Lajur AIxiv ialah lajur di mana kandungan akademik dan teknikal diterbitkan di laman web ini. Dalam beberapa tahun kebelakangan ini, lajur AIxiv laman web ini telah menerima lebih daripada 2,000 laporan, meliputi makmal terkemuka dari universiti dan syarikat utama di seluruh dunia, mempromosikan pertukaran dan penyebaran akademik secara berkesan. Jika anda mempunyai kerja yang sangat baik yang ingin anda kongsikan, sila berasa bebas untuk menyumbang atau hubungi kami untuk melaporkan. E-mel penyerahan: liyazhou@jiqizhixin.com; zhaoyunfeng@jiqizhixin.com
Dalam beberapa tahun kebelakangan ini, ekosistem video pendek telah muncul dengan pantas, dan alatan kreatif dan penyuntingan di sekitar video pendek, Wink Meitu yang profesional alat penyuntingan video mudah alih, mendahului dengan keupayaan pemulihan kualiti video yang unik, dan bilangan pengguna di dalam dan luar negara terus meningkat. Di sebalik populariti fungsi pembaikan kualiti imej Wink adalah cerapan Meitu tentang titik kesakitan penciptaan video pengguna seperti imej kabur, bunyi yang teruk dan kualiti imej yang rendah di tengah-tengah pelepasan permintaan yang dipercepatkan untuk aplikasi pengeditan video masa, ia juga berdasarkan pemulihan video yang berkuasa dan sokongan teknologi peningkatan video Meitu Imaging Research Institute (MT Lab) Ia kini telah melancarkan Pemulihan Kualiti Imej - HD, Pemulihan Kualiti Imej - Ultra HD, Pemulihan Kualiti Imej - Peningkatan Potret, dan Penyelesaian peningkatan kadar dan fungsi lain. Baru-baru ini, Institut Penyelidikan Pengimejan Meitu (MT Lab) dan Akademi Sains Universiti China telah mencadangkan kaedah penyahliputan video buta (BVD) berasaskan STE BlazeBVD, yang merupakan pemecah tanah untuk menangani kemerosotan kerlipan pencahayaan video berkualiti rendah yang tidak diketahui, yang mengekalkan integriti kandungan dan warna video asal sebanyak mungkin, telah diterima oleh persidangan penglihatan komputer teratas ECCV 2024. 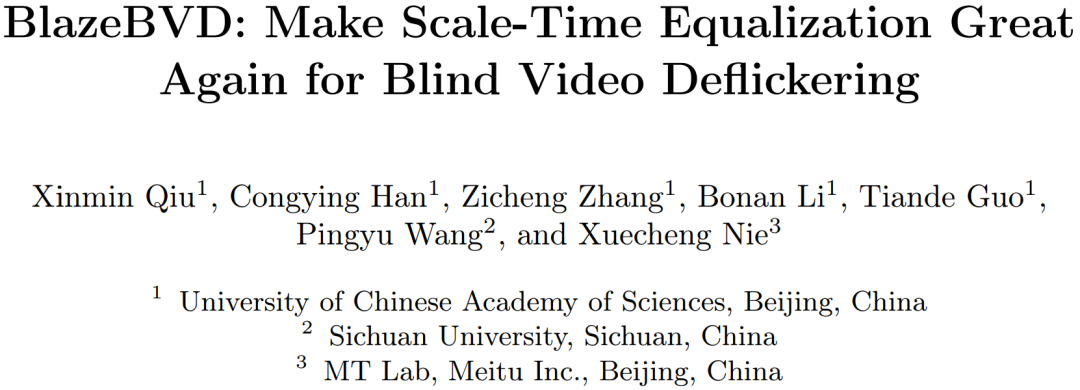
- Paper link: https://arxiv.org/pdf/2403.06243v1
BlazeBVD is aimed at video flickering scenarios. Video flickering can easily affect time consistency, and time consistency is high quality. A necessary condition for video output, even weak video flickering may seriously affect the viewing experience. The reason is generally caused by poor shooting environment and hardware limitations of the shooting equipment, and when image processing technology is applied to video frames, this problem is often further exacerbated. Furthermore, flicker artifacts and color distortion issues also frequently arise in recent video generation tasks, including those based on generative adversarial networks (GAN) and diffusion models (DM). Therefore, in various video processing scenarios, it is crucial to explore Blind Video Deflickering (BVD) to eliminate video flicker and maintain the integrity of video content. The BVD task is not affected by the cause and degree of video flicker, and has a wide range of application prospects. The current focus on such tasks mainly includes old movie restoration, high-speed camera shooting, color distortion processing, etc. and video flicker types. , tasks that have nothing to do with flicker degree, and tasks that only need to operate on a single flicker video without requiring additional guidance information such as video flicker type, reference video input, etc. In addition, BVD is now mainly focused on traditional filtering, forced temporal consistency, and atlas methods. Therefore, although deep learning methods have made significant progress in BVD tasks, they are greatly hindered at the application level due to the lack of prior knowledge. BVD still faces many challenges. BlazeBVD: Effectively improve the blind video de-flicker effectInspired by the classic flicker removal method scale-time equalization (STE), BlazeBVD introduces a histogram-assisted solution. Image histogram is defined as the distribution of pixel values. It is widely used in image processing to adjust the brightness or contrast of an image. Given an arbitrary video, STE can smooth the histogram by using Gaussian filtering and correct each image using histogram equalization. pixel values in the frame, thereby improving the visual stability of the video. Although STE is only effective for some minor flickers, it verifies:
- Histograms are much more compact than pixel values and can depict light and flicker information well.
- The video after histogram sequence smoothing has no obvious flicker visually.
Therefore, it is feasible to utilize cues from STE and histograms to improve the quality and speed of blind video de-flickering. BlazeBVD generates singular frame collections, filtered light maps, and exposure mask maps by smoothing these histograms to achieve fast and stable texture recovery in the presence of lighting fluctuations and over- or under-exposure. Compared with previous deep learning methods, BlazeBVD carefully uses histograms to reduce the learning complexity of BVD tasks for the first time, simplifying the complexity and resource consumption of learning video data. Its core is to use the flicker prior of STE, including for A filtered illumination map that guides the removal of global flicker, a singular frame set that identifies flicker frame indexes, and an exposure map that identifies regions that are locally affected by overexposure or darkness. At the same time, using flicker priors, BlazeBVD combines a global flicker removal module (GFRM) and a local flicker removal module (LFRM) to effectively correct the global illumination and local exposure texture of individual adjacent frames. . Additionally, to enhance inter-frame consistency, a lightweight timing network (TCM) is integrated, improving performance without consuming a lot of time. 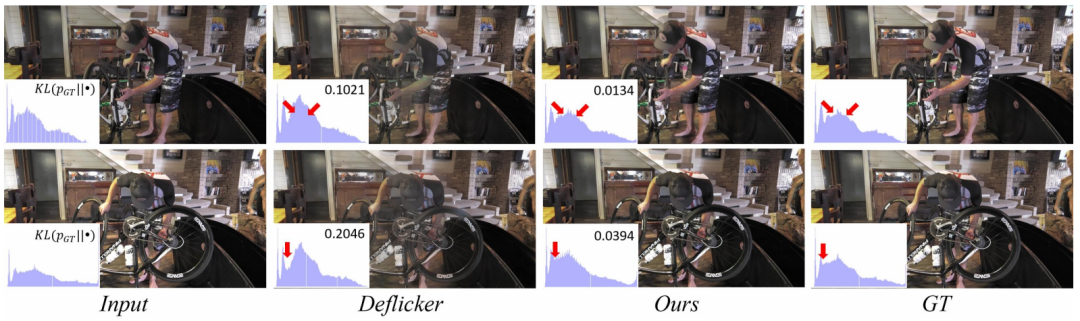
Figure 1: Comparison of results between the BlazeBVD method and existing methods on the blind video de-flicker task Specifically, BlazeBVD includes three stages:
- First, introduce STE The histogram sequence of the video frames in the illumination space is corrected, and the flicker prior including the singular frame set, the filtered illumination map and the exposure map are extracted.
- Secondly, since the filtered illumination maps have stable temporal performance, they will be used as cue conditions for the Global Flicker Removal Module (GFRM) containing a 2D network to guide the color correction of video frames. On the other hand, the Local Flicker Removal Module (LFRM) recovers overexposed or dark areas marked by local exposure maps based on optical flow information.
- Finally, a lightweight temporal network (TCM) is introduced to process all frames, in which an adaptive mask weighted loss is designed to improve video consistency.
Through comprehensive experiments on synthetic videos, real videos, and generated videos, we demonstrate the superior qualitative and quantitative results of BlazeBVD, achieving model inference speeds that are 10 times faster than state-of-the-art model inference speeds. 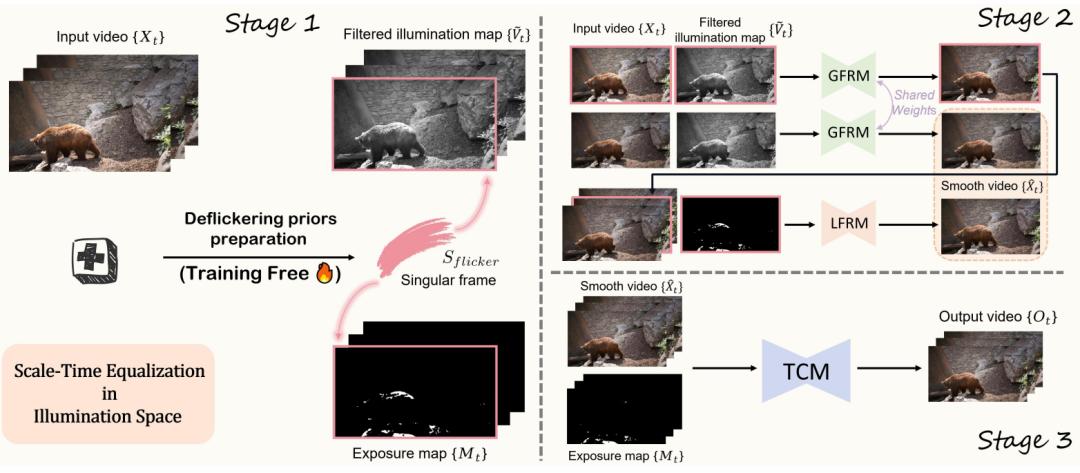
Figure 2: BlazeBVD’s training and inference process A large number of experiments have shown that BlazeBVD, a general method for blind video flashing tasks, is effective in synthesizing It is better than previous work on data sets and real data sets, and ablation experiments also verify the effectiveness of the module designed by BlazeBVD. 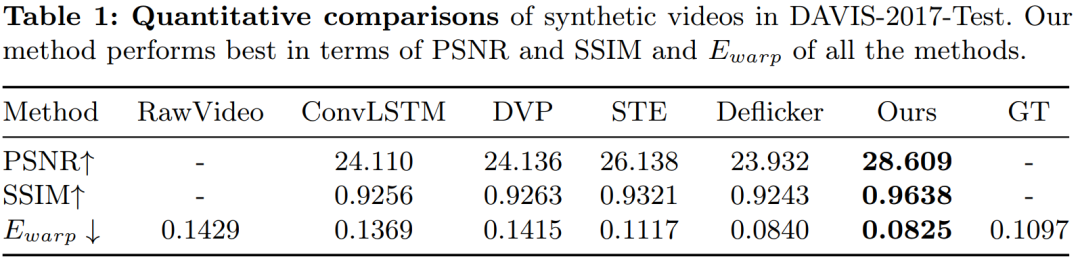
Figure 3: Visual comparison with baseline method
Figure 4: Ablation experiment 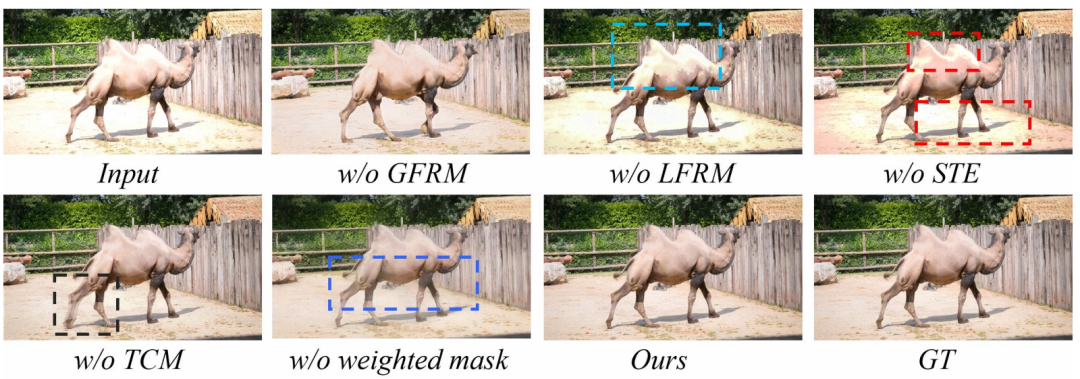
Using imaging technology to help productivity
This paper proposes BlazeBVD, a general method for blind video flicker tasks, using 2D networks to repair low-quality flickers affected by illumination changes or local exposure problems. video. Its core is to preprocess flicker priors within the STE filter in the lighting space; then use these priors, combined with the global flicker removal module (GFRM) and the local flicker removal module (LFRM), to correct global flicker and local exposure textures; Finally, a lightweight temporal network (TCM) is used to improve the coherence and inter-frame consistency of the video, and also achieves a 10x acceleration in model inference. As an explorer in the field of imaging and design in China, Meitu continues to launch convenient and efficient AI functions to bring innovative services and experiences to users. As the core R&D center, Meitu Imaging Research Institute (MT Lab) will continue to Iteratively upgrade AI capabilities to provide video creators with new video creation methods and open up a broader world.
Atas ialah kandungan terperinci ECCV 2024|BlazeBVD, kaedah umum untuk penyahkelipan video buta, ada di sini, dicadangkan bersama oleh Meitu dan Universiti Kebangsaan Sains dan Teknologi China. Untuk maklumat lanjut, sila ikut artikel berkaitan lain di laman web China PHP!
Kenyataan:Kandungan artikel ini disumbangkan secara sukarela oleh netizen, dan hak cipta adalah milik pengarang asal. Laman web ini tidak memikul tanggungjawab undang-undang yang sepadan. Jika anda menemui sebarang kandungan yang disyaki plagiarisme atau pelanggaran, sila hubungi admin@php.cn