如何对数据框进行透视?
什么是透视?
透视是一种用于通过交换行和列来重塑 DataFrame 的数据转换技术。它通常用于以更易于分析或可视化的方式组织数据。
如何进行数据透视?
有多种方法可以在其中透视 DataFrame使用 Pandas 库的 Python:
1. pd.DataFrame.pivot_table:
此方法是用于旋转数据的多功能且功能丰富的选项。它允许您指定要聚合的值、聚合函数以及行索引和列索引。
示例:
import pandas as pd # Create a sample DataFrame df = pd.DataFrame({ "row": ["row0", "row1", "row2", "row3", "row4"], "col": ["col0", "col1", "col2", "col3", "col4"], "val0": [0.81, 0.44, 0.77, 0.15, 0.81], "val1": [0.04, 0.07, 0.01, 0.59, 0.64] }) # Pivot the DataFrame using pivot_table df_pivoted = df.pivot_table( index="row", columns="col", values="val0", aggfunc="mean", ) print(df_pivoted) # Output: col0 col1 col2 col3 col4 row row0 0.77 0.445 0.000 0.860 0.650 row1 0.130 0.000 0.395 0.500 0.250 row2 0.000 0.310 0.000 0.545 0.000 row3 0.000 0.100 0.395 0.760 0.240 row4 0.000 0.000 0.000 0.000 0.000
2. pd.DataFrame.groupby pd.DataFrame.unstack:
此方法涉及按所需的行和列索引对 DataFrame 进行分组,然后使用 unstack 来旋转分组的数据。
示例:
# Group the DataFrame by row and col df_grouped = df.groupby(["row", "col"]) # Perform pivot using unstack df_pivoted = df_grouped["val0"].unstack(fill_value=0) print(df_pivoted) # Output: col col0 col1 col2 col3 col4 row row0 0.81 0.445 0.000 0.860 0.650 row1 0.130 0.000 0.395 0.500 0.250 row2 0.000 0.310 0.000 0.545 0.000 row3 0.000 0.100 0.395 0.760 0.240 row4 0.000 0.000 0.000 0.000 0.000
3. pd.DataFrame.set_index pd.DataFrame.unstack:
此方法涉及将所需的行和列索引设置为 DataFrame 的索引,然后使用 unstack 来旋转数据。
示例:
# Set the row and col as the DataFrame's index df = df.set_index(["row", "col"]) # Perform pivot using unstack df_pivoted = df["val0"].unstack(fill_value=0) print(df_pivoted) # Output: col col0 col1 col2 col3 col4 row row0 0.81 0.445 0.000 0.860 0.650 row1 0.130 0.000 0.395 0.500 0.250 row2 0.000 0.310 0.000 0.545 0.000 row3 0.000 0.100 0.395 0.760 0.240 row4 0.000 0.000 0.000 0.000 0.000
4. pd.DataFrame.pivot:
与pivot_table相比,此方法提供了更简单的语法,但功能有限。它只允许您指定行索引和列索引,并且不能执行聚合。
示例:
# Perform pivot using pivot df_pivoted = df.pivot(index="row", columns="col") print(df_pivoted) # Output: col col0 col1 col2 col3 col4 row row0 key0 0.81 0.44 0.00 0.86 0.65 row1 key1 0.13 0.00 0.39 0.50 0.25 row2 key1 0.00 0.31 0.00 0.54 0.00 row3 key0 0.00 0.10 0.39 0.76 0.24 row4 key1 0.00 0.00 0.00 0.00 0.00
长格式转宽格式
仅使用两列将 DataFrame 从长格式转换为宽格式:
1. pd.DataFrame.pivot(index=column_to_index, columns=column_to_columns, values=values_to_pivot**):
示例:
df["Combined"] = df["row"] + "|" + df["col"] df_pivoted = df.pivot(index="Combined", columns="A", values="B") print(df_pivoted) # Output: A a b c Combined row0|col0 0.0 10.0 7.0 row1|col1 11.0 10.0 NaN row2|col2 2.0 14.0 NaN row3|col3 11.0 NaN NaN row4|col4 NaN NaN NaN
2. pd.DataFrame.groupby pd.DataFrame.unstack:
df["Combined"] = df["row"] + "|" + df["col"] df_grouped = df.groupby(["Combined", "A"]) df_pivoted = df_grouped["B"].unstack(fill_value=0) print(df_pivoted) # Output: A a b c Combined row0|col0 0.0 10.0 7.0 row1|col1 11.0 10.0 NaN row2|col2 2.0 14.0 NaN row3|col3 11.0 NaN NaN row4|col4 NaN NaN NaN
在透视后将多个索引展平为单个索引:
df_pivoted.columns = df_pivoted.columns.map("|".join) print(df_pivoted) # Output: a|col0 b|col0 c|col0 a|col1 b|col1 c|col1 a|col2 b|col2 c|col2 a|col3 b|col3 c|col3 row row0 0.0 10.0 7.0 11.0 10.0 NaN 2.0 14.0 NaN 11.0 NaN NaN row1 0.0 10.0 7.0 11.0 10.0 NaN 2.0 14.0 NaN 11.0 NaN NaN
以上是如何在 Python 中透视 Pandas DataFrame?的详细内容。更多信息请关注PHP中文网其他相关文章!
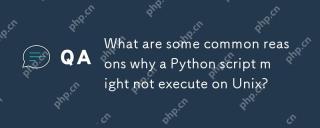
Python脚本在Unix系统上无法运行的原因包括:1)权限不足,使用chmod xyour_script.py赋予执行权限;2)Shebang行错误或缺失,应使用#!/usr/bin/envpython;3)环境变量设置不当,可打印os.environ调试;4)使用错误的Python版本,可在Shebang行或命令行指定版本;5)依赖问题,使用虚拟环境隔离依赖;6)语法错误,使用python-mpy_compileyour_script.py检测。
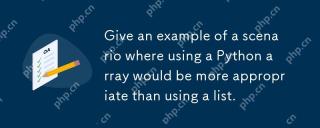
使用Python数组比列表更适合处理大量数值数据。1)数组更节省内存,2)数组对数值运算更快,3)数组强制类型一致性,4)数组与C语言数组兼容,但在灵活性和便捷性上不如列表。
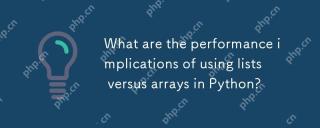
列表列表更好的forflexibility andmixDatatatypes,何时出色的Sumerical Computitation sand larged数据集。1)不可使用的列表xbilese xibility xibility xibility xibility xibility xibility xibility xibility xibility xibility xibles and comply offrequent elementChanges.2)
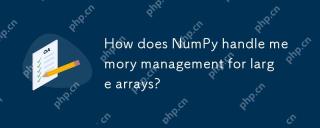
numpymanagesmemoryforlargearraysefefticefticefipedlyuseviews,副本和内存模拟文件.1)viewsAllowSinglicingWithOutCopying,直接modifytheoriginalArray.2)copiesCanbecopy canbecreatedwitheDedwithTheceDwithThecevithThece()methodervingdata.3)metservingdata.3)memore memore-mappingfileShessandAstaStaStstbassbassbassbassbassbassbassbassbassbassbb
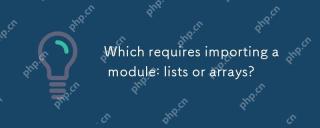
Listsinpythondonotrequireimportingamodule,helilearraysfomthearraymoduledoneedanimport.1)列表列表,列表,多功能和canholdMixedDatatatepes.2)arraysaremoremoremoremoremoremoremoremoremoremoremoremoremoremoremoremoremeremeremeremericdatabuteffeftlessdatabutlessdatabutlessfiblesible suriplyElsilesteletselementEltecteSemeTemeSemeSemeSemeTypysemeTypysemeTysemeTypysemeTypepe。
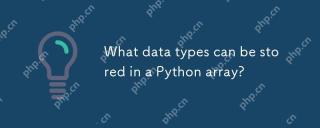
pythonlistscanStoryDatatepe,ArrayModulearRaysStoreOneType,and numpyArraySareSareAraysareSareAraysareSareComputations.1)列出sareversArversAtileButlessMemory-Felide.2)arraymoduleareareMogeMogeNareSaremogeNormogeNoreSoustAta.3)
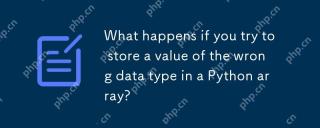
WhenyouattempttostoreavalueofthewrongdatatypeinaPythonarray,you'llencounteraTypeError.Thisisduetothearraymodule'sstricttypeenforcement,whichrequiresallelementstobeofthesametypeasspecifiedbythetypecode.Forperformancereasons,arraysaremoreefficientthanl
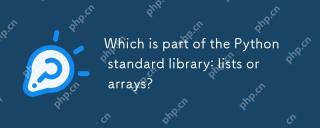
pythonlistsarepartofthestAndArdLibrary,herilearRaysarenot.listsarebuilt-In,多功能,和Rused ForStoringCollections,而EasaraySaraySaraySaraysaraySaraySaraysaraySaraysarrayModuleandleandleandlesscommonlyusedDduetolimitedFunctionalityFunctionalityFunctionality。


热AI工具

Undresser.AI Undress
人工智能驱动的应用程序,用于创建逼真的裸体照片

AI Clothes Remover
用于从照片中去除衣服的在线人工智能工具。

Undress AI Tool
免费脱衣服图片

Clothoff.io
AI脱衣机

Video Face Swap
使用我们完全免费的人工智能换脸工具轻松在任何视频中换脸!

热门文章

热工具
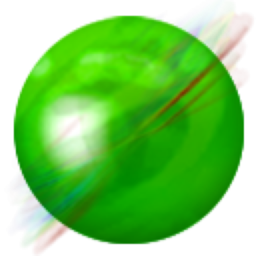
ZendStudio 13.5.1 Mac
功能强大的PHP集成开发环境

记事本++7.3.1
好用且免费的代码编辑器

DVWA
Damn Vulnerable Web App (DVWA) 是一个PHP/MySQL的Web应用程序,非常容易受到攻击。它的主要目标是成为安全专业人员在合法环境中测试自己的技能和工具的辅助工具,帮助Web开发人员更好地理解保护Web应用程序的过程,并帮助教师/学生在课堂环境中教授/学习Web应用程序安全。DVWA的目标是通过简单直接的界面练习一些最常见的Web漏洞,难度各不相同。请注意,该软件中
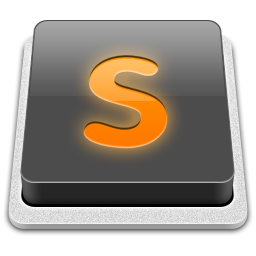
SublimeText3 Mac版
神级代码编辑软件(SublimeText3)

SublimeText3 英文版
推荐:为Win版本,支持代码提示!