>对比特币或其价格波动了解不多,而是想做出投资决定来获利吗?该机器学习模型有您的支持。它可以比占星家更好地预测价格。在本文中,我们将使用ZenML和MLFlow构建一个用于预测和预测比特币价格的ML模型。因此,让我们开始我们的旅程,了解任何人如何使用ML和MLOPS工具来预测未来。
学习目标- 学会有效地使用API获取实时数据。
- > 了解Zenml是什么,为什么我们使用MLFLOW以及如何将其与Zenml集成。
- >探索从想法到生产的机器学习模型的部署过程。
- > >发现如何为交互式机器学习模型预测创建用户友好的简化应用程序。
- >
>本文是> > data Science Blogathon的一部分。 内容表>
问题语句- >项目实现
- 步骤1:访问API
- 步骤2:使用mongodb
- 5:数据清洁
- 步骤6:特征工程
- 步骤7:数据拆分
- 步骤8:型号培训
- 步骤9:型号评估
- 步骤10:模型部署 问题
- 问题语句
>项目实施
让我们从访问API开始。
我们为什么要这样做?您可以从不同的数据集中获取历史比特币价格数据,但是使用API,我们可以访问Live Market Data。
>步骤1:访问API
>注册API访问:
-
在以下代码的情况下,您可以从CCDATA API获取比特币价格数据,并将其转换为PANDAS DataFrame。另外,将API密钥保留在.env文件中。
步骤2:使用mongodb - 连接到数据库
MongoDB是一个NOSQL数据库,以其适应性,可扩展性和以JSON式格式存储非结构化数据的能力。
import requests import pandas as pd from dotenv import load_dotenv import os # Load the .env file load_dotenv() def fetch_crypto_data(api_uri): response = requests.get( api_uri, params={ "market": "cadli", "instrument": "BTC-USD", "limit": 5000, "aggregate": 1, "fill": "true", "apply_mapping": "true", "response_format": "JSON" }, headers={"Content-type": "application/json; charset=UTF-8"} ) if response.status_code == 200: print('API Connection Successful! \nFetching the data...') data = response.json() data_list = data.get('Data', []) df = pd.DataFrame(data_list) df['DATE'] = pd.to_datetime(df['TIMESTAMP'], unit='s') return df # Return the DataFrame else: raise Exception(f"API Error: {response.status_code} - {response.text}")
此代码连接到MongoDB,通过API检索比特币价格数据,并在最新记录日期后使用所有新条目更新数据库。
介绍Zenml⚠️如果您是Windows用户,请尝试在系统上安装WSL。 Zenml不支持Windows。
在此项目中,我们将实施使用Zenml的传统管道,并将MLFlow与Zenml集成进行实验跟踪。> >前提条件和基本zenml命令> python 3.12或更高:
您可以从这里获得:https://www.python.org/downloads/- 激活您的虚拟环境: >
import os from pymongo import MongoClient from dotenv import load_dotenv from data.management.api import fetch_crypto_data # Import the API function import pandas as pd load_dotenv() MONGO_URI = os.getenv("MONGO_URI") API_URI = os.getenv("API_URI") client = MongoClient(MONGO_URI, ssl=True, ssl_certfile=None, ssl_ca_certs=None) db = client['crypto_data'] collection = db['historical_data'] try: latest_entry = collection.find_one(sort=[("DATE", -1)]) # Find the latest date if latest_entry: last_date = pd.to_datetime(latest_entry['DATE']).strftime('%Y-%m-%d') else: last_date = '2011-03-27' # Default start date if MongoDB is empty print(f"Fetching data starting from {last_date}...") new_data_df = fetch_crypto_data(API_URI) if latest_entry: new_data_df = new_data_df[new_data_df['DATE'] > last_date] if not new_data_df.empty: data_to_insert = new_data_df.to_dict(orient='records') result = collection.insert_many(data_to_insert) print(f"Inserted {len(result.inserted_ids)} new records into MongoDB.") else: print("No new data to insert.") except Exception as e: print(f"An error occurred: {e}")
-
>所有核心Zenml命令及其功能如下:
我们正在使用MLFlow进行实验跟踪,以跟踪我们的模型,工件,指标和超参数值。我们正在注册MLFLOW以进行实验跟踪和模型部署者:
#create a virtual environment python3 -m venv venv #Activate your virtual environmnent in your project folder source venv/bin/activate
> zenml堆栈列表#Install zenml pip install zenml #To Launch zenml server and dashboard locally pip install "zenml[server]" #To check the zenml Version: zenml version #To initiate a new repository zenml init #To run the dashboard locally: zenml login --local #To know the status of our zenml Pipelines zenml show #To shutdown the zenml server zenml clean
项目结构>
步骤4:数据摄入#Integrating mlflow with ZenML zenml integration install mlflow -y #Register the experiment tracker zenml experiment-tracker register mlflow_tracker --flavor=mlflow #Registering the model deployer zenml model-deployer register mlflow --flavor=mlflow #Registering the stack zenml stack register local-mlflow-stack-new -a default -o default -d mlflow -e mlflow_tracker --set #To view the stack list zenml stack --list
> ingest_data()
函数中,以将其声明为我们训练管道的一步。以同样的方式,我们将在项目体系结构中为每个步骤编写代码并创建管道。bitcoin_price_prediction_mlops/ # Project directory ├── data/ │ └── management/ │ ├── api_to_mongodb.py # Code to fetch data and save it to MongoDB │ └── api.py # API-related utility functions │ ├── pipelines/ │ ├── deployment_pipeline.py # Deployment pipeline │ └── training_pipeline.py # Training pipeline │ ├── saved_models/ # Directory for storing trained models ├── saved_scalers/ # Directory for storing scalers used in data preprocessing │ ├── src/ # Source code │ ├── data_cleaning.py # Data cleaning and preprocessing │ ├── data_ingestion.py # Data ingestion │ ├── data_splitter.py # Data splitting │ ├── feature_engineering.py # Feature engineering │ ├── model_evaluation.py # Model evaluation │ └── model_training.py # Model training │ ├── steps/ # ZenML steps │ ├── clean_data.py # ZenML step for cleaning data │ ├── data_splitter.py # ZenML step for data splitting │ ├── dynamic_importer.py # ZenML step for importing dynamic data │ ├── feature_engineering.py # ZenML step for feature engineering │ ├── ingest_data.py # ZenML step for data ingestion │ ├── model_evaluation.py # ZenML step for model evaluation │ ├── model_training.py # ZenML step for training the model │ ├── prediction_service_loader.py # ZenML step for loading prediction services │ ├── predictor.py # ZenML step for prediction │ └── utils.py # Utility functions for steps │ ├── .env # Environment variables file ├── .gitignore # Git ignore file │ ├── app.py # Streamlit user interface app │ ├── README.md # Project documentation ├── requirements.txt # List of required packages ├── run_deployment.py # Code for running deployment and prediction pipeline ├── run_pipeline.py # Code for running training pipeline └── .zen/ # ZenML directory (created automatically after ZenML initialization)
>要查看我如何使用@Step 装饰器,请查看下面的github链接(步骤文件夹)以浏览管道其他步骤的代码,即数据清洁,功能工程,数据拆分,模型培训和模型评估。>>>>>>>>。 步骤5:数据清洁 在此步骤中,我们将创建清洁摄入数据的不同策略。我们将在数据中删除不需要的列和缺失值。>
import requests import pandas as pd from dotenv import load_dotenv import os # Load the .env file load_dotenv() def fetch_crypto_data(api_uri): response = requests.get( api_uri, params={ "market": "cadli", "instrument": "BTC-USD", "limit": 5000, "aggregate": 1, "fill": "true", "apply_mapping": "true", "response_format": "JSON" }, headers={"Content-type": "application/json; charset=UTF-8"} ) if response.status_code == 200: print('API Connection Successful! \nFetching the data...') data = response.json() data_list = data.get('Data', []) df = pd.DataFrame(data_list) df['DATE'] = pd.to_datetime(df['TIMESTAMP'], unit='s') return df # Return the DataFrame else: raise Exception(f"API Error: {response.status_code} - {response.text}")
>步骤6:功能工程
>此步骤从较早的data_cleaning步骤中获取已清洁的数据。我们正在创建新功能,例如简单的移动平均值(SMA),指数移动平均值(EMA)以及滞后和滚动统计数据,以捕获趋势,减少噪声并从时间序列数据中做出更可靠的预测。此外,我们使用MinMax缩放缩放特征和目标变量。
> import os from pymongo import MongoClient from dotenv import load_dotenv from data.management.api import fetch_crypto_data # Import the API function import pandas as pd load_dotenv() MONGO_URI = os.getenv("MONGO_URI") API_URI = os.getenv("API_URI") client = MongoClient(MONGO_URI, ssl=True, ssl_certfile=None, ssl_ca_certs=None) db = client['crypto_data'] collection = db['historical_data'] try: latest_entry = collection.find_one(sort=[("DATE", -1)]) # Find the latest date if latest_entry: last_date = pd.to_datetime(latest_entry['DATE']).strftime('%Y-%m-%d') else: last_date = '2011-03-27' # Default start date if MongoDB is empty print(f"Fetching data starting from {last_date}...") new_data_df = fetch_crypto_data(API_URI) if latest_entry: new_data_df = new_data_df[new_data_df['DATE'] > last_date] if not new_data_df.empty: data_to_insert = new_data_df.to_dict(orient='records') result = collection.insert_many(data_to_insert) print(f"Inserted {len(result.inserted_ids)} new records into MongoDB.") else: print("No new data to insert.") except Exception as e: print(f"An error occurred: {e}")
步骤7:数据拆分#create a virtual environment python3 -m venv venv #Activate your virtual environmnent in your project folder source venv/bin/activate
步骤8:模型培训。 >步骤9:模型评估 #Install zenml pip install zenml #To Launch zenml server and dashboard locally pip install "zenml[server]" #To check the zenml Version: zenml version #To initiate a new repository zenml init #To run the dashboard locally: zenml login --local #To know the status of our zenml Pipelines zenml show #To shutdown the zenml server zenml clean
这是一个回归问题,我们正在使用评估指标,例如均方误差(MSE),均方根误差(MSE),均值绝对误差(MAE)和R-squared。现在,我们将在管道中组织上述所有步骤。让我们创建一个新的文件triench_pipeline.py。
>#Integrating mlflow with ZenML zenml integration install mlflow -y #Register the experiment tracker zenml experiment-tracker register mlflow_tracker --flavor=mlflow #Registering the model deployer zenml model-deployer register mlflow --flavor=mlflow #Registering the stack zenml stack register local-mlflow-stack-new -a default -o default -d mlflow -e mlflow_tracker --set #To view the stack list zenml stack --list
@pipelinedecorator用于将functionml_pipeline()定义为zenml中的管道。
要查看训练管道的仪表板,只需运行run_pipeline.py脚本即可。让我们创建一个run_pipeline.py文件。bitcoin_price_prediction_mlops/ # Project directory ├── data/ │ └── management/ │ ├── api_to_mongodb.py # Code to fetch data and save it to MongoDB │ └── api.py # API-related utility functions │ ├── pipelines/ │ ├── deployment_pipeline.py # Deployment pipeline │ └── training_pipeline.py # Training pipeline │ ├── saved_models/ # Directory for storing trained models ├── saved_scalers/ # Directory for storing scalers used in data preprocessing │ ├── src/ # Source code │ ├── data_cleaning.py # Data cleaning and preprocessing │ ├── data_ingestion.py # Data ingestion │ ├── data_splitter.py # Data splitting │ ├── feature_engineering.py # Feature engineering │ ├── model_evaluation.py # Model evaluation │ └── model_training.py # Model training │ ├── steps/ # ZenML steps │ ├── clean_data.py # ZenML step for cleaning data │ ├── data_splitter.py # ZenML step for data splitting │ ├── dynamic_importer.py # ZenML step for importing dynamic data │ ├── feature_engineering.py # ZenML step for feature engineering │ ├── ingest_data.py # ZenML step for data ingestion │ ├── model_evaluation.py # ZenML step for model evaluation │ ├── model_training.py # ZenML step for training the model │ ├── prediction_service_loader.py # ZenML step for loading prediction services │ ├── predictor.py # ZenML step for prediction │ └── utils.py # Utility functions for steps │ ├── .env # Environment variables file ├── .gitignore # Git ignore file │ ├── app.py # Streamlit user interface app │ ├── README.md # Project documentation ├── requirements.txt # List of required packages ├── run_deployment.py # Code for running deployment and prediction pipeline ├── run_pipeline.py # Code for running training pipeline └── .zen/ # ZenML directory (created automatically after ZenML initialization)
>>现在我们已经完成了创建管道。在下面运行命令以查看管道仪表板。
在运行上述命令后,它将返回跟踪仪表板URL,看起来像这样。
import os import logging from pymongo import MongoClient from dotenv import load_dotenv from zenml import step import pandas as pd # Load the .env file load_dotenv() # Get MongoDB URI from environment variables MONGO_URI = os.getenv("MONGO_URI") def fetch_data_from_mongodb(collection_name:str, database_name:str): """ Fetches data from MongoDB and converts it into a pandas DataFrame. collection_name: Name of the MongoDB collection to fetch data. database_name: Name of the MongoDB database. return: A pandas DataFrame containing the data """ # Connect to the MongoDB client client = MongoClient(MONGO_URI) db = client[database_name] # Select the database collection = db[collection_name] # Select the collection # Fetch all documents from the collection try: logging.info(f"Fetching data from MongoDB collection: {collection_name}...") data = list(collection.find()) # Convert cursor to a list of dictionaries if not data: logging.info("No data found in the MongoDB collection.") # Convert the list of dictionaries into a pandas DataFrame df = pd.DataFrame(data) # Drop the MongoDB ObjectId field if it exists (optional) if '_id' in df.columns: df = df.drop(columns=['_id']) logging.info("Data successfully fetched and converted to a DataFrame!") return df except Exception as e: logging.error(f"An error occurred while fetching data: {e}") raise e @step(enable_cache=False) def ingest_data(collection_name: str = "historical_data", database_name: str = "crypto_data") -> pd.DataFrame: logging.info("Started data ingestion process from MongoDB.") try: # Use the fetch_data_from_mongodb function to fetch data df = fetch_data_from_mongodb(collection_name=collection_name, database_name=database_name) if df.empty: logging.warning("No data was loaded. Check the collection name or the database content.") else: logging.info(f"Data ingestion completed. Number of records loaded: {len(df)}.") return df except Exception as e: logging.error(f"Error while reading data from {collection_name} in {database_name}: {e}") raise e
class DataPreprocessor: def __init__(self, data: pd.DataFrame): self.data = data logging.info("DataPreprocessor initialized with data of shape: %s", data.shape) def clean_data(self) -> pd.DataFrame: """ Performs data cleaning by removing unnecessary columns, dropping columns with missing values, and returning the cleaned DataFrame. Returns: pd.DataFrame: The cleaned DataFrame with unnecessary and missing-value columns removed. """ logging.info("Starting data cleaning process.") # Drop unnecessary columns, including '_id' if it exists columns_to_drop = [ 'UNIT', 'TYPE', 'MARKET', 'INSTRUMENT', 'FIRST_MESSAGE_TIMESTAMP', 'LAST_MESSAGE_TIMESTAMP', 'FIRST_MESSAGE_VALUE', 'HIGH_MESSAGE_VALUE', 'HIGH_MESSAGE_TIMESTAMP', 'LOW_MESSAGE_VALUE', 'LOW_MESSAGE_TIMESTAMP', 'LAST_MESSAGE_VALUE', 'TOTAL_INDEX_UPDATES', 'VOLUME_TOP_TIER', 'QUOTE_VOLUME_TOP_TIER', 'VOLUME_DIRECT', 'QUOTE_VOLUME_DIRECT', 'VOLUME_TOP_TIER_DIRECT', 'QUOTE_VOLUME_TOP_TIER_DIRECT', '_id' # Adding '_id' to the list ] logging.info("Dropping columns: %s") self.data = self.drop_columns(self.data, columns_to_drop) # Drop columns where the number of missing values is greater than 0 logging.info("Dropping columns with missing values.") self.data = self.drop_columns_with_missing_values(self.data) logging.info("Data cleaning completed. Data shape after cleaning: %s", self.data.shape) return self.data def drop_columns(self, data: pd.DataFrame, columns: list) -> pd.DataFrame: """ Drops specified columns from the DataFrame. Returns: pd.DataFrame: The DataFrame with the specified columns removed. """ logging.info("Dropping columns: %s", columns) return data.drop(columns=columns, errors='ignore') def drop_columns_with_missing_values(self, data: pd.DataFrame) -> pd.DataFrame: """ Drops columns with any missing values from the DataFrame. Parameters: data: pd.DataFrame The DataFrame from which columns with missing values will be removed. Returns: pd.DataFrame: The DataFrame with columns containing missing values removed. """ missing_columns = data.columns[data.isnull().sum() > 0] if not missing_columns.empty: logging.info("Columns with missing values: %s", missing_columns.tolist()) else: logging.info("No columns with missing values found.") return data.loc[:, data.isnull().sum() == 0]
培训管道在仪表板上看起来像这样,给出了以下内容:>
步骤10:模型部署
>到目前为止,我们已经构建了模型和管道。现在,让我们将管道推入用户可以做出预测的生产中。
连续部署管道
该管道负责连续部署训练有素的模型。它首先从
> triagn_pipeline.py.py文件训练模型,然后使用
mlflow模型exployer来部署训练有素的模型,使用
推理管道 >我们使用推理管道使用已部署的模型对新数据进行预测。让我们看一下我们如何在项目中实施该管道的方式。
>import requests import pandas as pd from dotenv import load_dotenv import os # Load the .env file load_dotenv() def fetch_crypto_data(api_uri): response = requests.get( api_uri, params={ "market": "cadli", "instrument": "BTC-USD", "limit": 5000, "aggregate": 1, "fill": "true", "apply_mapping": "true", "response_format": "JSON" }, headers={"Content-type": "application/json; charset=UTF-8"} ) if response.status_code == 200: print('API Connection Successful! \nFetching the data...') data = response.json() data_list = data.get('Data', []) df = pd.DataFrame(data_list) df['DATE'] = pd.to_datetime(df['TIMESTAMP'], unit='s') return df # Return the DataFrame else: raise Exception(f"API Error: {response.status_code} - {response.text}")
>让我们查看下面的推理管道中调用的每个功能:
> dynamic_importer()
此功能加载新数据,执行数据处理并返回数据。import os from pymongo import MongoClient from dotenv import load_dotenv from data.management.api import fetch_crypto_data # Import the API function import pandas as pd load_dotenv() MONGO_URI = os.getenv("MONGO_URI") API_URI = os.getenv("API_URI") client = MongoClient(MONGO_URI, ssl=True, ssl_certfile=None, ssl_ca_certs=None) db = client['crypto_data'] collection = db['historical_data'] try: latest_entry = collection.find_one(sort=[("DATE", -1)]) # Find the latest date if latest_entry: last_date = pd.to_datetime(latest_entry['DATE']).strftime('%Y-%m-%d') else: last_date = '2011-03-27' # Default start date if MongoDB is empty print(f"Fetching data starting from {last_date}...") new_data_df = fetch_crypto_data(API_URI) if latest_entry: new_data_df = new_data_df[new_data_df['DATE'] > last_date] if not new_data_df.empty: data_to_insert = new_data_df.to_dict(orient='records') result = collection.insert_many(data_to_insert) print(f"Inserted {len(result.inserted_ids)} new records into MongoDB.") else: print("No new data to insert.") except Exception as e: print(f"An error occurred: {e}")
prediction_service_loader()>此功能以
@Step装饰。我们加载了基于pipeline_name的部署服务W.R.T和step_name,我们的部署模型可以处理新数据的预测查询。 > line 现有_services = mlflow_model_deployer_component.find_model_server()
>>基于诸如pipeline名称和管道名称的给定参数,搜索可用的部署服务。如果没有可用的服务,则表明部署管道要么没有进行或遇到部署管道问题,因此它会抛出RuntimeError。
preditionor()#create a virtual environment python3 -m venv venv #Activate your virtual environmnent in your project folder source venv/bin/activate
该函数通过MLFlowDeploymentservice和新数据采用MLFlow部署模型。进一步处理数据以匹配模型的预期格式以进行实时推断。 为了可视化连续部署和推理管道,我们需要运行run_deployment.py脚本,在此将定义部署和预测配置。 (请在下面给出的github中检查run_deployment.py代码)。>
现在,让我们运行run_deployment.py文件以查看连续部署管道和推理管道的仪表板。
#Install zenml pip install zenml #To Launch zenml server and dashboard locally pip install "zenml[server]" #To check the zenml Version: zenml version #To initiate a new repository zenml init #To run the dashboard locally: zenml login --local #To know the status of our zenml Pipelines zenml show #To shutdown the zenml server zenml clean
连续部署管道 - 输出
#Integrating mlflow with ZenML zenml integration install mlflow -y #Register the experiment tracker zenml experiment-tracker register mlflow_tracker --flavor=mlflow #Registering the model deployer zenml model-deployer register mlflow --flavor=mlflow #Registering the stack zenml stack register local-mlflow-stack-new -a default -o default -d mlflow -e mlflow_tracker --set #To view the stack list zenml stack --list
bitcoin_price_prediction_mlops/ # Project directory ├── data/ │ └── management/ │ ├── api_to_mongodb.py # Code to fetch data and save it to MongoDB │ └── api.py # API-related utility functions │ ├── pipelines/ │ ├── deployment_pipeline.py # Deployment pipeline │ └── training_pipeline.py # Training pipeline │ ├── saved_models/ # Directory for storing trained models ├── saved_scalers/ # Directory for storing scalers used in data preprocessing │ ├── src/ # Source code │ ├── data_cleaning.py # Data cleaning and preprocessing │ ├── data_ingestion.py # Data ingestion │ ├── data_splitter.py # Data splitting │ ├── feature_engineering.py # Feature engineering │ ├── model_evaluation.py # Model evaluation │ └── model_training.py # Model training │ ├── steps/ # ZenML steps │ ├── clean_data.py # ZenML step for cleaning data │ ├── data_splitter.py # ZenML step for data splitting │ ├── dynamic_importer.py # ZenML step for importing dynamic data │ ├── feature_engineering.py # ZenML step for feature engineering │ ├── ingest_data.py # ZenML step for data ingestion │ ├── model_evaluation.py # ZenML step for model evaluation │ ├── model_training.py # ZenML step for training the model │ ├── prediction_service_loader.py # ZenML step for loading prediction services │ ├── predictor.py # ZenML step for prediction │ └── utils.py # Utility functions for steps │ ├── .env # Environment variables file ├── .gitignore # Git ignore file │ ├── app.py # Streamlit user interface app │ ├── README.md # Project documentation ├── requirements.txt # List of required packages ├── run_deployment.py # Code for running deployment and prediction pipeline ├── run_pipeline.py # Code for running training pipeline └── .zen/ # ZenML directory (created automatically after ZenML initialization)
>推理管道 - 输出现在,您需要在命令行中复制并粘贴上述MLFLOW UI链接并运行它。
这是MLFlow仪表板,您可以在其中看到评估指标和模型参数:import os import logging from pymongo import MongoClient from dotenv import load_dotenv from zenml import step import pandas as pd # Load the .env file load_dotenv() # Get MongoDB URI from environment variables MONGO_URI = os.getenv("MONGO_URI") def fetch_data_from_mongodb(collection_name:str, database_name:str): """ Fetches data from MongoDB and converts it into a pandas DataFrame. collection_name: Name of the MongoDB collection to fetch data. database_name: Name of the MongoDB database. return: A pandas DataFrame containing the data """ # Connect to the MongoDB client client = MongoClient(MONGO_URI) db = client[database_name] # Select the database collection = db[collection_name] # Select the collection # Fetch all documents from the collection try: logging.info(f"Fetching data from MongoDB collection: {collection_name}...") data = list(collection.find()) # Convert cursor to a list of dictionaries if not data: logging.info("No data found in the MongoDB collection.") # Convert the list of dictionaries into a pandas DataFrame df = pd.DataFrame(data) # Drop the MongoDB ObjectId field if it exists (optional) if '_id' in df.columns: df = df.drop(columns=['_id']) logging.info("Data successfully fetched and converted to a DataFrame!") return df except Exception as e: logging.error(f"An error occurred while fetching data: {e}") raise e @step(enable_cache=False) def ingest_data(collection_name: str = "historical_data", database_name: str = "crypto_data") -> pd.DataFrame: logging.info("Started data ingestion process from MongoDB.") try: # Use the fetch_data_from_mongodb function to fetch data df = fetch_data_from_mongodb(collection_name=collection_name, database_name=database_name) if df.empty: logging.warning("No data was loaded. Check the collection name or the database content.") else: logging.info(f"Data ingestion completed. Number of records loaded: {len(df)}.") return df except Exception as e: logging.error(f"Error while reading data from {collection_name} in {database_name}: {e}") raise e
>这是对项目的GitHub代码和视频说明,以便您更好地理解。
结论
在本文中,我们成功地建立了一个端到端的,可提供生产的比特币价格预测MLOPS项目。从通过API获取数据并预处理数据到模型培训,评估和部署,我们的项目突出了MLOP在将开发与生产联系起来的关键作用。我们距离实时预测比特币价格的未来更近了一步。 API提供了对外部数据的平稳访问,例如CCDATA API的比特币价格数据,消除了对预先存在的数据集的需求。- >
- > zenml和mlflow是可靠的工具,可促进现实世界应用程序中机器学习模型的开发,跟踪和部署。
- >我们遵循最佳实践,通过正确执行数据摄入,清洁,功能工程,模型培训和评估。 >连续部署和推理管道对于确保模型保持有效且在生产环境中可用。
- 常见问题
- > Q1。 Zenml可以免费使用吗?是的,Zenml是一个完全开源的MLOPS框架,它使从本地开发到生产管道的过渡与1行代码一样容易。
Q2。 mlflow用于什么? MLFlow通过提供用于跟踪实验,版本控制模型和部署的工具来使机器学习开发变得更加容易。
Q3。如何调试服务器守护程序没有运行错误?这是您将在项目中面临的常见错误。只需运行`Zenml注销–Local`然后`Zenml clean'',然后再运行管道。它将得到解决。
以上是使用MLOPS的比特币价格预测的详细内容。更多信息请关注PHP中文网其他相关文章!
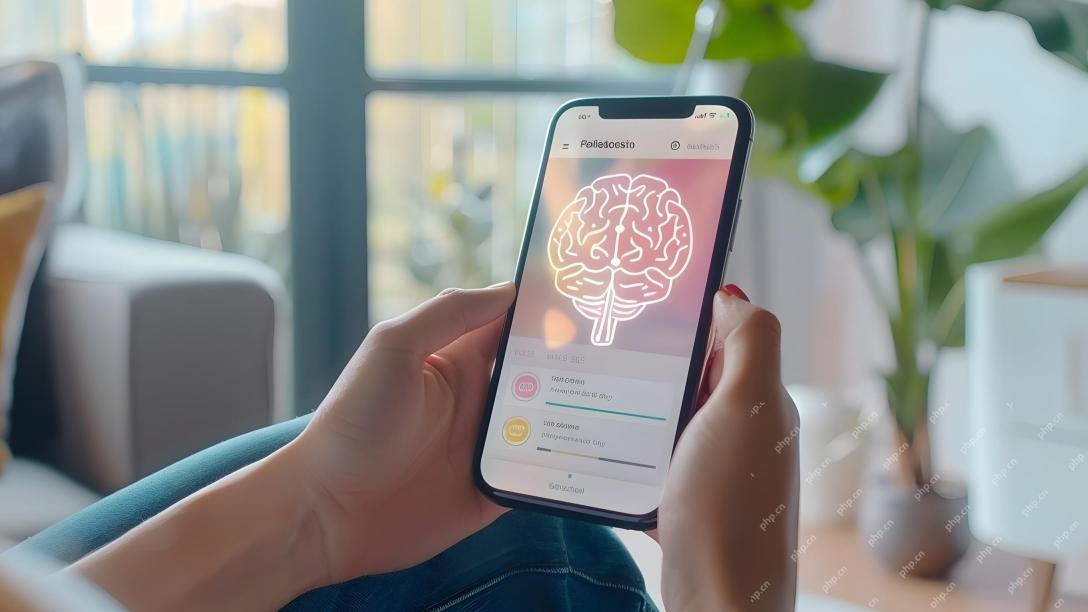
尽管它无法提供训练有素的治疗师的人类联系和直觉,但研究表明,许多人很乐意与相对无面和匿名的AI机器人分享他们的担忧和担忧。 这是否总是好我
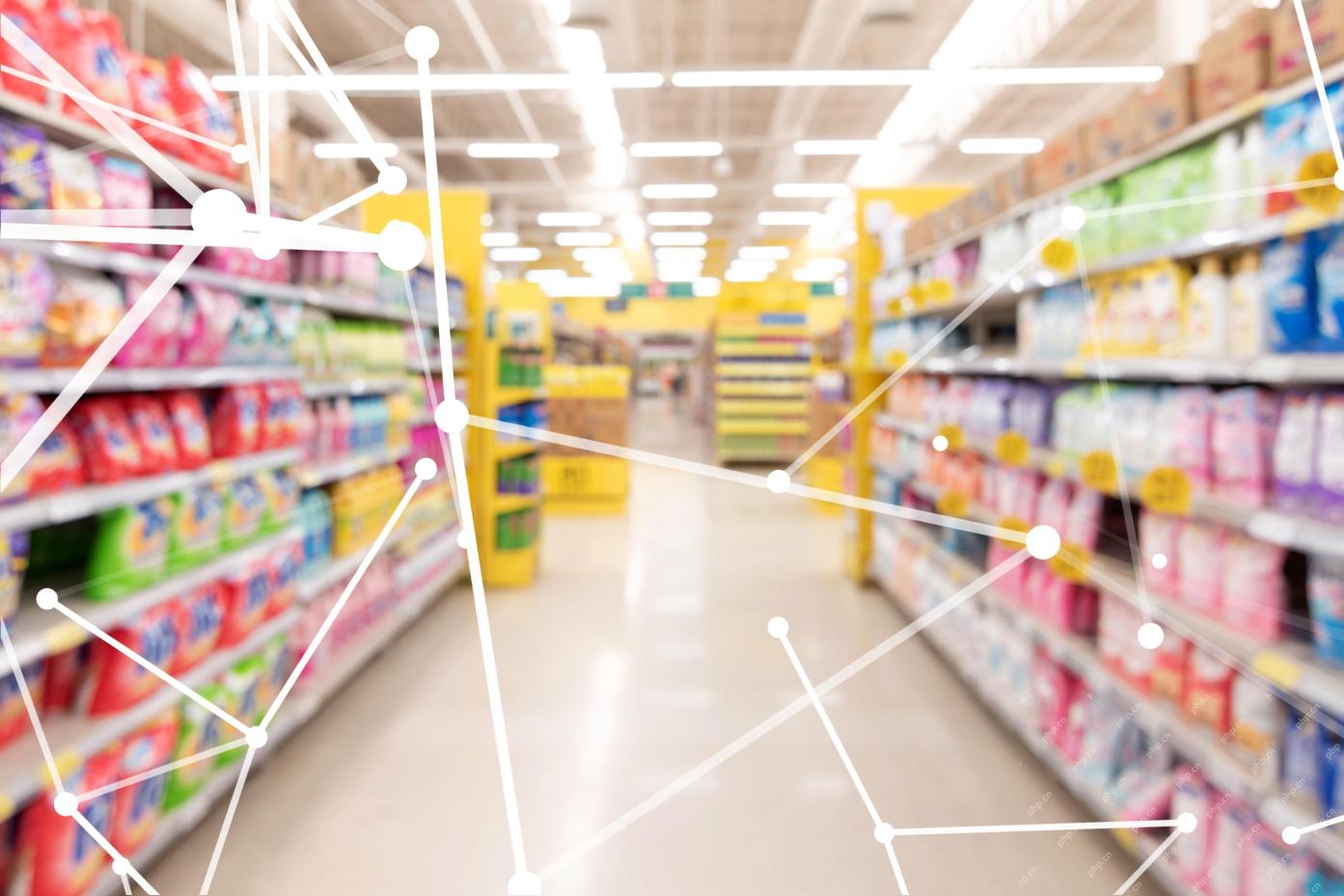
人工智能(AI)是一种技术数十年的技术,正在彻底改变食品零售业。 从大规模的效率提高和成本降低到精简的各种业务功能的流程,AI的影响是Undeniabl
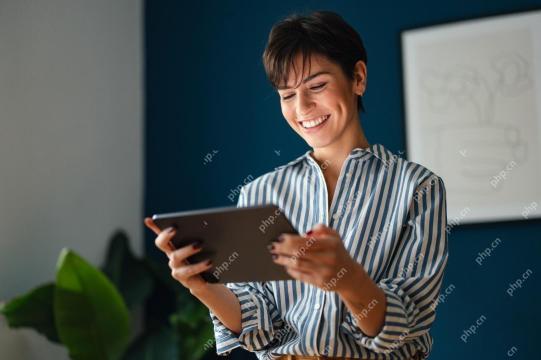
让我们来谈谈。 对创新AI突破的分析是我正在进行的AI中正在进行的福布斯列覆盖的一部分,包括识别和解释各种有影响力的AI复杂性(请参阅此处的链接)。此外,对于我的comp
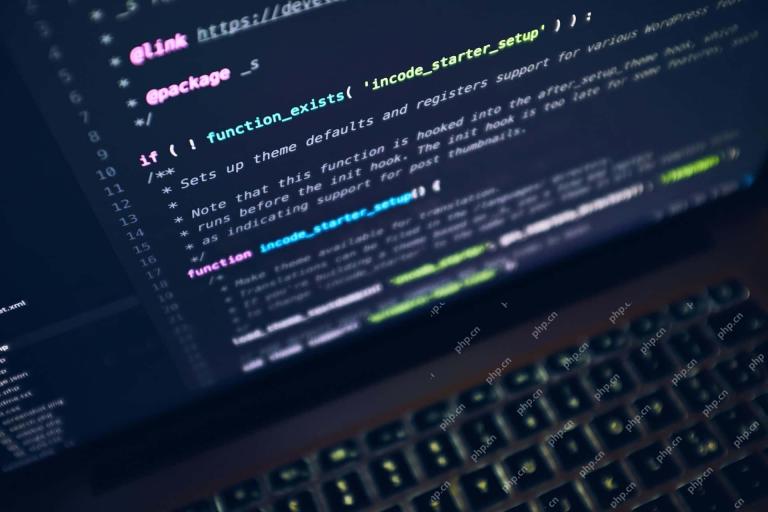
保持专业形象需要偶尔的衣柜更新。 在线购物方便时,它缺乏面对面尝试的确定性。 我的解决方案? AI驱动的个性化。 我设想AI助手策划服装Selecti
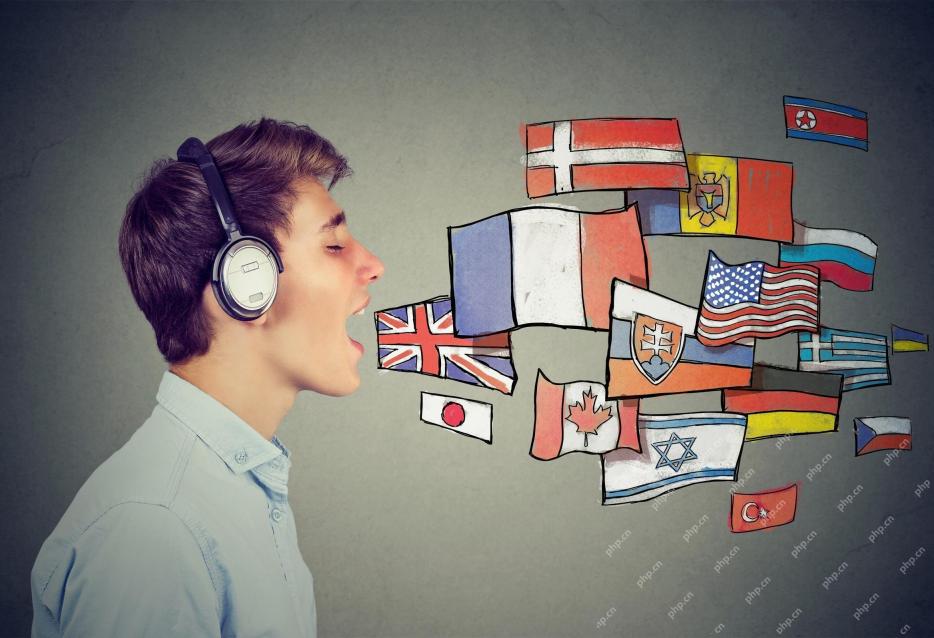
谷歌翻译新增语言学习功能 据Android Authority报道,应用专家AssembleDebug发现,最新版本的谷歌翻译应用包含一个新的“练习”模式的测试代码,旨在帮助用户通过个性化活动来提高他们的语言技能。此功能目前对用户不可见,但AssembleDebug能够部分激活它并查看其一些新的用户界面元素。 激活后,该功能会在屏幕底部添加一个新的“毕业帽”图标,标有“Beta”徽章,表明“练习”功能最初将以实验形式发布。 相关的弹出提示显示“练习为你量身定制的活动!”,这意味着谷歌将生成定制的
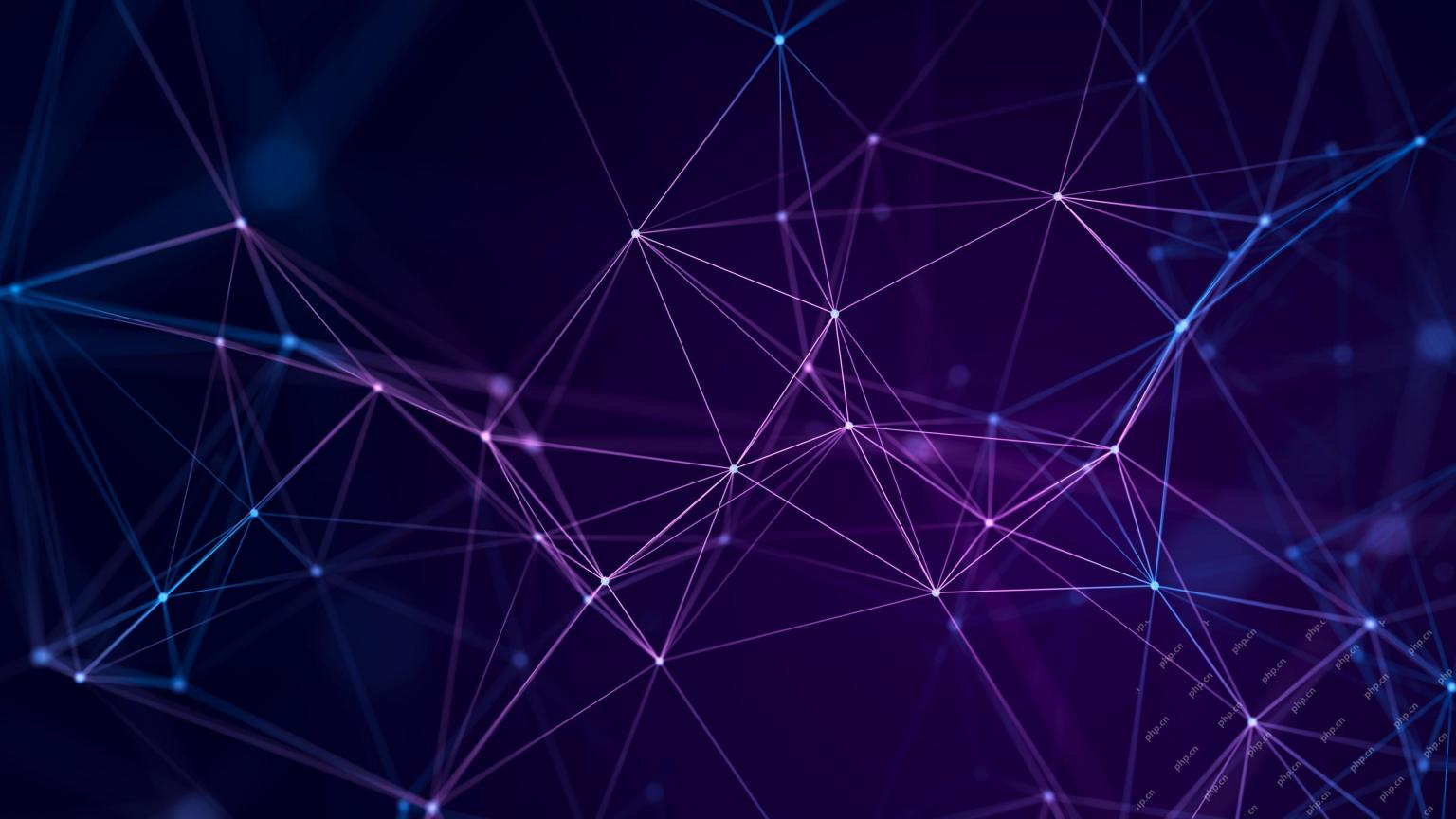
麻省理工学院的研究人员正在开发Nanda,这是为AI代理设计的开创性的Web协议。 Nanda的缩写是网络代理和分散的AI,通过添加Internet功能,使AI Agen能够构建人类的模型上下文协议(MCP)。
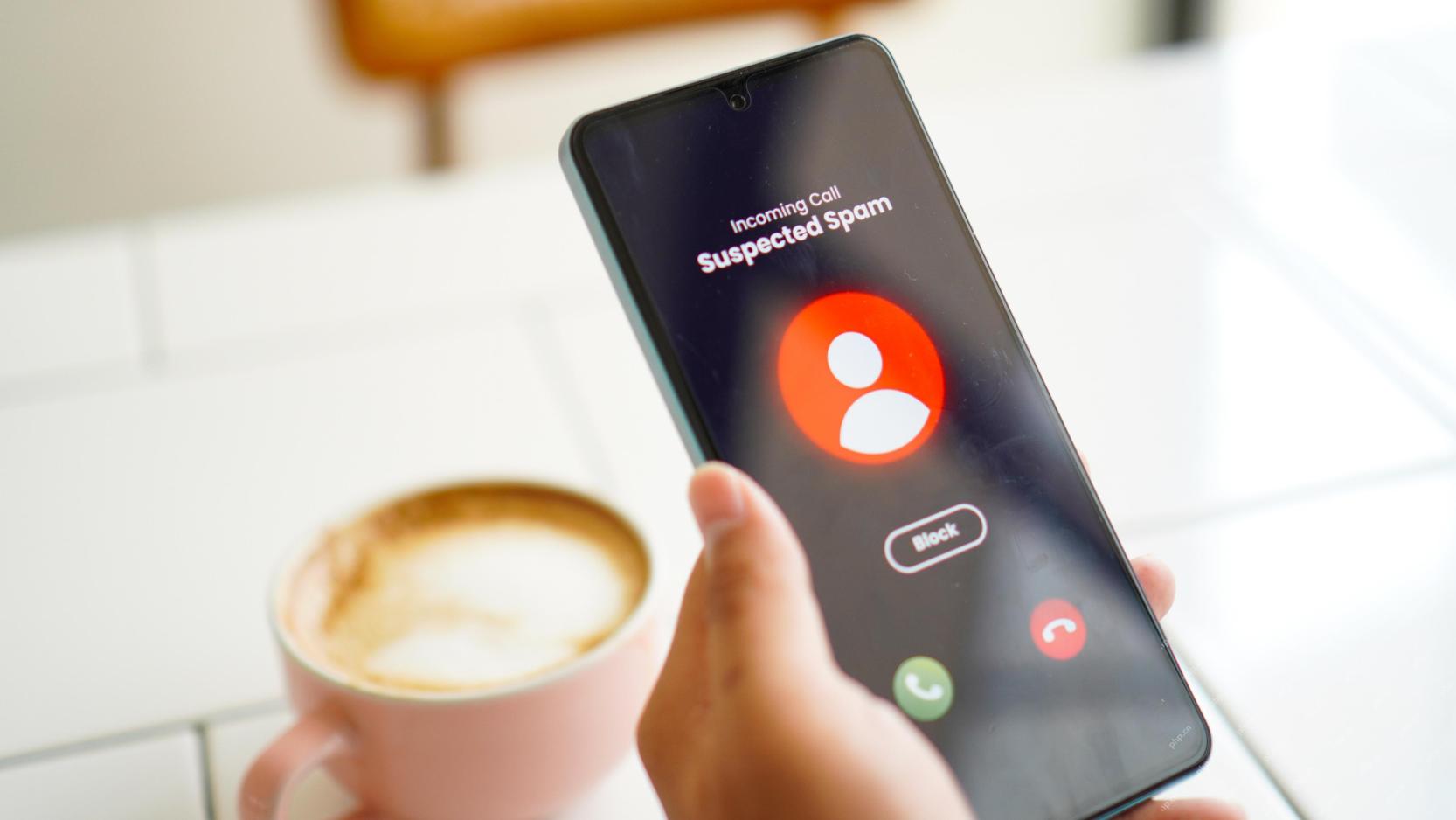
Meta的最新冒险:与Chatgpt竞争的AI应用程序 Facebook,Instagram,WhatsApp和Threads的母公司Meta正在启动新的AI功能应用程序。 这个独立的应用程序Meta AI旨在直接与Openai的Chatgpt竞争。 杠杆
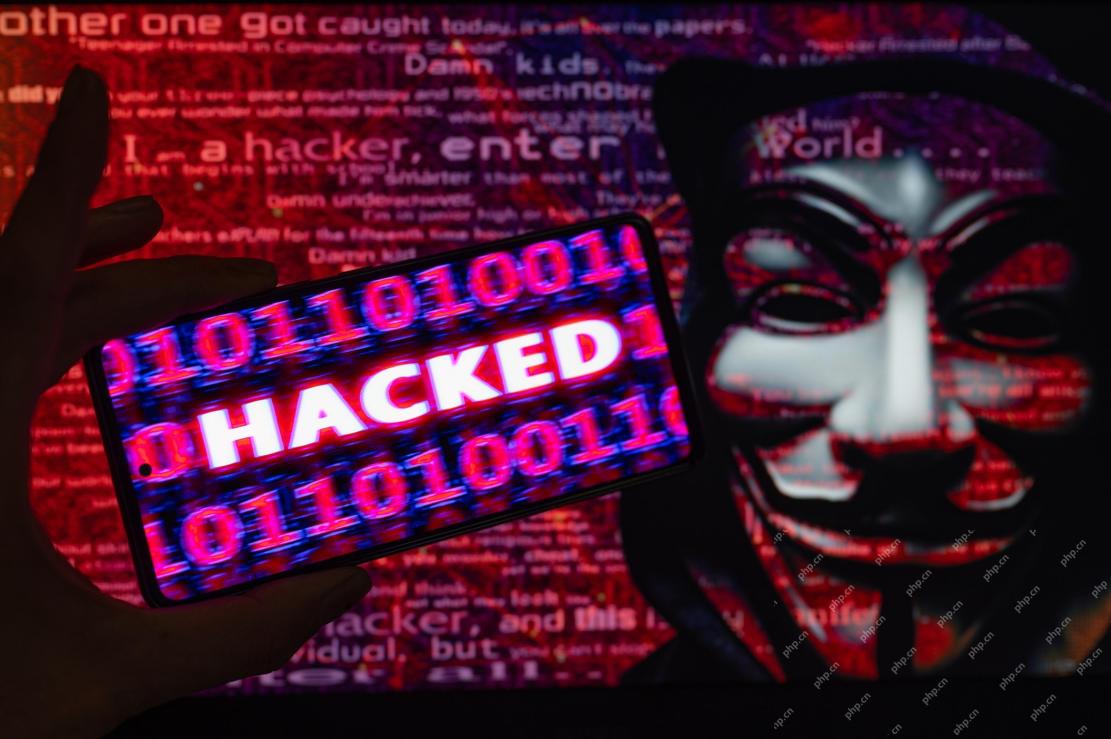
导航AI网络攻击的上升潮流 最近,CISO的杰森·克林顿(Jason Clinton)拟人化,强调了与非人类身份相关的新兴风险 - 作为机器对机器的通信增殖,维护这些“身份”


热AI工具

Undresser.AI Undress
人工智能驱动的应用程序,用于创建逼真的裸体照片

AI Clothes Remover
用于从照片中去除衣服的在线人工智能工具。

Undress AI Tool
免费脱衣服图片

Clothoff.io
AI脱衣机

Video Face Swap
使用我们完全免费的人工智能换脸工具轻松在任何视频中换脸!

热门文章

热工具
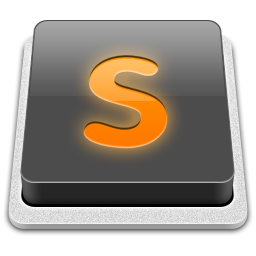
SublimeText3 Mac版
神级代码编辑软件(SublimeText3)

SublimeText3汉化版
中文版,非常好用
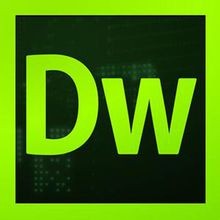
Dreamweaver CS6
视觉化网页开发工具

记事本++7.3.1
好用且免费的代码编辑器
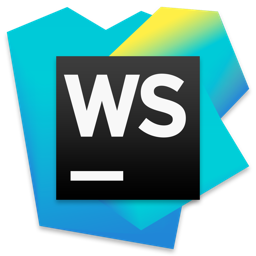
WebStorm Mac版
好用的JavaScript开发工具