Golang technology accelerates model training in machine learning
By taking advantage of Go's high-performance concurrency, machine learning model training can be accelerated: 1. Parallel data loading, making full use of Goroutine to load data; 2. Optimization algorithm, distributed computing through the channel mechanism; 3. Distributed computing, using native The network supports training on multiple machines.
Accelerate machine learning model training with Go
The Go language is known for its high performance and concurrency, which makes it the Ideal for accelerating machine learning model training. This article will introduce how to use Go to process data in parallel, optimize algorithms, and utilize distributed computing to greatly improve model training speed.
1. Parallel data loading
Loading and preprocessing data is the bottleneck in the machine learning training process. Go's Goroutines can easily parallelize this process, allowing data to be loaded from multiple sources simultaneously. The following code snippet demonstrates how to use Goroutine to load image data in parallel:
import "sync" type imageData struct { label int pixels []float32 } func main() { var data []imageData var wg sync.WaitGroup for i := 0; i < numImages; i++ { wg.Add(1) go func(i int) { data[i] = loadAndPreprocessImage(i) wg.Done() }(i) } wg.Wait() }
2. Optimization Algorithm
Go's unique channel mechanism can easily optimize the algorithm to run on multiple Distribute computation among Goroutines. The following code snippet shows how to use channels to parallelize gradient calculations:
import "sync" type gradients struct { weights []float32 biases []float32 } func main() { var gradientsCh = make(chan gradients, 10) var wg sync.WaitGroup for i := 0; i < numLayers; i++ { wg.Add(1) go func(i int) { gradientsCh <- computeGradients(i) wg.Done() }(i) } wg.Wait() }
3. Distributed Computing
For large data sets, distribute across multiple machines It is necessary to train the model in a formal way. The Go language's native networking support makes it easy to build distributed computing systems. The following code snippet demonstrates how to use gRPC to distribute model training across multiple nodes:
import "google.golang.org/grpc" type modelTrainRequest struct { inputData []float32 labels []int } func main() { conn, err := grpc.Dial("grpc-server:8080", grpc.WithInsecure()) if err != nil { // Handle error } defer conn.Close() client := modelTrainServiceClient{conn} resp, err := client.TrainModel(ctx, &modelTrainRequest{}) if err != nil { // Handle error } }
Practical Case
Using Go-optimized machine learning model training has been widely used Used in various practical projects. For example:
- Large-scale image classification
- Natural language processing
- Recommendation system
Conclusion
By using Go's parallel processing, optimization algorithms, and distributed computing capabilities, machine learning model training can be greatly accelerated. The techniques and code snippets presented in this article provide a starting point for applying these concepts in practice.
The above is the detailed content of Golang technology accelerates model training in machine learning. For more information, please follow other related articles on the PHP Chinese website!
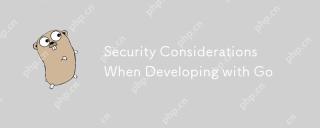
Gooffersrobustfeaturesforsecurecoding,butdevelopersmustimplementsecuritybestpracticeseffectively.1)UseGo'scryptopackageforsecuredatahandling.2)Manageconcurrencywithsynchronizationprimitivestopreventraceconditions.3)SanitizeexternalinputstoavoidSQLinj
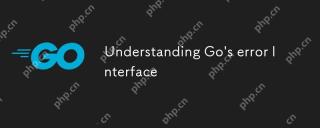
Go's error interface is defined as typeerrorinterface{Error()string}, allowing any type that implements the Error() method to be considered an error. The steps for use are as follows: 1. Basically check and log errors, such as iferr!=nil{log.Printf("Anerroroccurred:%v",err)return}. 2. Create a custom error type to provide more information, such as typeMyErrorstruct{MsgstringDetailstring}. 3. Use error wrappers (since Go1.13) to add context without losing the original error message,
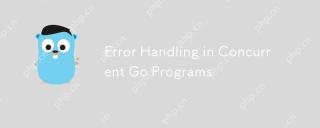
ToeffectivelyhandleerrorsinconcurrentGoprograms,usechannelstocommunicateerrors,implementerrorwatchers,considertimeouts,usebufferedchannels,andprovideclearerrormessages.1)Usechannelstopasserrorsfromgoroutinestothemainfunction.2)Implementanerrorwatcher
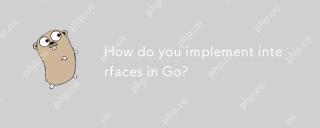
In Go language, the implementation of the interface is performed implicitly. 1) Implicit implementation: As long as the type contains all methods defined by the interface, the interface will be automatically satisfied. 2) Empty interface: All types of interface{} types are implemented, and moderate use can avoid type safety problems. 3) Interface isolation: Design a small but focused interface to improve the maintainability and reusability of the code. 4) Test: The interface helps to unit test by mocking dependencies. 5) Error handling: The error can be handled uniformly through the interface.
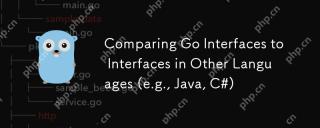
Go'sinterfacesareimplicitlyimplemented,unlikeJavaandC#whichrequireexplicitimplementation.1)InGo,anytypewiththerequiredmethodsautomaticallyimplementsaninterface,promotingsimplicityandflexibility.2)JavaandC#demandexplicitinterfacedeclarations,offeringc
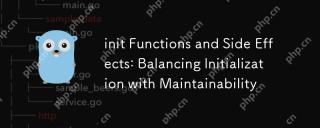
Toensureinitfunctionsareeffectiveandmaintainable:1)Minimizesideeffectsbyreturningvaluesinsteadofmodifyingglobalstate,2)Ensureidempotencytohandlemultiplecallssafely,and3)Breakdowncomplexinitializationintosmaller,focusedfunctionstoenhancemodularityandm
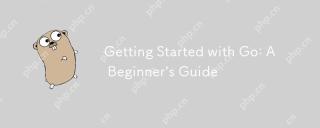
Goisidealforbeginnersandsuitableforcloudandnetworkservicesduetoitssimplicity,efficiency,andconcurrencyfeatures.1)InstallGofromtheofficialwebsiteandverifywith'goversion'.2)Createandrunyourfirstprogramwith'gorunhello.go'.3)Exploreconcurrencyusinggorout
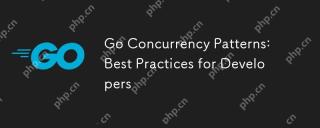
Developers should follow the following best practices: 1. Carefully manage goroutines to prevent resource leakage; 2. Use channels for synchronization, but avoid overuse; 3. Explicitly handle errors in concurrent programs; 4. Understand GOMAXPROCS to optimize performance. These practices are crucial for efficient and robust software development because they ensure effective management of resources, proper synchronization implementation, proper error handling, and performance optimization, thereby improving software efficiency and maintainability.


Hot AI Tools

Undresser.AI Undress
AI-powered app for creating realistic nude photos

AI Clothes Remover
Online AI tool for removing clothes from photos.

Undress AI Tool
Undress images for free

Clothoff.io
AI clothes remover

Video Face Swap
Swap faces in any video effortlessly with our completely free AI face swap tool!

Hot Article

Hot Tools

Atom editor mac version download
The most popular open source editor

SecLists
SecLists is the ultimate security tester's companion. It is a collection of various types of lists that are frequently used during security assessments, all in one place. SecLists helps make security testing more efficient and productive by conveniently providing all the lists a security tester might need. List types include usernames, passwords, URLs, fuzzing payloads, sensitive data patterns, web shells, and more. The tester can simply pull this repository onto a new test machine and he will have access to every type of list he needs.
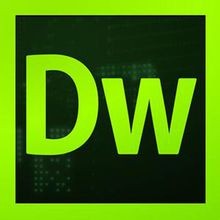
Dreamweaver CS6
Visual web development tools

SublimeText3 Chinese version
Chinese version, very easy to use

DVWA
Damn Vulnerable Web App (DVWA) is a PHP/MySQL web application that is very vulnerable. Its main goals are to be an aid for security professionals to test their skills and tools in a legal environment, to help web developers better understand the process of securing web applications, and to help teachers/students teach/learn in a classroom environment Web application security. The goal of DVWA is to practice some of the most common web vulnerabilities through a simple and straightforward interface, with varying degrees of difficulty. Please note that this software
