


APISR, a two-dimensional dedicated super-resolution AI model: available online, selected by CVPR
Animation works such as "Dragon Ball", "Pokémon", "Neon Genesis Evangelion" and other animations that were aired in the last century are part of many people's childhood memories. They have brought us full of passion, friendship and dreams. visual journey. At some point, we will suddenly have the urge to revisit these childhood memories, but we may regretfully find that the recognition rate of these childhood memories is very low, and it is impossible to create a good visual experience on a widescreen TV, so that it hinders us. Share these childhood memories with children growing up in a digital world with HD resolution.
For this kind of vicious competition (and potential market), one way is to have animation companies produce remakes. This task will be costly in both human and financial terms, but may be worth more than ignoring the problem and losing market share.
The performance of multi-modal artificial intelligence is becoming increasingly powerful, and using AI-based super-resolution technology to improve animation resolution has become a direction worth exploring. This technology can reconstruct high-resolution images from a small number of low-resolution images, making animation images clearer and more detailed. This method uses depth by training a large number of sample data. Recently, a joint team from the University of Michigan, Yale University and Zhejiang University created a set of tools for animation super-resolution tasks by analyzing the animation production process. A very practical new method. This includes datasets, models, and some improvements. This research has been accepted into the CVPR 2024 conference. The team also open sourced the relevant code and launched a trial model on Huggingface.
- Paper title: APISR: Anime Production Inspired Real-World Anime Super-Resolution
- Paper address :https://arxiv.org/pdf/2403.01598.pdf
- Code address: https://github.com/Kiteretsu77/APISR
- Trial model: https://huggingface.co/spaces/HikariDawn/APISR
- The picture below is the result of this site’s attempt using the screenshot of the first episode of "Dragon Ball". The effect is visible to the naked eye. Visible good.
In addition, some people have tried to use this technology to improve video resolution, and the results are great:
In order to understand the innovation of this new method, let’s first take a look at how animation is generally produced.
First, a human sketches on paper, which is then colored and enhanced through computer generated image (CGI) processing. These processed sketches are then connected to create a video.
However, because the drawing process is very labor-intensive and the human eye is not sensitive to motion, when compositing videos, the industry standard practice is to reuse a single image for multiple consecutive frames.
By analyzing this process, the joint team couldn’t help but wonder whether it was necessary to use video models and video datasets to train animation super-resolution models: it is entirely possible to perform super-resolution on images and then concatenate these images. Get up!
So they decided to use image-based methods and data sets to create a unified super-resolution and restoration framework suitable for images and videos.
New proposed methodImage super-resolution (API SR) data set for animation production
The team The API SR data set is proposed, and its collection and organization method is briefly introduced here. This method takes advantage of the characteristics of animation videos (see Figure 2) and can select the least compressed and most informative frames from the video.
I-frame based image collection: Video compression involves a trade-off between video quality and data size. There are many video compression standards now, each with its own complex engineering system, but they all have a similar backbone design.
These characteristics cause the compression quality of each frame to be different. The video compression process designates a number of key frames (i.e., I-frames) as individual compression units. In practice, the I-frame is the first frame when the scene changes. These I-frames can occupy a large amount of data. Non-I frames (i.e. P frames and B frames) have higher compression rates, and they need to use the I frame as a reference during the compression process to introduce changes over time. As shown in Figure 3a, in the animation videos collected by the team, the data size of I frames is generally higher than that of non-I frames, and the quality of I frames is indeed higher. Therefore, the team used the video processing tool ffmpeg to extract all I-frames from the video source and use them as an initial data pool.
画像の複雑さに基づく選択: チームは、アニメーションにより適した指標である画像複雑性評価 (ICA) に基づいて初期 I フレーム プールをスクリーニングしました (図 4 を参照)。
API データセット: チームは 562 個の高品質のアニメ ビデオを手動で収集しました。上記の 2 つの手順に基づいて、各ビデオから最高スコアの 10 フレームが収集されました。その後、不適切な画像を除去するためにいくつかのフィルタリングが実行され、最終的に 3740 枚の高品質画像を含むデータセットが取得されました。図 5 にいくつかの画像の例を示します。さらに、図 3b からは、画像の複雑さに関する API データ セットの利点もわかります。
元の 720P 解像度に戻る: アニメーション制作プロセスを調査すると、ほとんどのアニメーション制作で 720P 形式が使用されていることがわかります (つまり、画像の高さは 720 ピクセルです) )。しかし、現実のシナリオでは、マルチメディア形式を標準化するために、アニメが誤って 1080P または他の形式にアップスケールされることがよくあります。チームは実験的に、すべてのアニメ画像のサイズをネイティブ 720P に変更すると、クリエイターが思い描いた機能密度が提供され、より緻密なアニメ手描きの線と CGI 情報が得られることを発見しました。
#アニメーション用の実用的な劣化モデル
現実世界の超解像タスクでは、劣化モデルの設計が非常に重要です。研究チームは、高次の劣化モデルと最近の画像ベースのビデオ圧縮回復モデルに基づいて、歪んだ手描きの線やさまざまな圧縮アーティファクトを復元し、劣化モデルの表現を強化できる 2 つの改善を提案しました。図 6a は、この劣化モデルを示しています。アニメーション用に手描きの線を強化する
チームの選択は、鮮明になった手描きの線情報を直接抽出し、それをグラウンド トゥルース (GT/ground) と比較することです。 -truth ) を融合し、擬似 GT を形成します。この特別にターゲットを絞った強化された擬似 GT を超解像度トレーニング プロセスに導入することで、追加のニューラル ネットワーク モジュールや別個の後処理ネットワークを導入することなく、ネットワークは鮮明な手描きの線を生成できます。 手描きの線をより適切に抽出するために、チームは、GT のシャープなエッジ マップを抽出できる、ピクセル単位のガウス カーネルに基づくスケッチ抽出アルゴリズムである XDoG を使用しました。 ただし、XDoG エッジ マップには、異常値のピクセルや破線表現を含む過度のノイズが発生します。この問題を解決するために、チームは、カスタム設計のパッシブ拡張法と組み合わせた外れ値フィルタリング手法を提案しました。このようにして、手描きの線のより一貫性のある、乱れのない表現が得られます。 チームは、前処理された GT を過度にシャープ化すると、手描きの線のエッジが他の無関係なシャドウ エッジの詳細よりも目立つようになり、外れ値フィルターがそれらを区別しやすくなる可能性があることを実験的に発見しました。これを行うために、チームはまず GT 上で 3 ラウンドのデシャープニング マスキング操作を実行することを提案しました。図 8 は、このプロセスを簡単に示しています。アニメーション向けのバランスのとれたデュアル知覚損失
主にトレーニング内データが原因で、不要なカラー アーティファクトの問題もあります。ジェネレーターと知覚損失の間のドメインの不一致。この問題を解決し、以前の方法の欠点を補うために、チームのアプローチは、Danbooru データ セットのアニメーション ターゲット分類タスクでトレーニングされた事前トレーニング済み ResNet を使用することでした。 Danbooru データセットは、大規模で豊富な注釈を含むアニメ イラスト データベースです。この事前トレーニング済みネットワークは VGG ではなく ResNet50 であるため、チームは同様の中間層の比較も提案しました。
ただし、ResNet ベースの損失のみを使用する場合は、視覚的な結果が不十分になる可能性があります。これは、Danbooru データセットに固有のバイアスが原因で発生します。このデータセット内のほとんどの画像は人間の顔か、比較的単純なものです。 .イラスト。したがって、チームは検討を加え、トレーニング中の ResNet ベースの知覚損失をガイドする補助として現実世界の特徴を使用することを決定しました。この方法により、視覚的に心地よい画像が得られると同時に、不要な色の問題も解決されます。
実験
実装の詳細
実験では、チームは新しく提案された API データ セットを画像ネットワークとして使用しました。トレーニングデータセット。画像ネットワークに関しては、GRL の小型バージョンが最も近い畳み込みアップサンプリング モジュールとともに使用されます。
詳細とパラメータについては、元の論文を参照してください。
現在の最良の方法との比較
チームは、新しく提案された APISR を、Real-ESRGAN、BSRGAN、RealBasicVSR、AnimeSR などの他の高度な方法と定量的および定性的に比較しました。そしてVQD-SR。
定量的比較
表 1 に示すように、新しいモデルのネットワーク サイズは最小であり、パラメーターは 103 万個のみですが、すべての指標でのパフォーマンスは他のすべてを上回っています。 。 方法。
チームは、予測指向の圧縮モデルの役割を特に強調しました。
さらに、新しい方法では、AnimeSR と VQDSR のトレーニング サンプルの複雑さがそれぞれ 13.3% と 25% の場合にのみこのような結果が得られることを指摘しておく必要があります。これは主に、データセットの並べ替えプロセスに画像の複雑さ評価が導入されたことによるもので、情報が豊富な画像を選択することでアニメーション画像表現の学習効果を向上させることができます。さらに、新たに設計された明示的な劣化モデルにより、劣化モデル側のトレーニングは不要です。
定性的比較
図 10 に示すように、APISR によって得られる視覚的な品質は、他の方法よりもはるかに優れています。
チームはまた、新しいデータセット、劣化モデル、損失設計の有効性を検証するためにアブレーション研究も実施しました。詳細については元の論文を参照してください。
The above is the detailed content of APISR, a two-dimensional dedicated super-resolution AI model: available online, selected by CVPR. For more information, please follow other related articles on the PHP Chinese website!
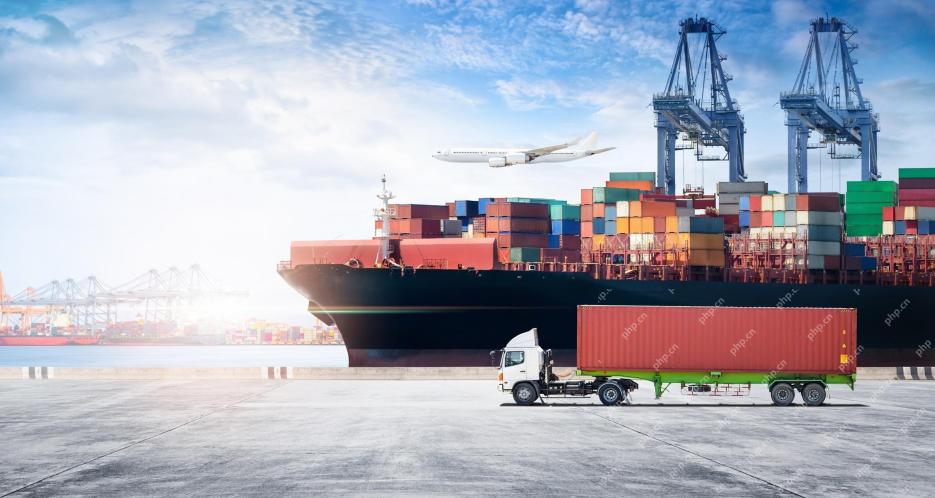
The term "AI-ready workforce" is frequently used, but what does it truly mean in the supply chain industry? According to Abe Eshkenazi, CEO of the Association for Supply Chain Management (ASCM), it signifies professionals capable of critic
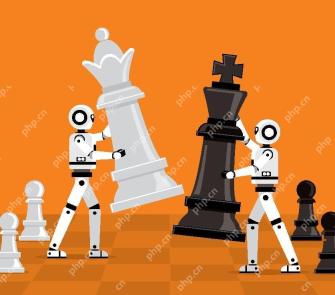
The decentralized AI revolution is quietly gaining momentum. This Friday in Austin, Texas, the Bittensor Endgame Summit marks a pivotal moment, transitioning decentralized AI (DeAI) from theory to practical application. Unlike the glitzy commercial
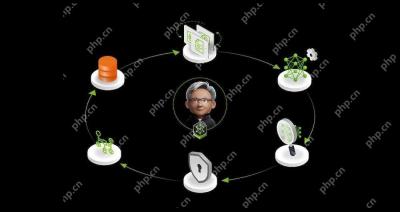
Enterprise AI faces data integration challenges The application of enterprise AI faces a major challenge: building systems that can maintain accuracy and practicality by continuously learning business data. NeMo microservices solve this problem by creating what Nvidia describes as "data flywheel", allowing AI systems to remain relevant through continuous exposure to enterprise information and user interaction. This newly launched toolkit contains five key microservices: NeMo Customizer handles fine-tuning of large language models with higher training throughput. NeMo Evaluator provides simplified evaluation of AI models for custom benchmarks. NeMo Guardrails implements security controls to maintain compliance and appropriateness
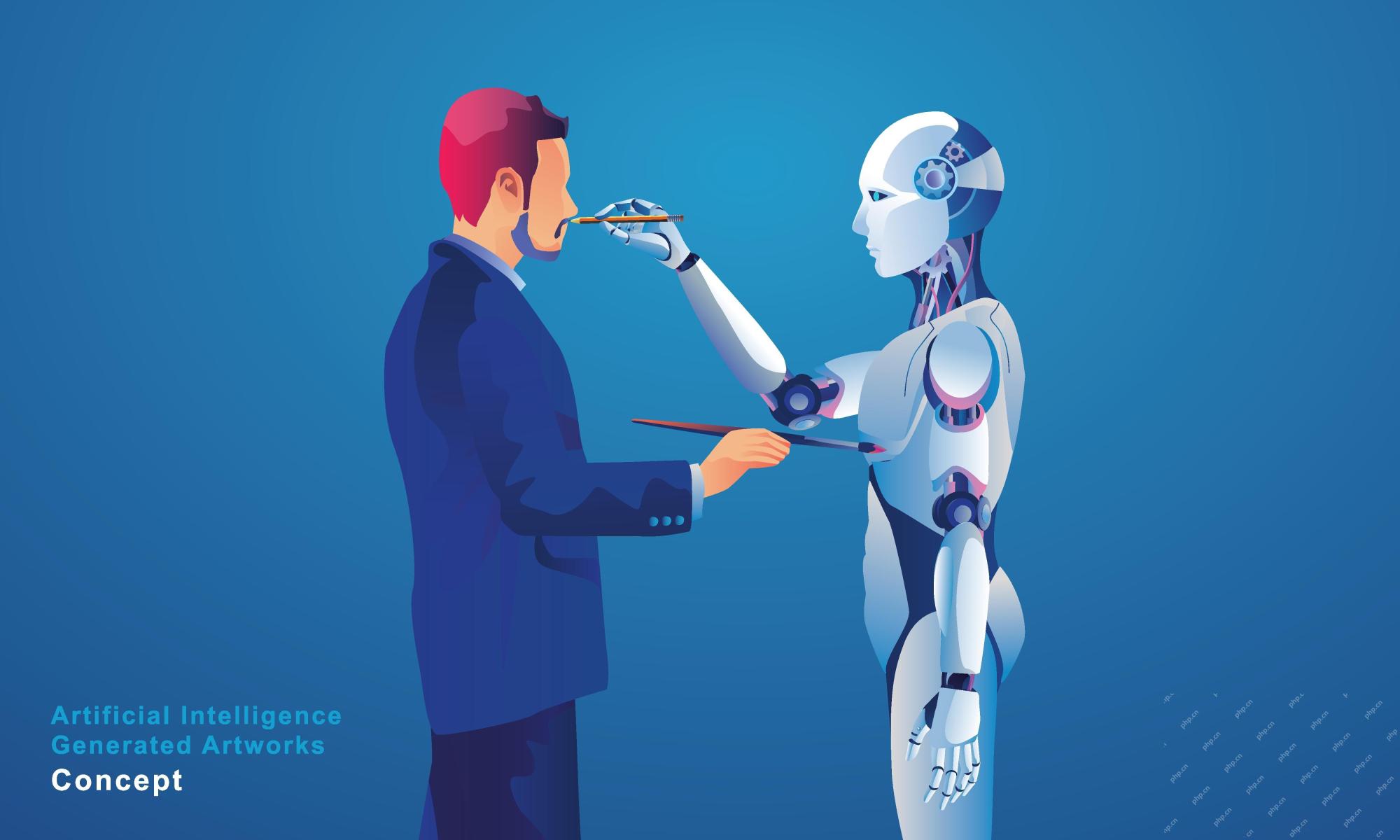
AI: The Future of Art and Design Artificial intelligence (AI) is changing the field of art and design in unprecedented ways, and its impact is no longer limited to amateurs, but more profoundly affecting professionals. Artwork and design schemes generated by AI are rapidly replacing traditional material images and designers in many transactional design activities such as advertising, social media image generation and web design. However, professional artists and designers also find the practical value of AI. They use AI as an auxiliary tool to explore new aesthetic possibilities, blend different styles, and create novel visual effects. AI helps artists and designers automate repetitive tasks, propose different design elements and provide creative input. AI supports style transfer, which is to apply a style of image
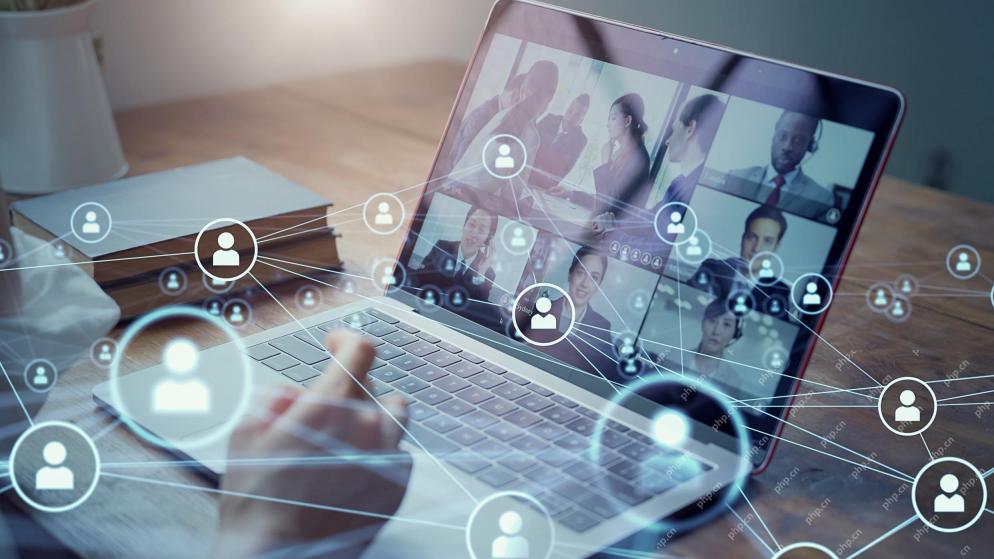
Zoom, initially known for its video conferencing platform, is leading a workplace revolution with its innovative use of agentic AI. A recent conversation with Zoom's CTO, XD Huang, revealed the company's ambitious vision. Defining Agentic AI Huang d
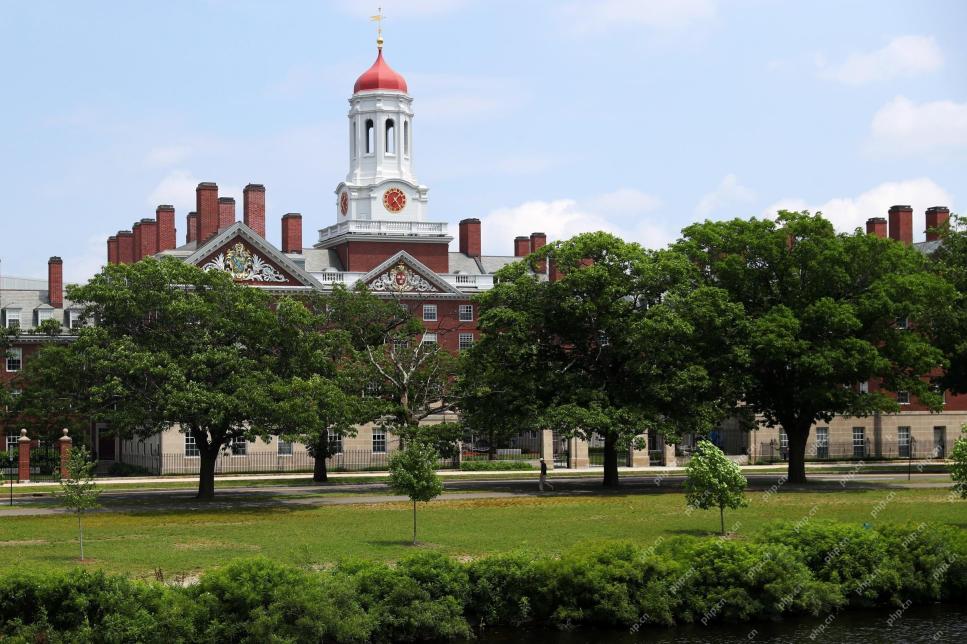
Will AI revolutionize education? This question is prompting serious reflection among educators and stakeholders. The integration of AI into education presents both opportunities and challenges. As Matthew Lynch of The Tech Edvocate notes, universit
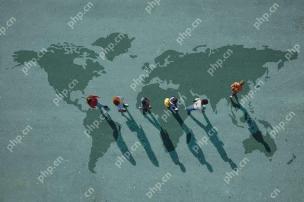
The development of scientific research and technology in the United States may face challenges, perhaps due to budget cuts. According to Nature, the number of American scientists applying for overseas jobs increased by 32% from January to March 2025 compared with the same period in 2024. A previous poll showed that 75% of the researchers surveyed were considering searching for jobs in Europe and Canada. Hundreds of NIH and NSF grants have been terminated in the past few months, with NIH’s new grants down by about $2.3 billion this year, a drop of nearly one-third. The leaked budget proposal shows that the Trump administration is considering sharply cutting budgets for scientific institutions, with a possible reduction of up to 50%. The turmoil in the field of basic research has also affected one of the major advantages of the United States: attracting overseas talents. 35
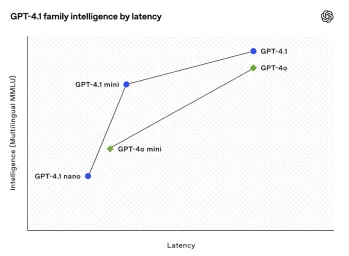
OpenAI unveils the powerful GPT-4.1 series: a family of three advanced language models designed for real-world applications. This significant leap forward offers faster response times, enhanced comprehension, and drastically reduced costs compared t


Hot AI Tools

Undresser.AI Undress
AI-powered app for creating realistic nude photos

AI Clothes Remover
Online AI tool for removing clothes from photos.

Undress AI Tool
Undress images for free

Clothoff.io
AI clothes remover

Video Face Swap
Swap faces in any video effortlessly with our completely free AI face swap tool!

Hot Article

Hot Tools
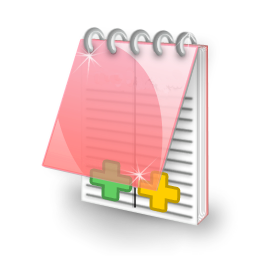
EditPlus Chinese cracked version
Small size, syntax highlighting, does not support code prompt function

MantisBT
Mantis is an easy-to-deploy web-based defect tracking tool designed to aid in product defect tracking. It requires PHP, MySQL and a web server. Check out our demo and hosting services.

SAP NetWeaver Server Adapter for Eclipse
Integrate Eclipse with SAP NetWeaver application server.

mPDF
mPDF is a PHP library that can generate PDF files from UTF-8 encoded HTML. The original author, Ian Back, wrote mPDF to output PDF files "on the fly" from his website and handle different languages. It is slower than original scripts like HTML2FPDF and produces larger files when using Unicode fonts, but supports CSS styles etc. and has a lot of enhancements. Supports almost all languages, including RTL (Arabic and Hebrew) and CJK (Chinese, Japanese and Korean). Supports nested block-level elements (such as P, DIV),

MinGW - Minimalist GNU for Windows
This project is in the process of being migrated to osdn.net/projects/mingw, you can continue to follow us there. MinGW: A native Windows port of the GNU Compiler Collection (GCC), freely distributable import libraries and header files for building native Windows applications; includes extensions to the MSVC runtime to support C99 functionality. All MinGW software can run on 64-bit Windows platforms.
