The AIxiv column is a column where this site publishes academic and technical content. In the past few years, the AIxiv column of this site has received more than 2,000 reports, covering top laboratories from major universities and companies around the world, effectively promoting academic exchanges and dissemination. If you have excellent work that you want to share, please feel free to contribute or contact us for reporting. Submission email: liyazhou@jiqizhixin.com; zhaoyunfeng@jiqizhixin.com
##Multimodal fusion is multimodal intelligence One of the basic tasks in . The motivation of multi-modal fusion is to jointly utilize effective information from different modalities to improve the accuracy and stability of downstream tasks. Traditional multi-modal fusion methods often rely on high-quality data and are difficult to adapt to the complex and low-quality multi-modal data in real applications.
Low-quality multimodal jointly released by Tianjin University, Renmin University of China, Singapore Agency for Science, Technology and Research, Sichuan University, Xi'an University of Electronic Science and Technology and Harbin Institute of Technology (Shenzhen) Data fusion review "Multimodal Fusion on Low-quality Data: A Comprehensive Survey" introduces the fusion challenges of multimodal data from a unified perspective, and focuses on the existing fusion methods of low-quality multimodal data and potential development directions in this field. Ordered.
arXiv link:
http://arxiv.org/abs/2404.18947
awesome-list link:
https://github.com/QingyangZhang/awesome-low-quality-multimodal-learning
Traditional multimodal fusion model#Humans perceive the world by fusing information from multiple modalities.
Humans have the ability to process these low-quality multi-modal data signals and perceive the environment even when the signals of some modalities are unreliable.
Although multimodal learning has made great progress, multimodal machine learning models still lack the ability to effectively fuse low-quality multimodal data in the real world. In practical experience, the performance of traditional multi-modal fusion models will decline significantly in the following scenarios:
(1)
Noisy multi-modal data : Some features of some modes are disturbed by noise and lose their original information. In the real world, unknown environmental factors, sensor failures, and signal loss during transmission may introduce noise interference, thereby damaging the reliability of the multi-modal fusion model. (2)
Missing multimodal data: Due to various practical factors, some modalities of the actual collected multimodal data samples There may be something missing. For example, in the medical field, the multimodal data composed of patients' various physiological examination results may be seriously missing, and some patients may have never had a certain examination. (3)
Imbalanced multi-modal data: Due to the inconsistency in the heterogeneous encoding properties and information quality differences between modalities, This leads to the emergence of imbalanced learning problems between modalities. During the multi-modal fusion process, the model may rely too much on certain modalities and ignore the potentially effective information contained in other modalities. (4)
Dynamic low-quality multi-modal data: Due to the complexity and change of the application environment, different samples, different time and space, the modal quality It has dynamic changing characteristics. The occurrence of low-quality modal data is often difficult to predict in advance, which brings challenges to multi-modal fusion. In order to fully characterize the nature and processing methods of low-quality multi-modal data, this article summarizes the current machine learning methods in the field of low-quality multi-modal fusion. The development process of this field is systematically reviewed, and issues that require further research are further prospected.
Figure 1. Low -quality multi -modal data classification schematic diagram, yellow and blue represent two modes, the deeper the color represents the higher the quality #Denoising method in multi-modal fusionNoise is one of the most common causes of multimodal data quality degradation.
This article mainly focuses on two types of noise:
(1)Mode-related multi-modal noise. This type of noise may be caused by factors such as sensor errors (such as instrument errors in medical diagnosis) and environmental factors (such as rain and fog in autonomous driving). The noise is limited to certain feature levels within a specific mode.
(2) Cross-modal noise at the semantic level. This type of noise is caused by the misalignment of high-level semantics between modalities, and is more difficult to process than multi-modal noise at the feature layer. Fortunately, due to the complementarity between multi-modal data modes and the redundancy of information, combining information from multiple modalities for denoising has proven to be an effective strategy in the multi-modal fusion process. .
Feature-level multi-mode State denoising methods are highly dependent on the specific modalities involved in the actual task.
This article mainly takes the multi-modal image fusion task as an example to illustrate. In multi-modal image fusion, the mainstream denoising methods include weighted fusion and joint variation.
Considering that feature noise is random and real data obeys a specific distribution, the influence of noise is eliminated through weighted summation;
is an expansion of traditional single-modal image variation denoising, which can transform the denoising process into an optimization problem. solution process, and utilizes complementary information from multiple modalities to improve the denoising effect. Semantic-level cross-modal noise results from weakly aligned or misaligned multimodal sample pairs.
For example, in the multi-modal target detection task of joint RGB and thermal images, due to differences in sensors, although the same target is present in both modalities appears, but its precise position and attitude may be slightly different in different modalities (weak alignment), which brings challenges to accurately estimate position information.
In the content understanding task of social media, the semantic information contained in the image and text modalities of a sample (such as a Weibo) may be very different or even completely different. Irrelevant (completely misaligned), which further brings greater challenges to multi-modal fusion. Ways to deal with cross-modal semantic noise include rule filtering, model filtering, noise-robust model regularization and other methods.
Although the processing of data noise has long been used in classic machine learning The task has been extensively studied, but in multi-modal scenarios, how to jointly utilize the complementarity and consistency between modalities to weaken the impact of noise is still an urgent research problem to be solved.
In addition, unlike traditional feature-level denoising, how to solve semantic-level noise during the pre-training and inference process of multi-modal large models is interesting and extremely Challenging questions.
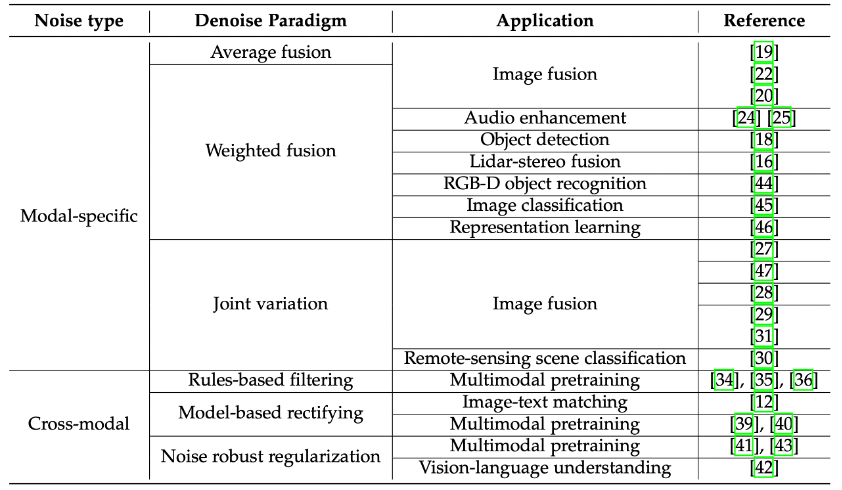
### Table 1. Classification of multi-modal fusion methods for noiseMissing many Modal data fusion methodIn real scenarios The collected multimodal data is often incomplete. Due to various factors such as damage to the storage device and unreliable data transmission process, multimodal data often inevitably loses part of the modal information. For example: in the recommendation system, the user's browsing history and credit rating constitute multi-modal data. However, due to permissions and privacy issues, it is often impossible to fully collect To build a multi-modal learning system based on user information from all modalities. In medical diagnosis, due to limited equipment in some hospitals and high cost of specific examinations, the multi-modal diagnostic data of different patients are often highly incomplete. . According to "Whether it is necessary to explicitly correct missing multi-mode Based on the classification principle of "completing modal data", missing multi-modal data fusion methods can be divided into: (1) Multi-modal fusion method based on completion Completion-based multi-modal fusion methods include model-independent completion methods: for example, completion methods that directly fill missing modes with 0 values or the mean value of residual modes ; Completion methods based on graphs or kernels: This type of method does not directly learn how to complete the original multi-modal data, but constructs a graph or kernel for each modality. Kernel, and then learn the similarity or correlation information between sample pairs, and then complete the missing data; Complete directly at the original feature level: some methods Use generative models, such as Generative Adversarial Network GAN and its variants, to directly complete the missing features. (2) Multi-modal fusion method without completion. Different from completion-based methods, completion-free methods focus on how to use the useful information contained in the non-missing modalities to fuse the best possible representation. This type of method often adds constraints to the unified representation expected to be learned, so that this representation can reflect the complete information of the observable modal data, so as to bypass the completion process for multi-modal fusion.
Although many methods have been proposed at home and abroad to solve many incomplete problems in classic machine learning tasks such as clustering and classification. modal data fusion problem, but there are still some deeper challenges.
For example: Quality assessment of completion data in missing modal completion schemes is often overlooked. In addition, the strategy of using a priori missing data location information to shield the missing modality itself is difficult to make up for the information gap and information imbalance caused by the missing modality. Table 2. Classification of fusion methods for missing multi-modal dataBalance Multi-modal fusion methodIn multi-modal fusion In modal learning, joint training is usually used to integrate data from different modalities to improve the overall performance and generalization performance of the model. However, this type of widely adopted joint training paradigm that uses a unified learning objective ignores the heterogeneity of data from different modalities. The heterogeneity of different modes in terms of data sources and forms
makes them have different characteristics in terms of convergence speed, etc. This makes it difficult for all modalities to be processed and learned well at the same time, which brings difficulties to multi-modal joint learning;
On the other hand, this difference also reflects On the quality of unimodal data
. Although all modalities describe the same concept, they vary in the amount of information related to the target event or target object. Deep neural networks based on the maximum likelihood learning objective have greedy learning characteristics, resulting in multi-modal models that often rely on high-quality modalities with high discriminative information and are easier to learn, while insufficiently modeling other modal information.
In order to address these challenges and improve the learning quality of multi-modal models, related research on balanced multi-modal learning
has received widespread attention recently. Method classification:
According to different balance angles, related methods can be divided into For methods based on characteristic differences
and methods based on quality differences.
(1) The widely used multi-modal joint training framework often ignores the inherent differences in learning properties of single-modal data
, which may have a negative impact on Negatively affects model performance. The method based on characteristic differences starts from the differences in learning characteristics of each modality and tries to solve this problem in terms of learning goals, optimization, and architecture.
(2) Recent research further found that multi-modal models often heavily rely on certain high-quality information modalities
while ignoring others modalities, resulting in insufficient learning of all modalities. Methods based on quality differences start from this perspective and try to solve this problem and promote the balanced utilization of different modalities in multi-modal models from the perspectives of learning objectives, optimization methods, model architecture and data enhancement. Table 3. Classification of balanced multi-modal data fusion methods The balanced multi-modal learning method mainly targets the differences in learning characteristics or data quality between different modalities caused by the heterogeneity of multi-modal data. These methods propose solutions from different perspectives such as learning objectives, optimization methods, model architecture, and data enhancement. Balanced multimodal learning is currently a booming field, and there are many theoretical and application directions that have not been fully explored. For example, current methods are mainly limited to typical multi-modal tasks, which are mostly discriminative tasks and a few generative tasks. In addition, multi-modal large models also need to combine modal data with different qualities. There is also this objective imbalance problem. Accordingly, it is expected that in Extend existing research or design new solutions in multimodal large-model scenarios. Dynamic multi-modal fusion methodDynamic multimodal data refers to the fact that the quality of the modality changes dynamically with different input samples and scenarios. For example, in autonomous driving scenarios, the system obtains road surface and target information through RGB and infrared sensors. Under good lighting conditions, the RGB camera can better support the decision-making of the intelligent system because it can capture the rich texture and color information of the target;# However, at night when there is insufficient light, the perception information provided by the infrared sensor is more reliable. How to enable the model to automatically sense changes in the quality of different modalities, so as to perform accurate and stable fusion, is the core task of the dynamic multi-modal fusion method. Dynamic multi-modal fusion methods can be roughly divided into three categories:
(1) Heuristic dynamic fusion method:
#The heuristic dynamic fusion method relies on the algorithm designer’s understanding of the multi-modal model application scenarios, generally through This is achieved by introducing a dynamic fusion mechanism
For example, in the multi-modal target detection task of RGB/thermal signal collaboration, researchers heuristically designed an illumination perception module to dynamically evaluate the illumination of the input image situation, and dynamically adjust the fusion weight of RGB and thermal modes based on the light intensity to adapt to the environment. When the brightness is high, the RGB mode is mainly relied on for decision-making, and vice versa, the thermal mode is mainly relied on for decision-making.
(2) Dynamic fusion method based on attention mechanism:
Dynamic fusion method based on attention mechanism Mainly focus on presentation layer fusion
. The attention mechanism itself has dynamic characteristics, so it can be naturally used in multi-modal dynamic fusion tasks. Various mechanisms such as Self-attention, Spatial attention, Channel attention and Transformer are widely used in the construction of multi-modal fusion models. Such methods automatically learn how to perform dynamic fusion, driven by task goals. The fusion based on the attention mechanism can adapt to dynamic low-quality multi-modal data to a certain extent in the absence of explicit or heuristic guidance.
(3) Dynamic fusion method of uncertainty perception:
Dynamic fusion method of uncertainty perception Often have clearer and explainable fusion mechanisms
. Different from complex fusion modes based on attention mechanisms, uncertainty-aware dynamic fusion methods rely on uncertainty estimates of modalities (such as evidence, energy, entropy, etc.) to adapt to low-quality multi-modal data. #Specifically, uncertainty perception can be used to characterize the quality changes of each modality of the input data. When the quality of a certain modality of the input sample becomes low, the uncertainty of the model's decision-making based on that modality becomes higher, providing clear guidance for subsequent fusion mechanism design. In addition, compared to heuristics and attention mechanisms, uncertainty-aware dynamic fusion methods can provide good theoretical guarantees.Although in traditional multi-modal fusion tasks, The superiority of uncertainty-aware dynamic fusion methods has been proven experimentally and theoretically. However, in SOTA's multi-modal models (not limited to fusion models, such as CLIP/BLIP, etc.), the idea of dynamics also has Greater potential for exploration and application. In addition, dynamic fusion mechanisms with theoretical guarantees are often limited to the decision-making level. How to make them work at the representation level is also worth thinking about and exploring. The above is the detailed content of Low-quality multi-modal data fusion, multiple institutions jointly published a review paper. For more information, please follow other related articles on the PHP Chinese website!