


How to create efficient and scalable machine learning algorithms using Java functions?
Using Java functional programming and scalability techniques, you can create efficient and scalable machine learning algorithms with the following benefits: immutability, simplified inference, and improved concurrency. Pure functions enhance testability and predictability. Higher-order functions that allow the creation of flexible and reusable components. Parallelization, using multi-threading or distributed computing parallelization algorithms. Algorithm optimization, using optimization techniques to reduce computational costs. Data partitioning facilitates processing of data sets in distributed systems.
Use Java functions to create efficient and scalable machine learning algorithms
Introduction
In In the field of machine learning, efficient and scalable algorithms are crucial. Java functional programming provides powerful tools to help create such algorithms. This article explores how to use Java functions to create efficient and scalable machine learning algorithms.
Advantages of Functional Programming
Functional programming provides the following advantages that greatly benefit machine learning algorithms:
- Immutability: Functional programming operations do not modify data, simplifying reasoning and improving concurrency.
- Pure function: A function produces output based only on its input, without side effects, enhancing testability and predictability.
- Higher-order functions: Functions can be used as parameters and return values of other functions, allowing the creation of flexible and reusable components.
Practical case: Using Java functions to build a linear regression model
The following are the steps to use Java functions to build a simple linear regression model:
-
Define data type: Define a
Point
class to represent data points. -
Create training data: Generate some training data, each data point contains a feature (
x
) and a label (y
). -
Define the regression function: Use Java function to implement the linear regression function:
double predict(double x, double slope, double intercept) { return slope * x + intercept; }
-
Train the model: Use the least squares method Train the model and determine the optimal slope (
slope
) and intercept (intercept
) values. - Evaluate the model: Evaluate the performance of the model using root mean square error (RMSE) or other metrics.
Scalability Technology
In addition to functional programming, there are some techniques that can improve the scalability of machine learning algorithms:
- Parallelization: Use multi-threading or distributed computing to parallelize algorithms.
- Algorithm optimization: Use optimization techniques such as recursive and iterative optimization to reduce the computational cost of the algorithm.
- Data Partitioning: Divide the data set into smaller partitions for easy processing in a distributed system.
By combining functional programming with scalability techniques, you can create efficient and scalable machine learning algorithms for handling large data sets and complex problems.
The above is the detailed content of How to create efficient and scalable machine learning algorithms using Java functions?. For more information, please follow other related articles on the PHP Chinese website!
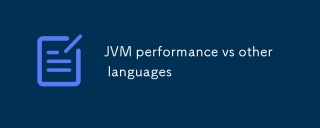
JVM'sperformanceiscompetitivewithotherruntimes,offeringabalanceofspeed,safety,andproductivity.1)JVMusesJITcompilationfordynamicoptimizations.2)C offersnativeperformancebutlacksJVM'ssafetyfeatures.3)Pythonisslowerbuteasiertouse.4)JavaScript'sJITisles

JavaachievesplatformindependencethroughtheJavaVirtualMachine(JVM),allowingcodetorunonanyplatformwithaJVM.1)Codeiscompiledintobytecode,notmachine-specificcode.2)BytecodeisinterpretedbytheJVM,enablingcross-platformexecution.3)Developersshouldtestacross
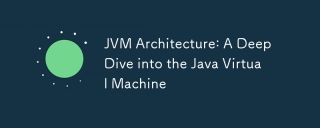
TheJVMisanabstractcomputingmachinecrucialforrunningJavaprogramsduetoitsplatform-independentarchitecture.Itincludes:1)ClassLoaderforloadingclasses,2)RuntimeDataAreafordatastorage,3)ExecutionEnginewithInterpreter,JITCompiler,andGarbageCollectorforbytec
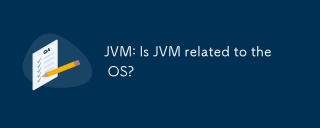
JVMhasacloserelationshipwiththeOSasittranslatesJavabytecodeintomachine-specificinstructions,managesmemory,andhandlesgarbagecollection.ThisrelationshipallowsJavatorunonvariousOSenvironments,butitalsopresentschallengeslikedifferentJVMbehaviorsandOS-spe
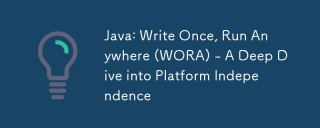
Java implementation "write once, run everywhere" is compiled into bytecode and run on a Java virtual machine (JVM). 1) Write Java code and compile it into bytecode. 2) Bytecode runs on any platform with JVM installed. 3) Use Java native interface (JNI) to handle platform-specific functions. Despite challenges such as JVM consistency and the use of platform-specific libraries, WORA greatly improves development efficiency and deployment flexibility.
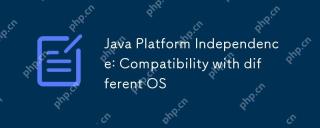
JavaachievesplatformindependencethroughtheJavaVirtualMachine(JVM),allowingcodetorunondifferentoperatingsystemswithoutmodification.TheJVMcompilesJavacodeintoplatform-independentbytecode,whichittheninterpretsandexecutesonthespecificOS,abstractingawayOS
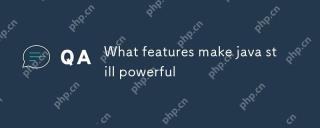
Javaispowerfulduetoitsplatformindependence,object-orientednature,richstandardlibrary,performancecapabilities,andstrongsecurityfeatures.1)PlatformindependenceallowsapplicationstorunonanydevicesupportingJava.2)Object-orientedprogrammingpromotesmodulara
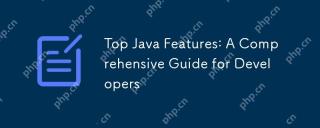
The top Java functions include: 1) object-oriented programming, supporting polymorphism, improving code flexibility and maintainability; 2) exception handling mechanism, improving code robustness through try-catch-finally blocks; 3) garbage collection, simplifying memory management; 4) generics, enhancing type safety; 5) ambda expressions and functional programming to make the code more concise and expressive; 6) rich standard libraries, providing optimized data structures and algorithms.


Hot AI Tools

Undresser.AI Undress
AI-powered app for creating realistic nude photos

AI Clothes Remover
Online AI tool for removing clothes from photos.

Undress AI Tool
Undress images for free

Clothoff.io
AI clothes remover

Video Face Swap
Swap faces in any video effortlessly with our completely free AI face swap tool!

Hot Article

Hot Tools
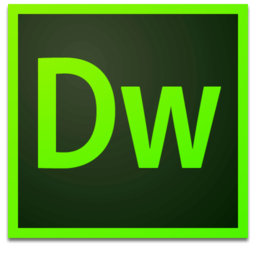
Dreamweaver Mac version
Visual web development tools
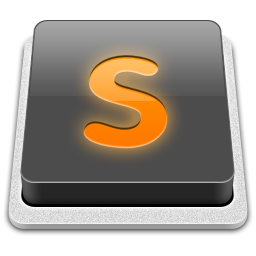
SublimeText3 Mac version
God-level code editing software (SublimeText3)
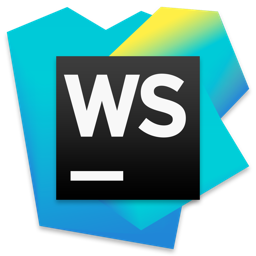
WebStorm Mac version
Useful JavaScript development tools

Atom editor mac version download
The most popular open source editor

DVWA
Damn Vulnerable Web App (DVWA) is a PHP/MySQL web application that is very vulnerable. Its main goals are to be an aid for security professionals to test their skills and tools in a legal environment, to help web developers better understand the process of securing web applications, and to help teachers/students teach/learn in a classroom environment Web application security. The goal of DVWA is to practice some of the most common web vulnerabilities through a simple and straightforward interface, with varying degrees of difficulty. Please note that this software
