使用Python数组比列表更适合处理大量数值数据。1)数组更节省内存,2)数组对数值运算更快,3)数组强制类型一致性,4)数组与C语言数组兼容,但在灵活性和便捷性上不如列表。
When it comes to choosing between a Python list and an array, understanding the nuances can significantly impact the performance and efficiency of your code. Let's dive into a scenario where using a Python array from the array
module would be more appropriate than using a list.
Imagine you're working on a project that involves processing large amounts of numerical data, such as a financial application that needs to handle stock prices or a scientific computing task dealing with sensor data. In such cases, using a Python array can offer substantial benefits over a list.
Here's a detailed exploration of why and how to use arrays effectively in this context:
In the world of Python, lists are incredibly versatile and easy to use. They can store elements of different types, grow or shrink dynamically, and are generally the go-to choice for many programming tasks. But what if you're dealing with a specific kind of data, like numbers, and performance matters a lot?
Let's say you're developing a financial application that processes millions of stock prices. Each stock price is a floating-point number, and you need to perform calculations on these numbers quickly. Here's where the array
module comes into play.
The array
module provides an array
object that is more memory-efficient and faster for numerical operations compared to a list. Unlike lists, which can contain elements of any type, arrays are typed, meaning they can only store elements of a single type. This restriction allows for more efficient memory usage and faster access times.
Here's a simple example to illustrate the difference:
import array import sys # Using a list to store numbers numbers_list = [1.0, 2.0, 3.0, 4.0, 5.0] print(f"Size of list: {sys.getsizeof(numbers_list)} bytes") # Using an array to store numbers numbers_array = array.array('d', [1.0, 2.0, 3.0, 4.0, 5.0]) print(f"Size of array: {sys.getsizeof(numbers_array)} bytes")
When you run this code, you'll notice that the array takes up less memory than the list. This difference becomes even more significant as the size of the data increases.
Now, let's consider a more practical scenario in our financial application:
import array import time # Simulating a large dataset of stock prices stock_prices_list = [float(i) for i in range(1000000)] stock_prices_array = array.array('d', [float(i) for i in range(1000000)]) # Measuring time to sum up all prices using a list start_time = time.time() total_list = sum(stock_prices_list) list_time = time.time() - start_time # Measuring time to sum up all prices using an array start_time = time.time() total_array = sum(stock_prices_array) array_time = time.time() - start_time print(f"Sum using list: {total_list}, Time: {list_time:.6f} seconds") print(f"Sum using array: {total_array}, Time: {array_time:.6f} seconds")
In this example, you'll likely see that the array performs the summation faster than the list, especially as the size of the dataset grows. This is because arrays are more optimized for numerical operations.
But it's not just about performance. Here are some additional considerations:
- Memory Efficiency: Arrays use less memory than lists for storing numerical data, which is crucial when dealing with large datasets.
- Type Safety: Arrays enforce type consistency, which can prevent bugs that might occur if you accidentally mix data types in a list.
- Interoperability: Arrays can be easily converted to and from C arrays, making them useful when interfacing with C libraries or when you need to optimize certain parts of your code.
However, there are some potential pitfalls to watch out for:
- Limited Flexibility: Since arrays are typed, you can't mix different types of data within the same array. This might limit their use in more general-purpose scenarios.
-
Less Convenient: Arrays don't support some of the convenient methods that lists do, like
append
orinsert
. You'll need to useextend
to add elements, which can be less intuitive.
In practice, I've found that the choice between lists and arrays often comes down to the specific needs of your project. For general-purpose programming, lists are usually the better choice due to their flexibility. But when you're dealing with large datasets of numerical data and performance is critical, arrays can be a game-changer.
To wrap up, if you're working on a project that involves processing millions of numbers quickly and efficiently, consider using a Python array from the array
module. It might just be the edge you need to optimize your code and make your application run faster and more smoothly.
以上是举一个场景的示例,其中使用Python数组比使用列表更合适。的详细内容。更多信息请关注PHP中文网其他相关文章!
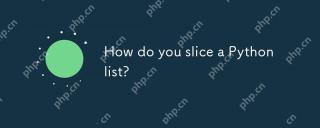
SlicingaPythonlistisdoneusingthesyntaxlist[start:stop:step].Here'showitworks:1)Startistheindexofthefirstelementtoinclude.2)Stopistheindexofthefirstelementtoexclude.3)Stepistheincrementbetweenelements.It'susefulforextractingportionsoflistsandcanuseneg
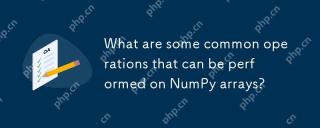
numpyallowsforvariousoperationsonArrays:1)basicarithmeticlikeaddition,减法,乘法和division; 2)evationAperationssuchasmatrixmultiplication; 3)element-wiseOperations wiseOperationswithOutexpliitloops; 4)
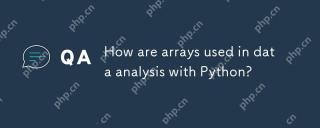
Arresinpython,尤其是Throughnumpyandpandas,weessentialFordataAnalysis,offeringSpeedAndeffied.1)NumpyArseNable efflaysenable efficefliceHandlingAtaSetSetSetSetSetSetSetSetSetSetSetsetSetSetSetSetsopplexoperationslikemovingaverages.2)
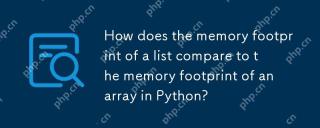
列表sandnumpyArraysInpyThonHavedIfferentMemoryfootprints:listSaremoreFlexibleButlessMemory-效率,而alenumpyArraySareSareOptimizedFornumericalData.1)listsStorReereReereReereReereFerenceStoObjects,withoverHeadeBheadaroundAroundaroundaround64bytaround64bitson64-bitsysysysyssyssyssyssyssyssysssys2)
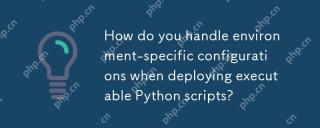
toensurepythonscriptsbehavecorrectlyacrycrossdevelvermations,登台和生产,USETHESTERTATE:1)Environment varriablesforsimplesettings,2)configurationFilesForefilesForcomPlexSetups,3)dynamiCofforAdaptapity.eachmethodofferSuniquebeneiquebeneiquebeneniqueBenefitsaniqueBenefitsandrefitsandRequiresandRequireSandRequireSca
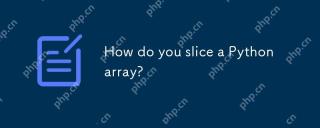
Python列表切片的基本语法是list[start:stop:step]。1.start是包含的第一个元素索引,2.stop是排除的第一个元素索引,3.step决定元素之间的步长。切片不仅用于提取数据,还可以修改和反转列表。
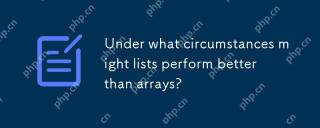
ListSoutPerformarRaysin:1)DynamicsizicsizingandFrequentInsertions/删除,2)储存的二聚体和3)MemoryFeliceFiceForceforseforsparsedata,butmayhaveslightperformancecostsinclentoperations。
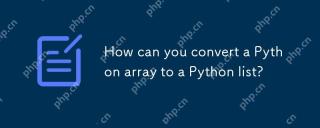
toConvertapythonarraytoalist,usEthelist()constructororageneratorexpression.1)intimpthearraymoduleandcreateanArray.2)USELIST(ARR)或[XFORXINARR] to ConconverTittoalist,请考虑performorefformanceandmemoryfformanceandmemoryfformienceforlargedAtasetset。


热AI工具

Undresser.AI Undress
人工智能驱动的应用程序,用于创建逼真的裸体照片

AI Clothes Remover
用于从照片中去除衣服的在线人工智能工具。

Undress AI Tool
免费脱衣服图片

Clothoff.io
AI脱衣机

Video Face Swap
使用我们完全免费的人工智能换脸工具轻松在任何视频中换脸!

热门文章

热工具

SublimeText3汉化版
中文版,非常好用
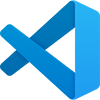
VSCode Windows 64位 下载
微软推出的免费、功能强大的一款IDE编辑器
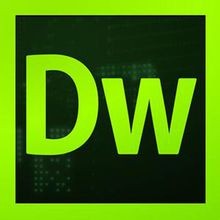
Dreamweaver CS6
视觉化网页开发工具
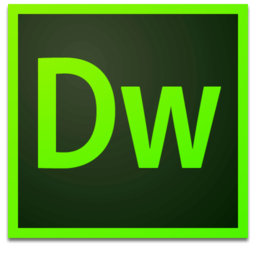
Dreamweaver Mac版
视觉化网页开发工具

SublimeText3 Linux新版
SublimeText3 Linux最新版