


ICLR 2024 | Model critical layers for federated learning backdoor attacks
Federated learning uses multiple parties to train models while data privacy is protected. However, because the server cannot monitor the training process performed locally by participants, participants can tamper with the local training model, thus posing security risks to the overall federated learning model, such as backdoor attacks.
This article focuses on how to launch a backdoor attack on federated learning under a defensively protected training framework. This paper finds that the implantation of backdoor attacks is more closely related to some neural network layers, and calls these layers the key layers for backdoor attacks. In federated learning, clients participating in training are distributed on different devices. They each train their own models, and then upload the updated model parameters to the server for aggregation. Since the client participating in the training is not trustworthy and there is a certain risk, the server
is based on the discovery of the key layer of the backdoor. This article proposes to bypass the defense algorithm detection by attacking the key layer of the backdoor, so that a small number of participants can be controlled to perform efficient backdoor attack.
Paper title: Backdoor Federated Learning By Poisoning Backdoor-Critical Layers
Paper link: https://openreview.net/pdf?id=AJBGSVSTT2
Code link: https://github.com/zhmzm/Poisoning_Backdoor-critical_Layers_Attack
Method
This article A layer replacement method is proposed to identify key layers of backdoors. The specific method is as follows:
The first step is to train the model on a clean data set until convergence, and save the model parameters as benign model
. Then copy the benign model and train it on the data set containing the backdoor. After convergence, save the model parameters and record them as malicious model
.
The second step is to replace a layer of parameters in the benign model into the malicious model containing the backdoor, and calculate the backdoor attack success rate
of the resulting model. The difference between the obtained backdoor attack success rate and the malicious model's backdoor attack success rate BSR is ΔBSR, which can be used to obtain the impact of this layer on backdoor attacks. Using the same method for each layer in the neural network, you can get a list of the impact of all layers on backdoor attacks.
The third step is to sort all layers according to their impact on backdoor attacks. Take the layer with the greatest impact from the list and add it to the backdoor attack key layer set
, and implant the backdoor attack key layer (layers in the set
) parameters in the malicious model into the benign model. Calculate the backdoor attack success rate
of the obtained model. If the backdoor attack success rate is greater than the set threshold τ multiplied by the malicious model backdoor attack success rate
, the algorithm will be stopped. If it is not satisfied, continue to add the largest layer among the remaining layers in the list to the key layer for backdoor attack
until the conditions are met.
After obtaining the collection of key layers of backdoor attacks, this article proposes a method to bypass the detection of defense methods by attacking the key layers of backdoors. In addition, this paper introduces simulation aggregation and benign model centers to further reduce the distance from other benign models.
Experimental results
This article verifies the effectiveness of key layer attacks based on backdoors on multiple defense methods on the CIFAR-10 and MNIST data sets. The experiment will use the backdoor attack success rate BSR and the malicious model acceptance rate MAR (benign model acceptance rate BAR) as indicators to measure the effectiveness of the attack.
First of all, layer-based attack LP Attack can allow malicious clients to obtain a high selection rate. As shown in the table below, LP Attack achieved a reception rate of 90% on the CIFAR-10 dataset, which is much higher than the 34% of benign users.
Then, LP Attack can achieve a high backdoor attack success rate, even in a setting with only 10% malicious clients. As shown in the table below, LP Attack can achieve a high backdoor attack success rate BSR under the protection of different data sets and different defense methods.
In the ablation experiment, this article poisoned the backdoor key layer and the non-backdoor key layer respectively and measured the backdoor attack success rate of the two experiments. As shown in the figure below, when attacking the same number of layers, the success rate of poisoning non-backdoor key layers is much lower than that of poisoning backdoor key layers. This shows that the algorithm in this article can select effective backdoor attack key layers.
In addition, we conduct ablation experiments on the model aggregation module Model Averaging and the adaptive control module Adaptive Control. As shown in the table below, both modules improve the selection rate and backdoor attack success rate, proving the effectiveness of these two modules.
Summary
This article found that backdoor attacks are closely related to some layers, and proposed an algorithm to search for key layers of backdoor attacks. This paper proposes a layer-wise attack on the protection algorithm in federated learning by using backdoors to attack key layers. The proposed attack reveals the vulnerabilities of the current three types of defense methods, indicating that more sophisticated defense algorithms will be needed to protect federated learning security in the future.
Introduction to the author
Zhuang Haomin, graduated from South China University of Technology with a bachelor's degree, worked as a research assistant in the IntelliSys Laboratory of Louisiana State University, and is currently studying for a doctoral degree at the University of Notre Dame . The main research directions are backdoor attacks and adversarial sample attacks.
The above is the detailed content of ICLR 2024 | Model critical layers for federated learning backdoor attacks. For more information, please follow other related articles on the PHP Chinese website!
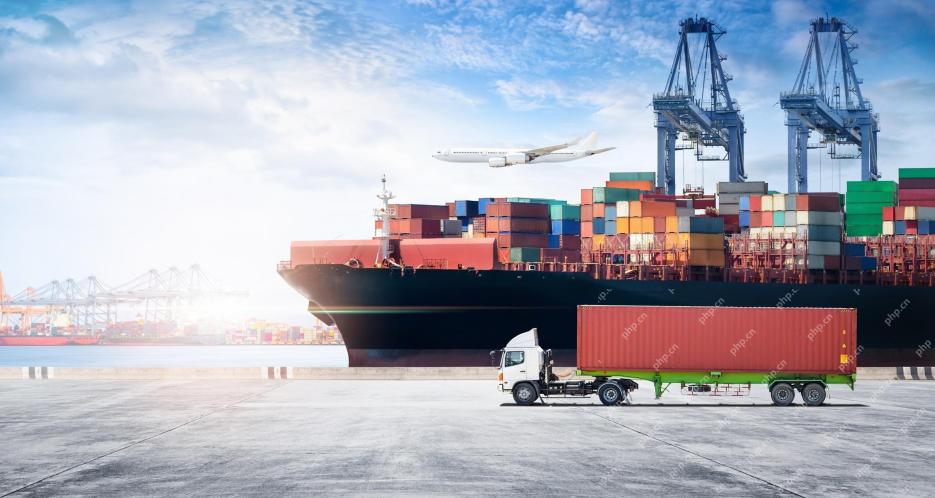
The term "AI-ready workforce" is frequently used, but what does it truly mean in the supply chain industry? According to Abe Eshkenazi, CEO of the Association for Supply Chain Management (ASCM), it signifies professionals capable of critic
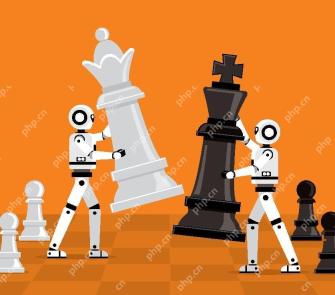
The decentralized AI revolution is quietly gaining momentum. This Friday in Austin, Texas, the Bittensor Endgame Summit marks a pivotal moment, transitioning decentralized AI (DeAI) from theory to practical application. Unlike the glitzy commercial
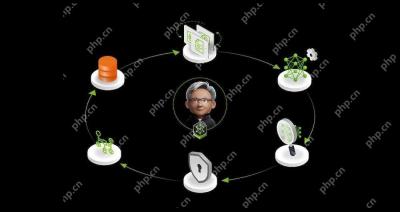
Enterprise AI faces data integration challenges The application of enterprise AI faces a major challenge: building systems that can maintain accuracy and practicality by continuously learning business data. NeMo microservices solve this problem by creating what Nvidia describes as "data flywheel", allowing AI systems to remain relevant through continuous exposure to enterprise information and user interaction. This newly launched toolkit contains five key microservices: NeMo Customizer handles fine-tuning of large language models with higher training throughput. NeMo Evaluator provides simplified evaluation of AI models for custom benchmarks. NeMo Guardrails implements security controls to maintain compliance and appropriateness
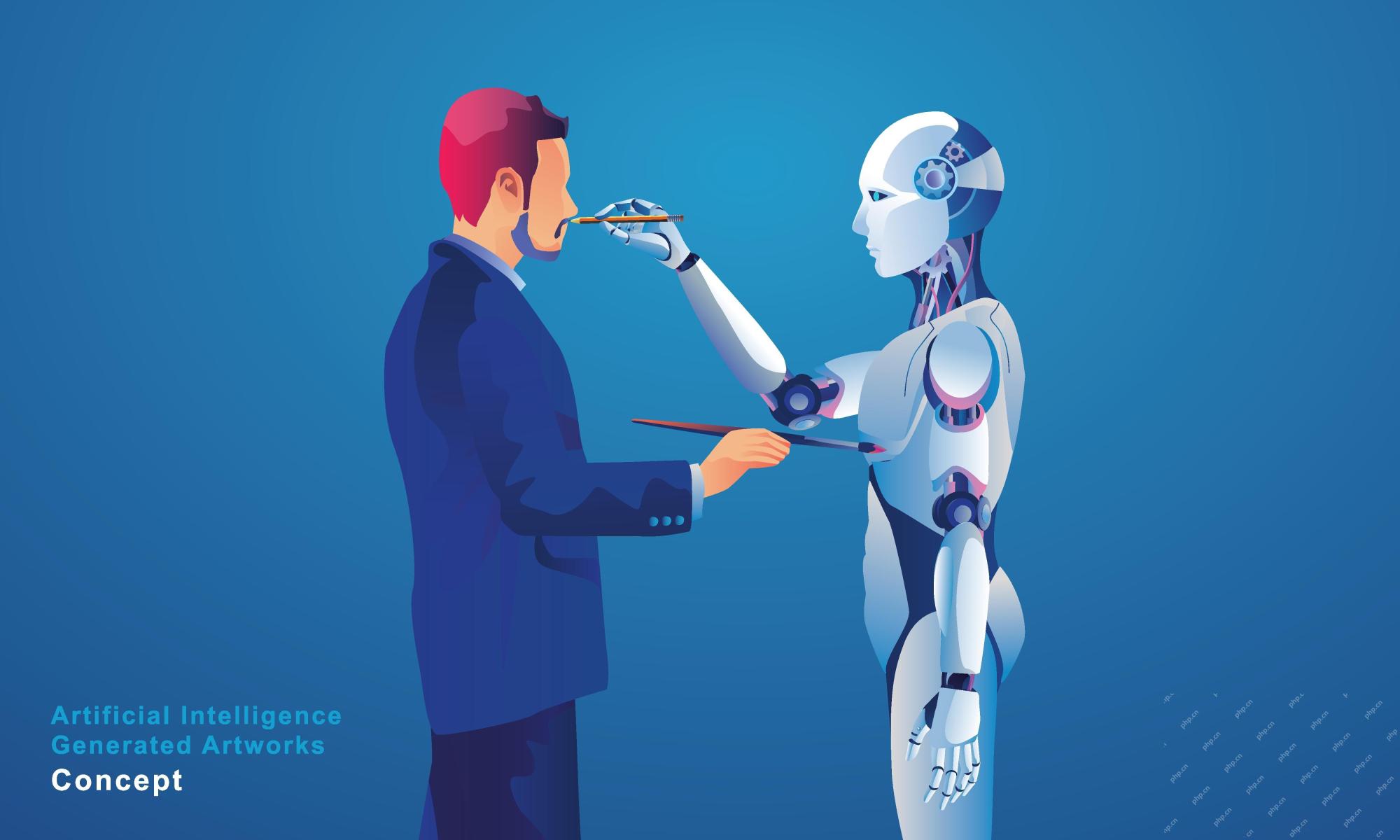
AI: The Future of Art and Design Artificial intelligence (AI) is changing the field of art and design in unprecedented ways, and its impact is no longer limited to amateurs, but more profoundly affecting professionals. Artwork and design schemes generated by AI are rapidly replacing traditional material images and designers in many transactional design activities such as advertising, social media image generation and web design. However, professional artists and designers also find the practical value of AI. They use AI as an auxiliary tool to explore new aesthetic possibilities, blend different styles, and create novel visual effects. AI helps artists and designers automate repetitive tasks, propose different design elements and provide creative input. AI supports style transfer, which is to apply a style of image
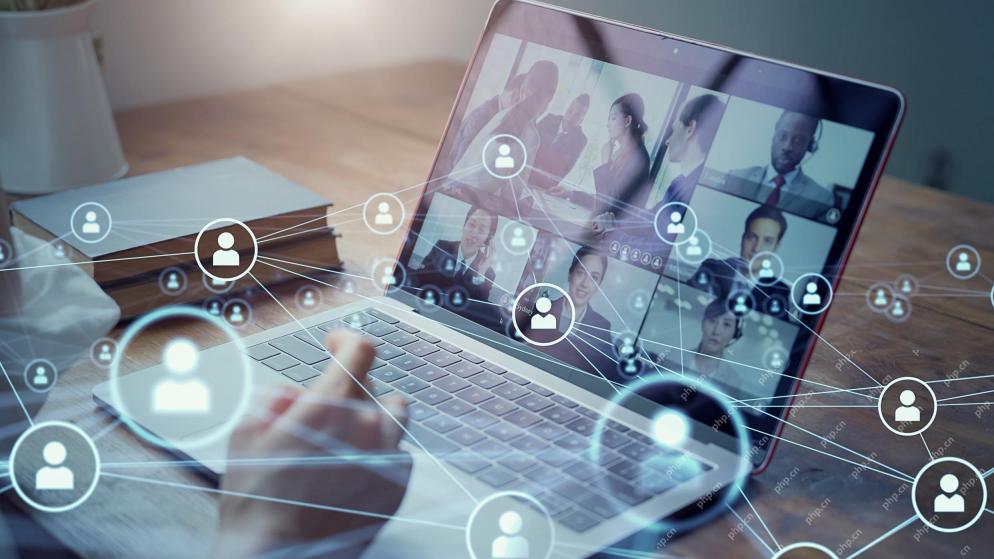
Zoom, initially known for its video conferencing platform, is leading a workplace revolution with its innovative use of agentic AI. A recent conversation with Zoom's CTO, XD Huang, revealed the company's ambitious vision. Defining Agentic AI Huang d
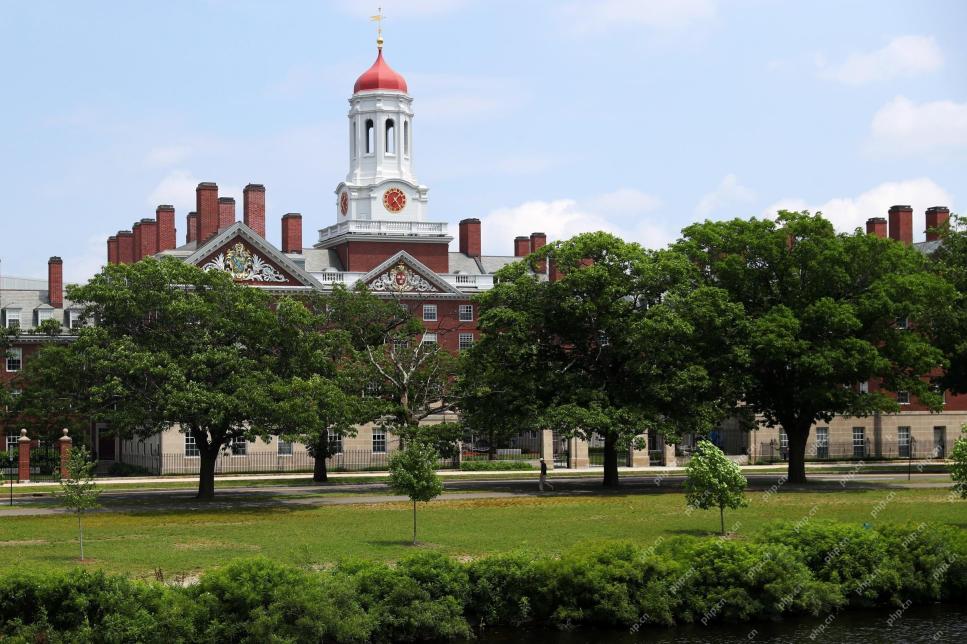
Will AI revolutionize education? This question is prompting serious reflection among educators and stakeholders. The integration of AI into education presents both opportunities and challenges. As Matthew Lynch of The Tech Edvocate notes, universit
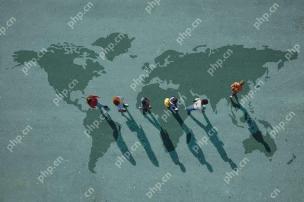
The development of scientific research and technology in the United States may face challenges, perhaps due to budget cuts. According to Nature, the number of American scientists applying for overseas jobs increased by 32% from January to March 2025 compared with the same period in 2024. A previous poll showed that 75% of the researchers surveyed were considering searching for jobs in Europe and Canada. Hundreds of NIH and NSF grants have been terminated in the past few months, with NIH’s new grants down by about $2.3 billion this year, a drop of nearly one-third. The leaked budget proposal shows that the Trump administration is considering sharply cutting budgets for scientific institutions, with a possible reduction of up to 50%. The turmoil in the field of basic research has also affected one of the major advantages of the United States: attracting overseas talents. 35
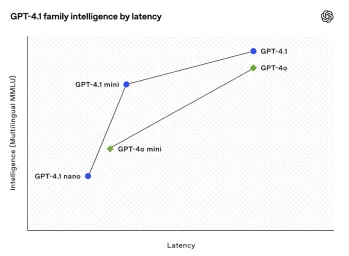
OpenAI unveils the powerful GPT-4.1 series: a family of three advanced language models designed for real-world applications. This significant leap forward offers faster response times, enhanced comprehension, and drastically reduced costs compared t


Hot AI Tools

Undresser.AI Undress
AI-powered app for creating realistic nude photos

AI Clothes Remover
Online AI tool for removing clothes from photos.

Undress AI Tool
Undress images for free

Clothoff.io
AI clothes remover

Video Face Swap
Swap faces in any video effortlessly with our completely free AI face swap tool!

Hot Article

Hot Tools
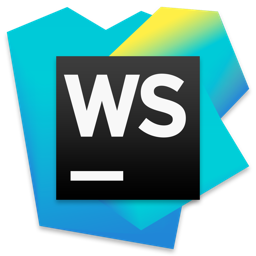
WebStorm Mac version
Useful JavaScript development tools

mPDF
mPDF is a PHP library that can generate PDF files from UTF-8 encoded HTML. The original author, Ian Back, wrote mPDF to output PDF files "on the fly" from his website and handle different languages. It is slower than original scripts like HTML2FPDF and produces larger files when using Unicode fonts, but supports CSS styles etc. and has a lot of enhancements. Supports almost all languages, including RTL (Arabic and Hebrew) and CJK (Chinese, Japanese and Korean). Supports nested block-level elements (such as P, DIV),
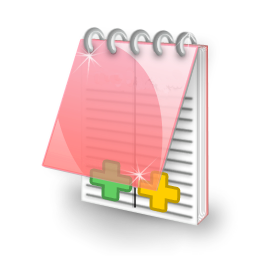
EditPlus Chinese cracked version
Small size, syntax highlighting, does not support code prompt function

DVWA
Damn Vulnerable Web App (DVWA) is a PHP/MySQL web application that is very vulnerable. Its main goals are to be an aid for security professionals to test their skills and tools in a legal environment, to help web developers better understand the process of securing web applications, and to help teachers/students teach/learn in a classroom environment Web application security. The goal of DVWA is to practice some of the most common web vulnerabilities through a simple and straightforward interface, with varying degrees of difficulty. Please note that this software

SublimeText3 English version
Recommended: Win version, supports code prompts!
