


Recently, researchers from Monash University in Australia, Ant Group, IBM Research and other institutions have explored the application of model reprogramming (model reprogramming) on large language models (LLMs), and proposed a new perspective: Efficient reprogramming of large language models for general time series forecasting systems, the Time-LLM framework. This framework can achieve high-precision and efficient predictions without modifying the language model, and can surpass traditional time series models in multiple data sets and prediction tasks, allowing LLMs to demonstrate outstanding performance when processing cross-modal time series data. Like an elephant dancing.
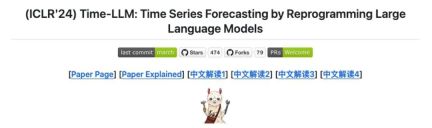
Recently, with the development of large language models in the field of general intelligence, the new direction of "large model time series/time data" has shown many related developments. Current LLMs have the potential to revolutionize time series/temporal data mining methods, thereby promoting efficient decision-making in classic complex systems such as cities, energy, transportation, health, etc., and moving towards more universal intelligent forms of time/space analysis.
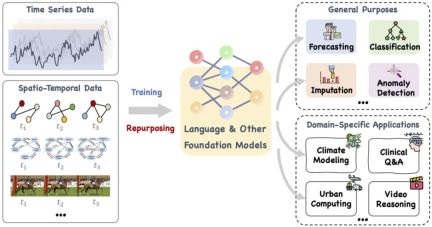
This paper proposes a large base model, such as language and other related models, that can be trained and also cleverly retuned Purpose to process time series and time-spatial data for a range of general-purpose tasks and domain-specific applications. Reference: https://arxiv.org/pdf/2310.10196.pdf.
Recent research has expanded large-scale language models from processing natural language to time series and spatio-temporal tasks. This new research direction, namely "large model time series / spatiotemporal data", has produced many related developments, such as LLMTime, which directly utilizes LLMs for zero-shot time series predictive inference. Although LLMs have powerful learning and expression capabilities and can effectively capture complex patterns and long-term dependencies in text sequence data, as a "black box" focused on processing natural language, the application of LLMs in time series and spatiotemporal tasks is still face the challenge. Compared with traditional time series models such as TimesNet, TimeMixer, etc., LLMs are comparable to "elephant" due to their huge parameters and scale.
What you are asking is how to "tame" such large language models (LLMs) trained in the field of natural language so that they can process numerical sequence data across text patterns and perform in time series and spatiotemporal tasks. Developing powerful reasoning and prediction capabilities has become a key focus of current research. To this end, more in-depth theoretical analysis is needed to explore potential pattern similarities between linguistic and temporal data and effectively apply them to specific time series and spatiotemporal tasks.
LLM Reprogramming model (LLM Reprogramming) is a general time series prediction technology. It proposes two key technologies, namely (1) temporal input reprogramming and (2) prompt pre-programming, which converts the temporal prediction task into a "language" task that can be effectively solved by LLMs, successfully activating large language models to achieve high performance. Ability to perform precise timing reasoning.
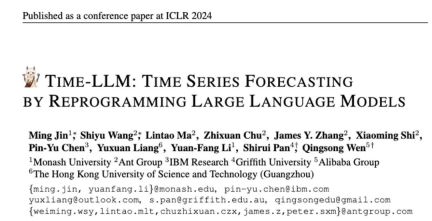
Paper address: https://openreview.net/pdf?id=Unb5CVPtae
Paper code: https://github.com/KimMeen/ Time-LLM
1. Problem background
Time series data is widely stored in reality, in which time series prediction is of great significance in many real-world dynamic systems , and has also been extensively studied. Unlike natural language processing (NLP) and computer vision (CV), where a single large model can handle multiple tasks, time series prediction models often need to be specially designed to meet the needs of different tasks and application scenarios. Recent research has shown that large language models (LLMs) are also reliable when processing complex temporal sequences. It is still a challenge to utilize the reasoning capabilities of large language models themselves to handle temporal analysis tasks.
2. Paper Overview
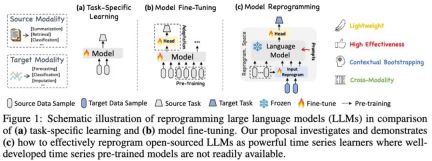
In this work, the author proposes Time-LLM, which is a general The language model reprogramming (LLM Reprogramming) framework can easily use LLM for general time series prediction without any training on the large language model itself. Time-LLM first uses text prototypes (Text Prototypes) to reprogram the input time series data, and uses natural language representation to represent the semantic information of the time series data, thereby aligning two different data modalities, so that the large language model does not require any modification. You can understand the information behind another data modality.
In order to further enhance LLM's understanding of input time series data and corresponding tasks, the author proposed the prompt-as-prefix (PaP) paradigm. By adding additional contextual prompts and task instructions before the time series data representation, Fully activate LLM's processing capabilities on timing tasks. In this work, the author conducted sufficient experiments on mainstream time series benchmark data sets, and the results showed that Time-LLM can surpass the traditional time series model in most cases and achieve better performance in few-shot and zero-shot samples. The sample (zero-shot) learning task has been greatly improved.
The main contributions in this work can be summarized as follows:
1. This work proposes a new concept of reprogramming large language models for timing analysis without having to do any modifications to the backbone language model. Any modifications. The authors show that time series prediction can be considered as another "linguistic" task that can be effectively solved by off-the-shelf LLMs.
2. This work proposes a general language model reprogramming framework, namely Time-LLM, which consists of reprogramming input temporal data into a more natural text prototype representation, and through declarative prompts such as domain expert knowledge and task description) to enhance the input context to guide LLM for effective cross-domain reasoning. This technology provides a solid foundation for the development of multimodal timing basic models.
3. Time-LLM consistently outperforms the best existing model performance in mainstream prediction tasks, especially in few-sample and zero-sample scenarios. Furthermore, Time-LLM is able to achieve higher performance while maintaining excellent model reprogramming efficiency. Dramatically unlock the untapped potential of LLM for time series and other sequential data.
3. Model framework
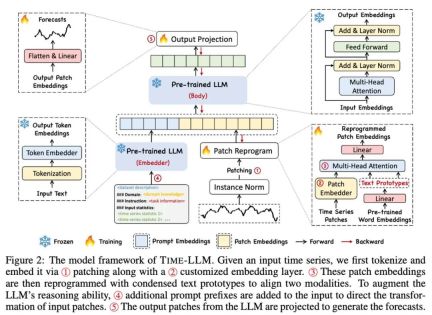
#As shown in ① and ② in the model framework diagram above, the input time series data is first refined through RevIN. The unification operation is then divided into different patches and mapped to the latent space.
There are significant differences in expression methods between time series data and text data, and they belong to different modalities. Time series can neither be directly edited nor described losslessly in natural language, which poses a significant challenge to directly prompting LLM to understand time series. Therefore, we need to align temporal input features to the natural language text domain.
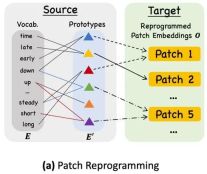
A common method to align different modalities is cross-attention. As shown in ③ in the model framework diagram, you only need to make an embedding and timing input feature of all words. cross-attention (where the time series input feature is Query, and the embedding of all words is Key and Value). However, the inherent vocabulary of LLM is very large, so it cannot effectively directly align temporal features to all words, and not all words have aligned semantic relationships with time series. In order to solve this problem, this work performs a linear combination of vocabulary to obtain text prototypes. The number of text prototypes is much smaller than the original vocabulary. The combination can be used to represent the changing characteristics of time series data, such as "a brief rise or a slow decline." ",As shown in FIG.
In order to fully activate the ability of LLM on specified timing tasks, this work proposes a prompt prefixing paradigm, which is a simple and effective method, as shown in ④ in the model framework diagram. Recent advances have shown that other data patterns such as images can be seamlessly integrated into the prefix of cues, allowing efficient inference based on these inputs. Inspired by these findings, the authors, in order to make their method directly applicable to real-world time series, pose an alternative question: Can hints serve as prefix information to enrich the input context and guide the transformation of reprogrammed time series patches? This concept is called Prompt-as-Prefix (PaP), and furthermore, the authors observed that it significantly improves the adaptability of LLM to downstream tasks while complementing patch reprogramming. In layman's terms, it means feeding some prior information of the time series data set in the form of natural language as a prefix prompt, and splicing it with the aligned time series features to LLM. Can it improve the prediction effect?
The picture above shows two prompt methods. In Patch-as-Prefix, a language model is prompted to predict subsequent values in a time series, expressed in natural language. This approach encounters some constraints: (1) Language models often exhibit low sensitivity when processing high-precision numbers without the assistance of external tools, which brings significant challenges to accurate processing of long-term prediction tasks; (2) Complex customized post-processing is required for different language models because they are pre-trained on different corpora and may employ different word segmentation types when generating high-precision numbers. This results in predictions being represented in different natural language formats, such as [‘0’, ‘.’, ‘6’, ‘1’] and [‘0’, ‘.’, ‘61’], which represents 0.61.
In practice, the authors identified three key components for building effective prompts: (1) dataset context; (2) task instructions to adapt LLM to different downstream tasks; (3) statistical description, For example, trends, time delays, etc. allow LLM to better understand the characteristics of time series data. The image below gives an example of a prompt.
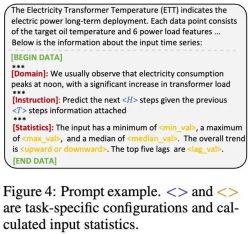
#4. Experimental results
We conducted comprehensive tests on 8 classic public data sets for long-range prediction. As shown in the table below, Time-LLM significantly exceeds the previous best results in the field in the benchmark comparison. In addition, compared with GPT4TS that directly uses GPT-2, Time-LLM adopts the reprogramming idea and Prompt-as-Prefix. There is also a significant improvement, indicating the effectiveness of this method.
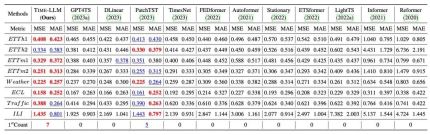
In addition, we evaluate the zero-shot zero-shot learning ability of reprogrammed LLM within the framework of cross-domain adaptation. Thanks to the ability of reprogramming, we fully activate It improves the prediction ability of LLM in cross-domain scenarios. As shown in the table below, Time-LLM also shows extraordinary prediction effects in zero-shot scenarios.
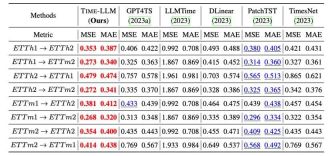
5. Summary
The rapid development of large language models (LLMs) has greatly promoted the application of artificial intelligence in cross-modal scenarios. progress and promote their wide application in many fields. However, the large parameter scale of LLMs and their design mainly for natural language processing (NLP) scenarios bring many challenges to their cross-modal and cross-domain applications. In view of this, we propose a new idea for reprogramming large models, aiming to achieve cross-modal interaction between text and sequence data, and widely apply this method to processing large-scale time series and spatiotemporal data. In this way, we hope to make LLMs like flexibly dancing elephants, able to demonstrate their powerful capabilities in a wider range of application scenarios.
Interested friends are welcome to read the paper (https://arxiv.org/abs/2310.01728) or visit the project page (https://github.com/KimMeen/Time-LLM) to learn more.
This project has received full support from NextEvo, the AI innovation R&D department of Ant Group’s Intelligent Engine Division, especially thanks to the close collaboration between the language and machine intelligence team and the optimization intelligence team. Under the leadership and guidance of Zhou Jun, Vice President of the Intelligent Engine Division, and Lu Xingyu, Head of the Optimization Intelligence Team, we worked together to successfully complete this important achievement.
The above is the detailed content of Who says elephants can't dance! Reprogramming large language models to achieve timing prediction of cross-modal interactions | ICLR 2024. For more information, please follow other related articles on the PHP Chinese website!
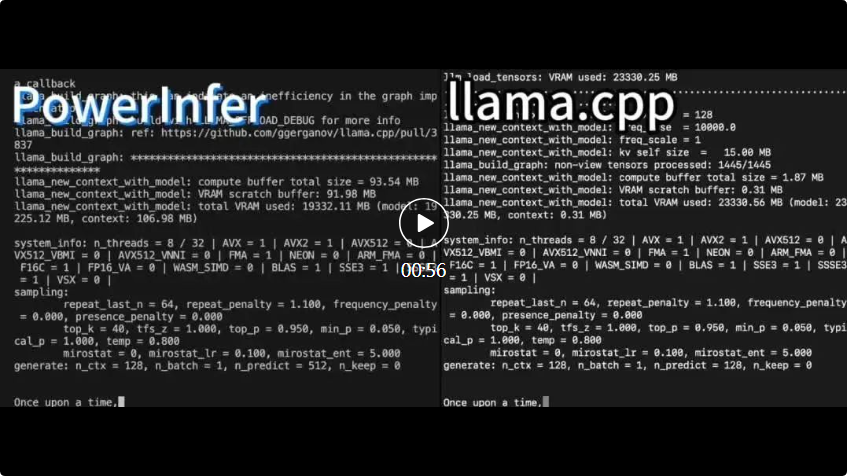
PowerInfer提高了在消费级硬件上运行AI的效率上海交大团队最新推出了超强CPU/GPULLM高速推理引擎PowerInfer。PowerInfer和llama.cpp都在相同的硬件上运行,并充分利用了RTX4090上的VRAM。这个推理引擎速度有多快?在单个NVIDIARTX4090GPU上运行LLM,PowerInfer的平均token生成速率为13.20tokens/s,峰值为29.08tokens/s,仅比顶级服务器A100GPU低18%,可适用于各种LLM。PowerInfer与
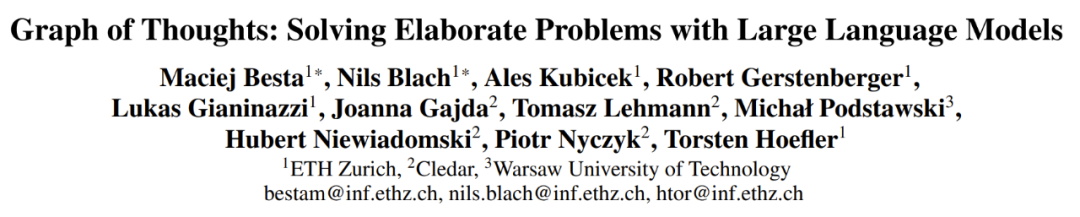
要让大型语言模型(LLM)充分发挥其能力,有效的prompt设计方案是必不可少的,为此甚至出现了promptengineering(提示工程)这一新兴领域。在各种prompt设计方案中,思维链(CoT)凭借其强大的推理能力吸引了许多研究者和用户的眼球,基于其改进的CoT-SC以及更进一步的思维树(ToT)也收获了大量关注。近日,苏黎世联邦理工学院、Cledar和华沙理工大学的一个研究团队提出了更进一步的想法:思维图(GoT)。让思维从链到树到图,为LLM构建推理过程的能力不断得到提升,研究者也通
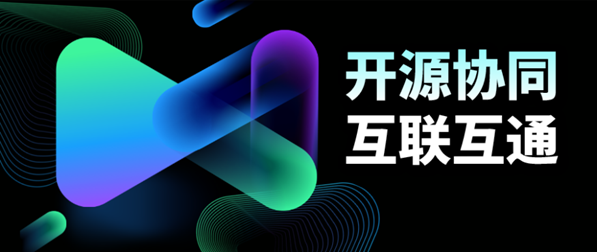
FATE2.0全面升级,推动隐私计算联邦学习规模化应用FATE开源平台宣布发布FATE2.0版本,作为全球领先的联邦学习工业级开源框架。此次更新实现了联邦异构系统之间的互联互通,持续增强了隐私计算平台的互联互通能力。这一进展进一步推动了联邦学习与隐私计算规模化应用的发展。FATE2.0以全面互通为设计理念,采用开源方式对应用层、调度、通信、异构计算(算法)四个层面进行改造,实现了系统与系统、系统与算法、算法与算法之间异构互通的能力。FATE2.0的设计兼容了北京金融科技产业联盟的《金融业隐私计算
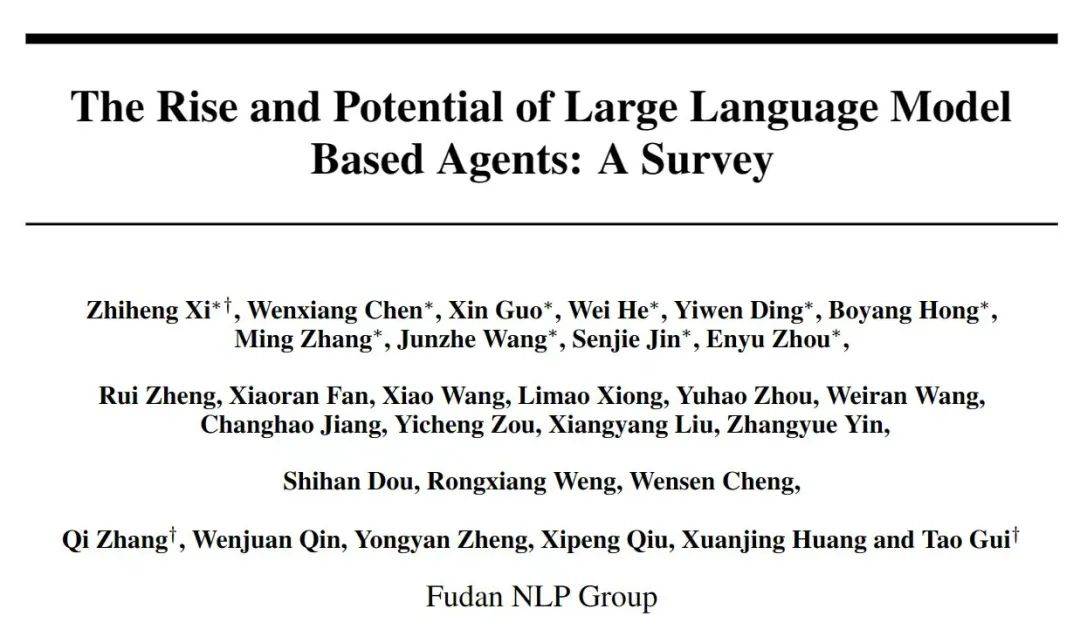
近期,复旦大学自然语言处理团队(FudanNLP)推出LLM-basedAgents综述论文,全文长达86页,共有600余篇参考文献!作者们从AIAgent的历史出发,全面梳理了基于大型语言模型的智能代理现状,包括:LLM-basedAgent的背景、构成、应用场景、以及备受关注的代理社会。同时,作者们探讨了Agent相关的前瞻开放问题,对于相关领域的未来发展趋势具有重要价值。论文链接:https://arxiv.org/pdf/2309.07864.pdfLLM-basedAgent论文列表:
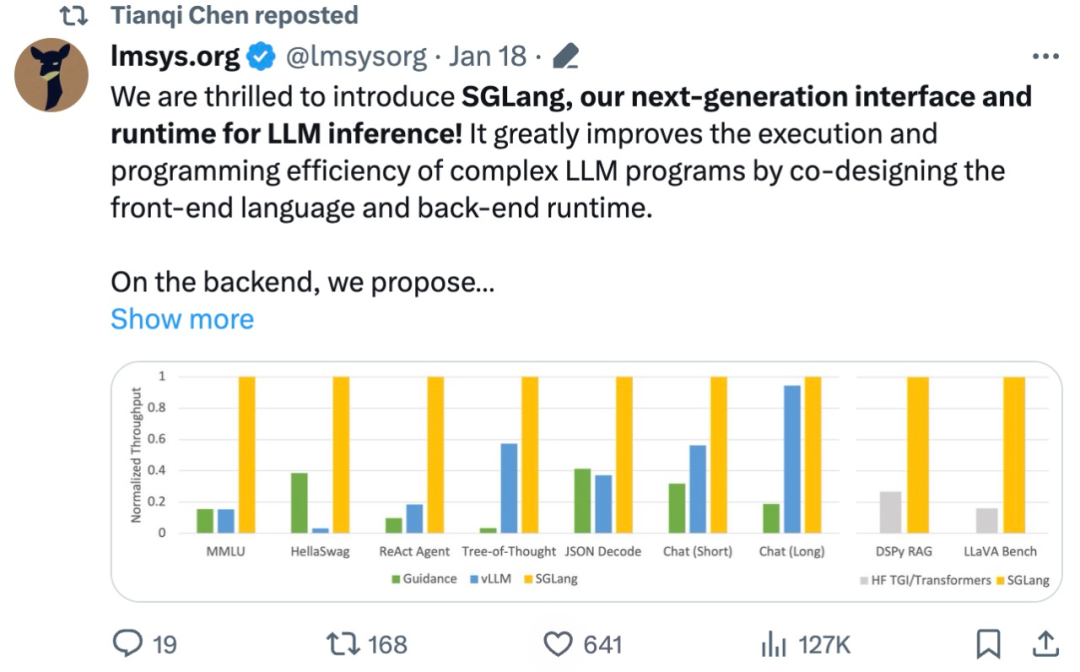
大型语言模型(LLM)被广泛应用于需要多个链式生成调用、高级提示技术、控制流以及与外部环境交互的复杂任务。尽管如此,目前用于编程和执行这些应用程序的高效系统却存在明显的不足之处。研究人员最近提出了一种新的结构化生成语言(StructuredGenerationLanguage),称为SGLang,旨在改进与LLM的交互性。通过整合后端运行时系统和前端语言的设计,SGLang使得LLM的性能更高、更易控制。这项研究也获得了机器学习领域的知名学者、CMU助理教授陈天奇的转发。总的来说,SGLang的
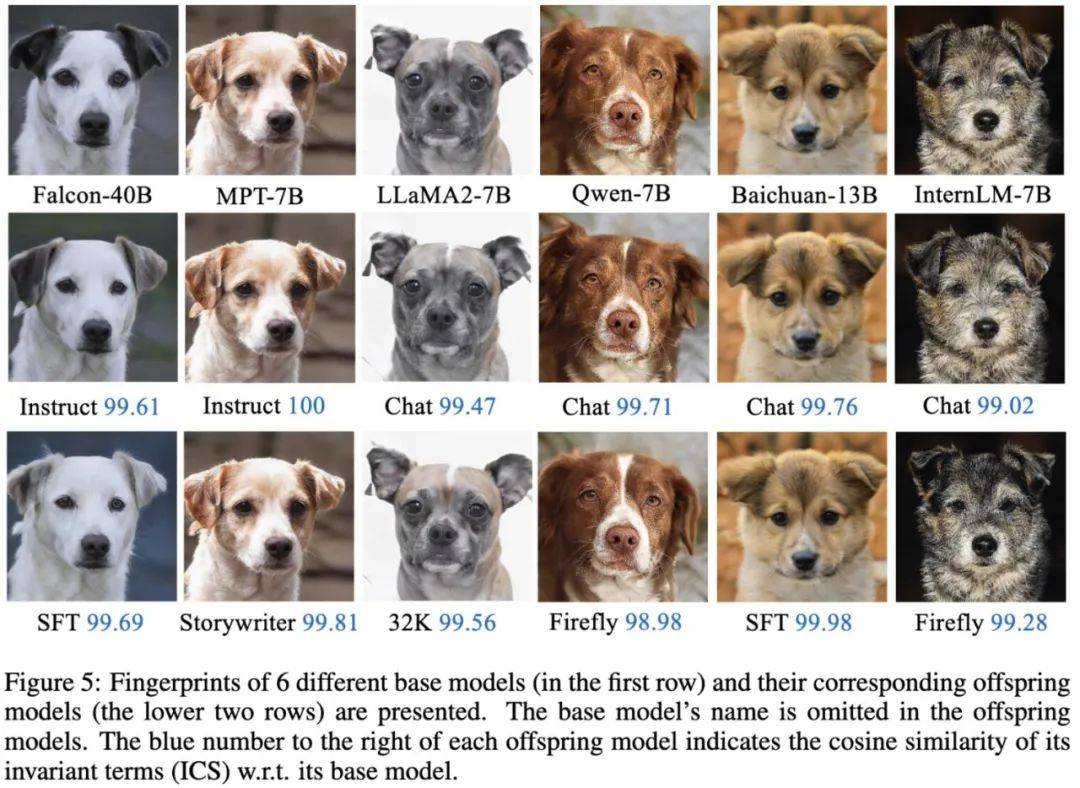
将不同的基模型象征为不同品种的狗,其中相同的「狗形指纹」表明它们源自同一个基模型。大模型的预训练需要耗费大量的计算资源和数据,因此预训练模型的参数成为各大机构重点保护的核心竞争力和资产。然而,与传统软件知识产权保护不同,对预训练模型参数盗用的判断存在以下两个新问题:1)预训练模型的参数,尤其是千亿级别模型的参数,通常不会开源。预训练模型的输出和参数会受到后续处理步骤(如SFT、RLHF、continuepretraining等)的影响,这使得判断一个模型是否基于另一个现有模型微调得来变得困难。无
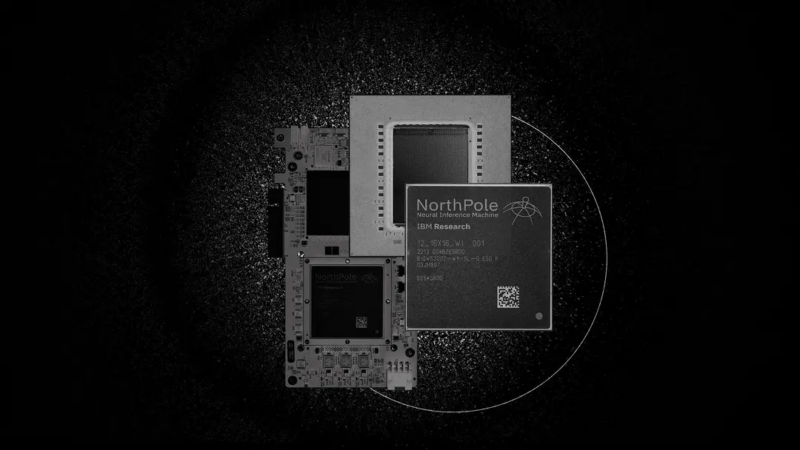
IBM再度发力。随着AI系统的飞速发展,其能源需求也在不断增加。训练新系统需要大量的数据集和处理器时间,因此能耗极高。在某些情况下,执行一些训练好的系统,智能手机就能轻松胜任。但是,执行的次数太多,能耗也会增加。幸运的是,有很多方法可以降低后者的能耗。IBM和英特尔已经试验过模仿实际神经元行为设计的处理器。IBM还测试了在相变存储器中执行神经网络计算,以避免重复访问RAM。现在,IBM又推出了另一种方法。该公司的新型NorthPole处理器综合了上述方法的一些理念,并将其与一种非常精简的计算运行
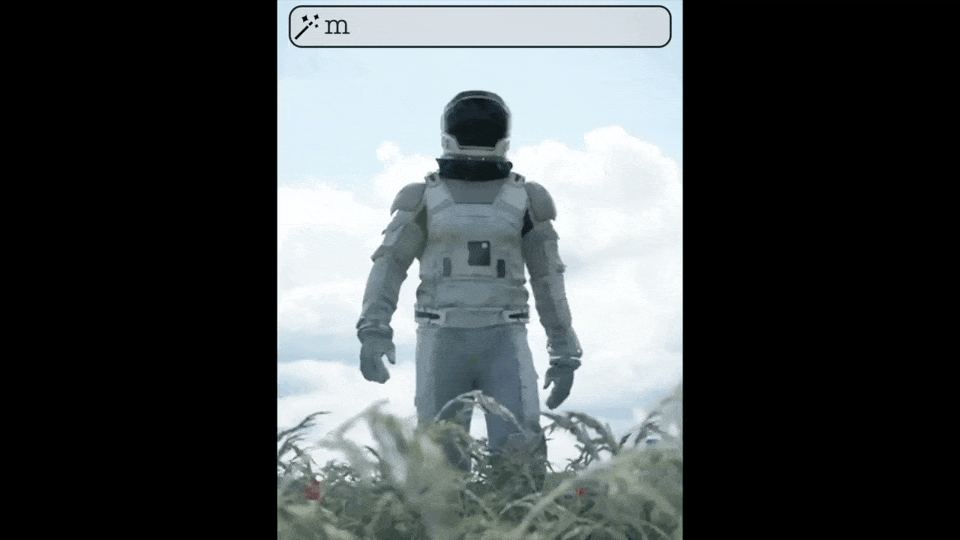
Meta的视频合成新框架给我们带来了一些惊喜就今天的人工智能发展水平来说,文生图、图生视频、图像/视频风格迁移都已经不算什么难事。生成式AI天赋异禀,能够毫不费力地创建或修改内容。尤其是图像编辑,在以十亿规模数据集为基础预训练的文本到图像扩散模型的推动下,经历了重大发展。这股浪潮催生了大量图像编辑和内容创建应用。基于图像的生成模型所取得的成就基础上,下一个挑战的领域必然是为其增加「时间维度」,从而实现轻松而富有创意的视频编辑。一种直接策略是使用图像模型逐帧处理视频,然而,生成式图像编辑本身就具有


Hot AI Tools

Undresser.AI Undress
AI-powered app for creating realistic nude photos

AI Clothes Remover
Online AI tool for removing clothes from photos.

Undress AI Tool
Undress images for free

Clothoff.io
AI clothes remover

AI Hentai Generator
Generate AI Hentai for free.

Hot Article

Hot Tools

MantisBT
Mantis is an easy-to-deploy web-based defect tracking tool designed to aid in product defect tracking. It requires PHP, MySQL and a web server. Check out our demo and hosting services.

mPDF
mPDF is a PHP library that can generate PDF files from UTF-8 encoded HTML. The original author, Ian Back, wrote mPDF to output PDF files "on the fly" from his website and handle different languages. It is slower than original scripts like HTML2FPDF and produces larger files when using Unicode fonts, but supports CSS styles etc. and has a lot of enhancements. Supports almost all languages, including RTL (Arabic and Hebrew) and CJK (Chinese, Japanese and Korean). Supports nested block-level elements (such as P, DIV),

Zend Studio 13.0.1
Powerful PHP integrated development environment
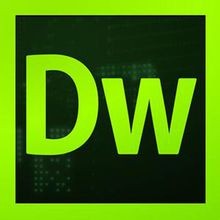
Dreamweaver CS6
Visual web development tools

Safe Exam Browser
Safe Exam Browser is a secure browser environment for taking online exams securely. This software turns any computer into a secure workstation. It controls access to any utility and prevents students from using unauthorized resources.
