = tf.data.Dataset.from_tensor_slices((features, labels)).shuffle(10000).batch(32) dist_dataset = strategy.experimental_distribute_dataset(dataset) # Train the model model.fit(dist_dataset, epochs=10)
Optimizing numerical data handling isn't without its challenges. One common pitfall is underestimating the importance of data serialization and deserialization. In high-throughput systems, the choice of serialization format (eg, JSON vs. Protocol Buffers) can have a significant impact on performance. I've encountered projects where switching from JSON to Protocol Buffers reduced data transfer times by up to 50%.
Another consideration is the trade-off between storage efficiency and processing speed. For instance, using compressed storage formats can save space but might slow down data retrieval. It's crucial to profile your application and find the right balance. I've seen cases where we had to revert from using compression because the decompression overhead was too high for real-time applications.
In conclusion, efficient storage and processing of numerical data are critical in numerous real-world applications, from finance and scientific research to healthcare and machine learning. By choosing the right tools and techniques, and being mindful of the trade-offs involved, you can significantly enhance the performance and scalability of your systems. Remember, the key is to always test and measure the impact of your optimizations – what works in one scenario might not be the best solution for another.
以上是讨论有效存储和数值数据的处理至关重要的实际用例。的详细内容。更多信息请关注PHP中文网其他相关文章!
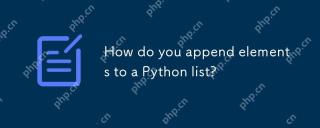
toAppendElementStoApythonList,usetheappend()方法forsingleements,Extend()formultiplelements,andinsert()forspecificpositions.1)useeAppend()foraddingoneOnelementAttheend.2)useextendTheEnd.2)useextendexendExendEnd(
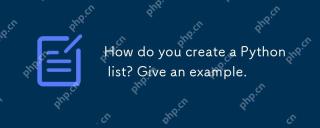
TocreateaPythonlist,usesquarebrackets[]andseparateitemswithcommas.1)Listsaredynamicandcanholdmixeddatatypes.2)Useappend(),remove(),andslicingformanipulation.3)Listcomprehensionsareefficientforcreatinglists.4)Becautiouswithlistreferences;usecopy()orsl
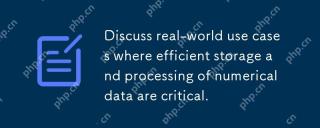
金融、科研、医疗和AI等领域中,高效存储和处理数值数据至关重要。 1)在金融中,使用内存映射文件和NumPy库可显着提升数据处理速度。 2)科研领域,HDF5文件优化数据存储和检索。 3)医疗中,数据库优化技术如索引和分区提高数据查询性能。 4)AI中,数据分片和分布式训练加速模型训练。通过选择适当的工具和技术,并权衡存储与处理速度之间的trade-off,可以显着提升系统性能和可扩展性。
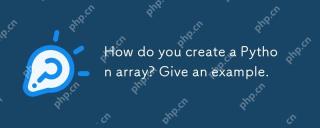
pythonarraysarecreatedusiseThearrayModule,notbuilt-Inlikelists.1)importThearrayModule.2)指定tefifythetypecode,例如,'i'forineizewithvalues.arreaysofferbettermemoremorefferbettermemoryfforhomogeNogeNogeNogeNogeNogeNogeNATATABUTESFELLESSFRESSIFERSTEMIFICETISTHANANLISTS。
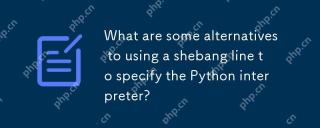
除了shebang线,还有多种方法可以指定Python解释器:1.直接使用命令行中的python命令;2.使用批处理文件或shell脚本;3.使用构建工具如Make或CMake;4.使用任务运行器如Invoke。每个方法都有其优缺点,选择适合项目需求的方法很重要。
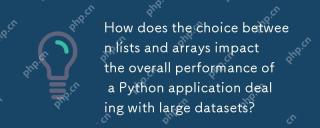
ForhandlinglargedatasetsinPython,useNumPyarraysforbetterperformance.1)NumPyarraysarememory-efficientandfasterfornumericaloperations.2)Avoidunnecessarytypeconversions.3)Leveragevectorizationforreducedtimecomplexity.4)Managememoryusagewithefficientdata
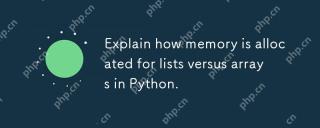
Inpython,ListSusedynamicMemoryAllocationWithOver-Asalose,而alenumpyArraySallaySallocateFixedMemory.1)listssallocatemoremoremoremorythanneededinentientary上,respizeTized.2)numpyarsallaysallaysallocateAllocateAllocateAlcocateExactMemoryForements,OfferingPrediCtableSageButlessemageButlesseflextlessibility。
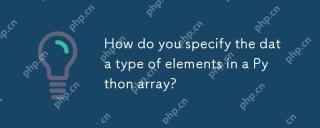
Inpython,YouCansspecthedatatAtatatPeyFelemereModeRernSpant.1)Usenpynernrump.1)Usenpynyp.dloatp.dloatp.ploatm64,formor professisconsiscontrolatatypes。


热AI工具

Undresser.AI Undress
人工智能驱动的应用程序,用于创建逼真的裸体照片

AI Clothes Remover
用于从照片中去除衣服的在线人工智能工具。

Undress AI Tool
免费脱衣服图片

Clothoff.io
AI脱衣机

Video Face Swap
使用我们完全免费的人工智能换脸工具轻松在任何视频中换脸!

热门文章

热工具

SublimeText3 Linux新版
SublimeText3 Linux最新版

安全考试浏览器
Safe Exam Browser是一个安全的浏览器环境,用于安全地进行在线考试。该软件将任何计算机变成一个安全的工作站。它控制对任何实用工具的访问,并防止学生使用未经授权的资源。
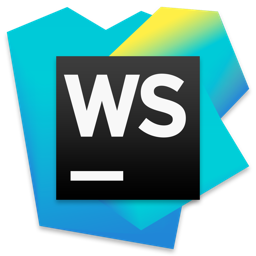
WebStorm Mac版
好用的JavaScript开发工具
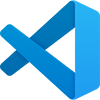
VSCode Windows 64位 下载
微软推出的免费、功能强大的一款IDE编辑器

禅工作室 13.0.1
功能强大的PHP集成开发环境